When Generative AI Gets It Wrong—Who Takes the Blame?
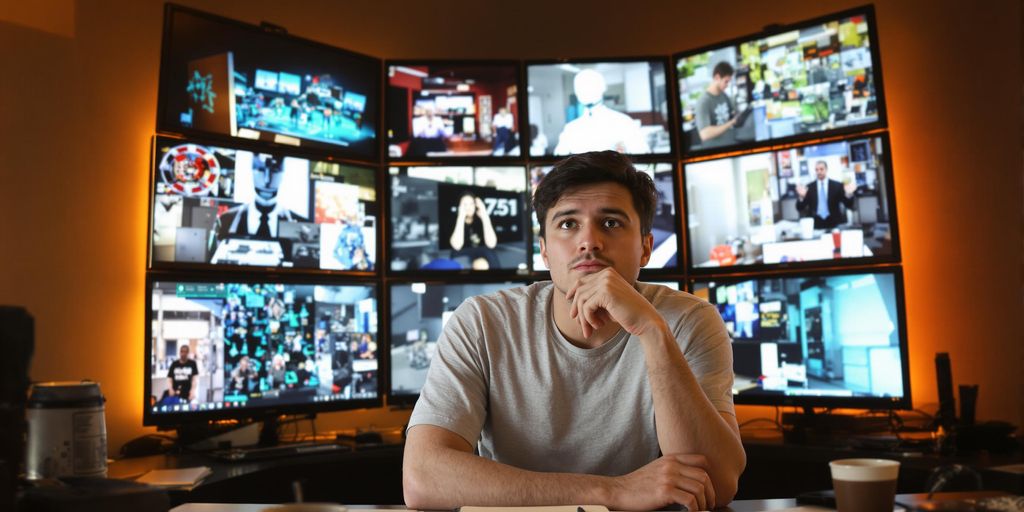
Generative AI is everywhere now, from chatbots to content creators. But what happens when it messes up? Whether it’s giving bad advice or producing biased outputs, the question of who takes the blame is a tricky one. Is it the programmers, the companies, or maybe even the AI itself? This article dives into the messy world of accountability when it comes to generative AI accuracy.
Key Takeaways
- Generative AI accuracy depends heavily on the quality of data and algorithms.
- Legal responsibility for AI errors is still a gray area with no clear answers.
- Ethical concerns like bias and transparency make accountability even harder.
- Regulations are struggling to keep up with the rapid advancement of AI technology.
- Human oversight remains crucial to minimizing AI mistakes and improving trust.
Understanding Generative AI Accuracy and Its Implications
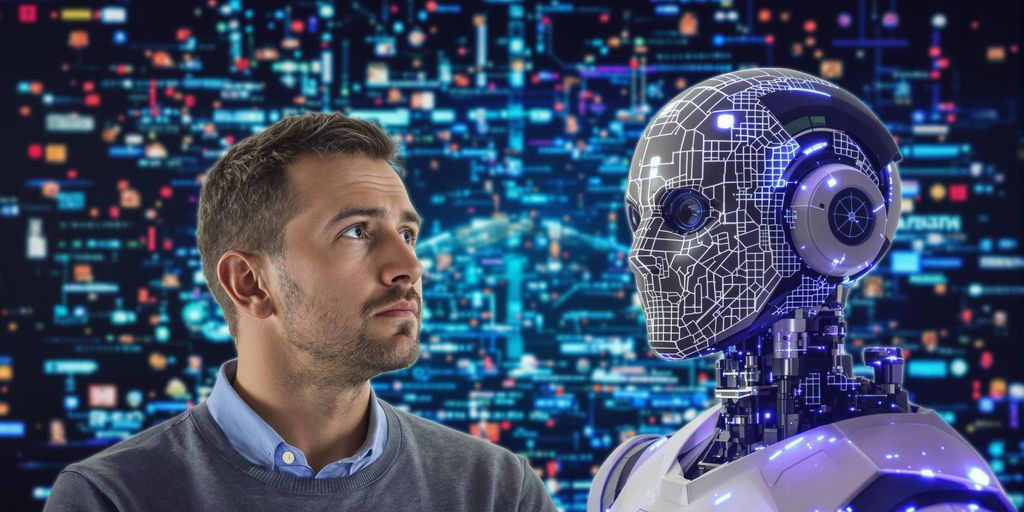
The Role of Data in AI Accuracy
Data is the backbone of any AI system. Generative AI tools rely on massive datasets to learn patterns, structures, and relationships. The quality of these datasets directly impacts the accuracy of the AI's outputs. If the data is biased, incomplete, or outdated, the AI will inevitably reflect those flaws. This is why curating diverse and high-quality data is so critical.
- High-quality data leads to more reliable outputs.
- Biased data can perpetuate harmful stereotypes.
- Incomplete data risks producing irrelevant or nonsensical results.
The phrase "garbage in, garbage out" perfectly sums up how vital data quality is to generative AI systems.
How Algorithms Influence Outcomes
An algorithm is essentially the set of instructions that guides the AI in making decisions. Different algorithms can lead to vastly different outcomes even when using the same dataset. For example:
- Some algorithms prioritize speed, potentially sacrificing depth or nuance.
- Others focus on precision but may require more computational resources.
- The choice of algorithm can amplify or mitigate biases in the data.
Developers must carefully design and test these algorithms to ensure they align with the intended use of the AI, as even minor oversights can lead to significant errors.
Challenges in Measuring AI Performance
Evaluating the performance of generative AI is no small feat. Unlike traditional software, where outcomes are predictable, generative AI outputs are probabilistic and often subjective. This makes it hard to establish clear metrics for success. Common challenges include:
- Defining "accuracy"—is it about factual correctness, user satisfaction, or something else?
- Accounting for edge cases—rare scenarios where the AI might fail spectacularly.
- Balancing performance across multiple languages or cultural contexts.
Table: Common Metrics for AI Performance
Metric | Description |
---|---|
BLEU Score | Measures the quality of text generation |
Perplexity | Evaluates how well a model predicts text |
User Satisfaction | Subjective but critical for usability |
Measuring AI performance isn’t just about numbers; it’s about understanding how the technology interacts with real-world complexities.
Legal Accountability in Generative AI Errors
Who Bears Responsibility for AI Mistakes?
Assigning responsibility for errors made by generative AI is a tangled issue. Should the blame fall on the creators, the users, or the AI itself? Some argue that developers should shoulder the responsibility since they design the algorithms and provide the training data. Others point to the users, especially in cases where misuse or negligence plays a role. Then there’s the idea of shared accountability, where responsibility is distributed across the AI’s lifecycle—from creation to deployment and maintenance. This model ensures that no single party bears the full weight of liability, but it also complicates legal frameworks.
The Debate Over AI as a Legal Entity
Can AI be treated like a corporation or an individual under the law? This question is at the heart of whether AI can be held accountable for its mistakes. Legal entities like companies can be sued, fined, or held liable for damages, but AI currently exists as property, not as an entity with rights or responsibilities. Some legal theorists propose granting AI limited personhood to handle liability issues, while others reject this notion, arguing that AI lacks consciousness and intent, making it fundamentally different from humans or corporations.
Case Studies of Legal Disputes Involving AI
Several high-profile cases have already tested the waters of AI accountability. For example:
- Copyright Infringement: Generative AI models like ChatGPT and image generators have faced lawsuits from authors and artists claiming their works were used without permission.
- Product Liability: If an AI-powered medical device makes an incorrect diagnosis, who is at fault—the manufacturer, the hospital, or the software developer?
- Defamation and Misinformation: AI systems have been accused of generating false or defamatory content, leading to legal challenges over who should be held accountable.
The legal landscape surrounding AI mistakes is still evolving, and courts are often left grappling with outdated laws that don’t fully address the complexities of this technology.
In the meantime, the conversation continues about how to balance innovation with accountability in a way that protects both creators and the public.
Ethical Dilemmas in Generative AI Accuracy
Bias and Fairness in AI Systems
Generative AI often inherits biases from the datasets it’s trained on. This "garbage in, garbage out" problem has led to real-world consequences. For example, a U.K. university admissions algorithm in 2018 disproportionately disadvantaged students from certain socioeconomic backgrounds. This sparked outrage and showed how biased AI can reinforce social inequalities.
Addressing bias is tricky because fairness isn’t a one-size-fits-all concept. What seems fair to one group might disadvantage another. Developers and ethicists are grappling with these challenges, but progress is slow. Here are some key questions:
- Should AI aim to replicate societal norms or challenge them?
- How do we define fairness in a diverse world?
- Who decides what’s fair?
The Black-Box Problem in AI Decision-Making
AI systems, especially complex ones, often operate as "black boxes." Even developers can’t always explain how certain decisions are made. This lack of transparency raises serious ethical concerns. Imagine an AI denying a loan or misdiagnosing a patient without a clear explanation—who takes responsibility?
The "black-box problem" isn’t just a technical issue; it’s a trust issue. If people can’t understand or question AI decisions, how can they trust them?
To tackle this, some experts advocate for transparent AI models. Others suggest mandatory audits to ensure accountability. However, implementing these solutions is easier said than done.
Ethical Frameworks for AI Accountability
Creating ethical frameworks for AI is like building a plane while flying it. The technology evolves faster than our ability to regulate it. Current efforts focus on:
- Transparency: Making AI operations understandable.
- Accountability: Ensuring someone is responsible for AI decisions.
- Inclusivity: Designing systems that consider diverse perspectives.
While these principles sound good on paper, applying them in practice is challenging. For instance, how do you balance transparency with protecting proprietary algorithms? Ethical dilemmas like these will only grow as AI becomes more integrated into society.
For a deeper dive into how bias in generative AI impacts fairness and transparency, it’s clear that addressing these issues requires collaboration across industries and disciplines.
The Role of Regulation in Ensuring AI Accuracy
Current Legal Gaps in AI Governance
Right now, there’s a legal gray area when it comes to AI. Most laws weren’t written with AI in mind, and they struggle to address the unique challenges it brings. For example, if an AI system makes a mistake—like providing harmful medical advice or misidentifying someone in a criminal investigation—who’s legally responsible? Is it the developer, the company using the AI, or the AI itself? The lack of clear guidelines leaves a lot of room for confusion and potential harm.
Some countries, like those in the European Union, are starting to roll out AI-specific regulations. The EU's Artificial Intelligence Act, for instance, aims to hold developers accountable for harm caused by their systems. But even these efforts have limitations. AI evolves over time, learning and adapting after it’s deployed. So, what happens if an AI causes harm after it’s "learned" something new? These are questions that current laws don’t fully address.
Proposed Models for Shared Accountability
One idea gaining traction is shared accountability. This approach spreads responsibility across everyone involved in an AI system’s lifecycle:
- Design and Development: Developers must ensure their algorithms are transparent and free from harmful biases.
- Deployment: Companies deploying AI need to monitor its real-world use and address issues promptly.
- Post-Deployment: Regular updates and audits should be mandatory to catch and fix problems as the AI evolves.
This model emphasizes collaboration between developers, users, and regulators. It’s not perfect, but it’s a step toward making sure no one escapes accountability.
The Future of AI Regulation
Looking ahead, governments and organizations need to work together to create global standards for AI. Right now, different countries are taking different approaches. The U.S. focuses more on encouraging innovation, while the EU prioritizes consumer protection. Meanwhile, some developing nations are rushing to adopt AI without fully understanding its risks. This patchwork of regulations could lead to loopholes and inconsistencies.
To make AI truly safe and reliable, we need a unified approach that balances innovation with accountability. Without it, the risks of harm and misuse will only grow.
One promising direction is the idea of "regulatory sandboxes." These are controlled environments where companies can test AI systems under the watchful eye of regulators. This not only encourages innovation but also helps identify potential issues before they become real-world problems. It’s a practical way to balance progress with safety.
The Human Factor in Generative AI Accuracy
The Programmer’s Role in AI Errors
Programmers are the architects of AI systems, and their decisions during development can significantly influence how these systems perform. Every line of code, every dataset chosen, and every algorithm designed carries the potential for errors or unintended consequences. For instance, if a programmer uses biased training data, the AI can inherit and amplify those biases.
Key ways programmers can unintentionally contribute to AI errors:
- Data Selection: Choosing datasets that don’t represent the diversity of real-world scenarios.
- Algorithm Design: Overlooking edge cases or failing to account for rare but impactful situations.
- Testing Limitations: Relying on insufficient or overly controlled testing environments.
Programmers must take extra care to anticipate potential pitfalls and implement safeguards. But even with the best intentions, mistakes can happen, highlighting the importance of collaboration and peer review in development teams.
User Responsibility in AI Interactions
Users often trust AI systems to perform tasks accurately, but this trust can sometimes be misplaced. While AI tools are designed to assist, they aren’t infallible. Users play a crucial role in ensuring the outputs are reliable by:
- Double-Checking Results: Especially in high-stakes scenarios like legal, medical, or financial applications.
- Providing Clear Inputs: Ambiguous or incomplete inputs can lead to unexpected outcomes.
- Understanding Limitations: Recognizing that AI is a tool, not a decision-maker, helps set realistic expectations.
If users blindly accept AI-generated outputs without scrutiny, errors can propagate unchecked. This shared responsibility underscores the need for better AI literacy among the general public.
The Impact of Human Oversight on AI Performance
Human oversight acts as a safety net for generative AI systems. By monitoring and intervening when necessary, humans can catch errors that the AI might miss. Oversight is particularly vital in:
- Critical Applications: Fields like healthcare, where mistakes can have life-or-death consequences.
- Continuous Learning: Providing feedback to improve AI accuracy over time.
- Ethical Decision-Making: Ensuring outputs align with societal values and norms.
Generative AI is only as good as the humans who build, use, and monitor it. Without active human involvement, the risk of errors grows exponentially.
In summary, while AI systems are incredibly powerful, their accuracy and reliability are deeply intertwined with human actions. Programmers, users, and overseers all share the responsibility of minimizing errors and maximizing the benefits of generative AI.
Technological Solutions to Improve Generative AI Accuracy
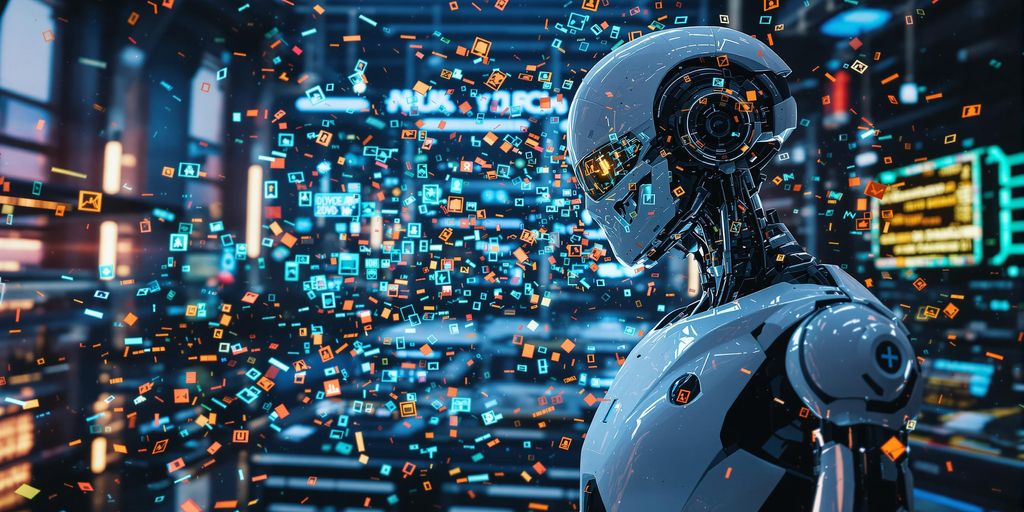
Advances in Algorithm Design
The foundation of any generative AI system is its algorithm. Improving algorithms is one of the most effective ways to enhance AI accuracy. Researchers are constantly exploring ways to make models more efficient, adaptable, and precise. For instance, techniques like reinforcement learning and transformer architecture refinements have shown promise in reducing errors. Additionally, hybrid models that combine neural networks with traditional rule-based systems are gaining traction for their ability to balance creativity with reliability.
The Importance of Transparent AI Systems
Transparency is key when it comes to generative AI. Users and developers alike need to understand how decisions are made. This is where explainable AI (XAI) comes into play. By making AI systems less of a "black box," stakeholders can identify and address errors more effectively. Transparency also builds trust, which is essential for wider adoption. For example, enterprise AI solutions often prioritize transparency to ensure their models align with business goals and comply with regulations. Enterprise AI can achieve greater accuracy and trustworthiness by focusing on tailored models and robust data governance.
Leveraging Feedback Loops for Better Accuracy
Feedback loops are a game-changer for improving AI systems. By incorporating user feedback, generative AI tools can learn from their mistakes and refine their outputs over time. This iterative process helps address issues like bias, inaccuracies, and irrelevance. Practical steps include:
- Allowing users to rate AI-generated content.
- Implementing automated systems to flag anomalies or inconsistencies.
- Regularly updating training data to reflect new information and changing contexts.
The more data an AI system interacts with, the smarter and more accurate it becomes. But it’s not just about quantity—it’s about the quality and diversity of that data.
By combining these technological solutions, the future of generative AI looks brighter, with fewer errors and more reliable outcomes.
The Societal Impact of Generative AI Errors
Economic Consequences of AI Mistakes
Generative AI errors can lead to some serious financial repercussions. Imagine a scenario where a financial AI system miscalculates market trends, leading to massive investment losses. Or think about an e-commerce platform using AI to set prices inaccurately, driving away customers or causing revenue dips. Mistakes like these ripple through industries, affecting not just companies but also employees, shareholders, and consumers.
Here’s a quick breakdown of how these errors can hit the economy:
- Business Disruptions: AI-powered tools failing at critical moments can halt operations, especially in logistics or supply chains.
- Job Market Shifts: Errors might lead to layoffs or hiring freezes as companies scramble to recover.
- Consumer Trust: A single high-profile mistake can make people hesitant to rely on AI-driven services.
AI in Critical Sectors: Risks and Rewards
Generative AI is being used in high-stakes fields like healthcare, law enforcement, and transportation. While it promises efficiency and innovation, the risks are equally significant. For example, a self-driving car misinterpreting road signals or a diagnostic AI giving inaccurate medical advice could have life-threatening consequences.
Risks in Critical Sectors:
- Healthcare: Misdiagnoses or wrong prescriptions based on flawed AI recommendations.
- Law Enforcement: Predictive policing tools unfairly targeting certain communities.
- Transportation: Errors in autonomous vehicles leading to accidents or fatalities.
But it’s not all bad. When done right, generative AI can save lives, streamline processes, and reduce human error. The challenge lies in minimizing risks while maximizing rewards.
Public Perception and Trust in AI Systems
Public trust in AI is a fragile thing. One major incident, like a deepfake spreading false information during an election, can erode confidence in the technology. People might start questioning whether AI is worth the risks it brings.
Public trust isn’t just about whether AI works—it’s about whether people feel they can rely on it without being harmed or misled.
Building trust requires transparency. Companies need to show users how their AI systems work and what safeguards are in place. Without this, skepticism will grow, and adoption might slow down.
Wrapping It Up
So, where does this leave us? Generative AI is here to stay, and while it’s exciting, it’s also messy. Mistakes will happen, and figuring out who’s responsible won’t always be straightforward. Is it the developers? The companies using the tech? Or maybe even the AI itself? Right now, the rules aren’t clear, and that’s a problem. As we keep pushing the boundaries of what AI can do, we also need to figure out how to handle the fallout when things go wrong. It’s not just about making smarter machines—it’s about making sure we’re ready to deal with their mistakes, too.
Frequently Asked Questions
What is generative AI and how does it work?
Generative AI is a type of artificial intelligence that creates new content, such as text, images, or music, based on patterns it has learned from data. It works by using algorithms to analyze data and then generate outputs that mimic the style or structure of the input data.
Why does generative AI sometimes make mistakes?
Generative AI can make mistakes because it relies on the data it was trained on. If the data is incomplete, biased, or flawed, the AI's outputs may also be inaccurate. Additionally, the algorithms may not always interpret the data correctly.
Who is responsible when AI makes an error?
Responsibility for AI errors can vary. It might fall on the developers who created the AI, the companies that deploy it, or even the users. Sometimes, legal systems struggle to define accountability clearly, especially when the AI acts independently.
Can AI be legally held accountable for its mistakes?
Currently, AI itself cannot be held legally accountable because it is not considered a legal entity. Responsibility typically falls on the humans or organizations involved in its creation, deployment, or oversight.
How can we reduce errors in generative AI systems?
Errors in generative AI can be reduced by improving the quality of training data, refining algorithms, and incorporating human oversight. Regular feedback and updates also help improve accuracy over time.
What are the risks of using generative AI in critical areas?
Using generative AI in critical areas like healthcare or law can lead to serious consequences if errors occur. For example, incorrect medical advice or flawed legal recommendations could harm individuals. This is why careful regulation and oversight are essential.