What Happens When AI Fails—Real-World Examples
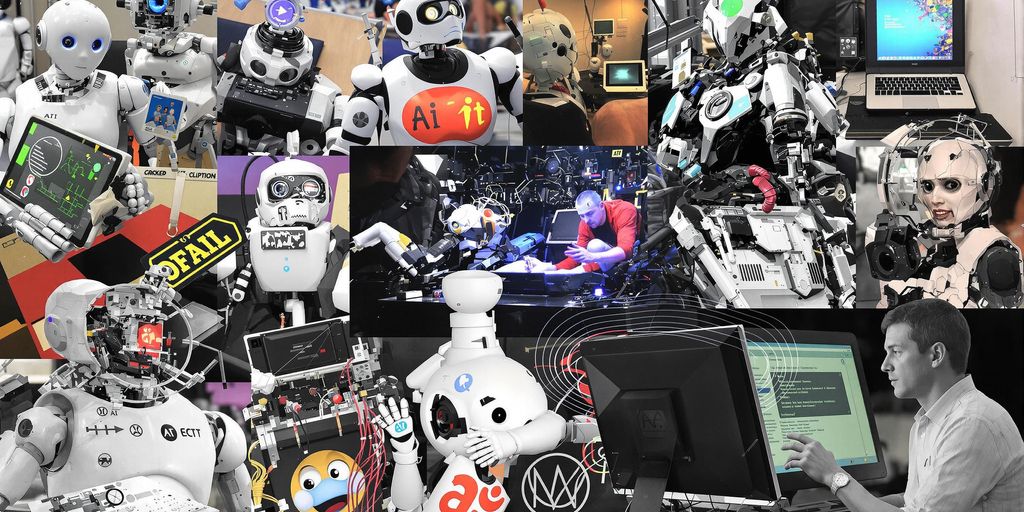
AI is everywhere these days, from helping doctors diagnose illnesses to deciding who gets hired for a job. But it’s not always smooth sailing. When AI messes up, the consequences can be huge, sometimes even dangerous. This post digs into some real-life examples of AI failures and what we can learn from them.
Key Takeaways
- AI failures can lead to significant real-world consequences, affecting millions of people.
- Algorithmic bias often results in unfair outcomes, especially for marginalized communities.
- Healthcare AI errors, like misdiagnoses, highlight the risks of relying too heavily on technology.
- Autonomous systems, such as self-driving cars, still face reliability and safety challenges.
- Building trust in AI requires transparency, accountability, and preparation for rare, unpredictable events.
Algorithmic Bias and Discrimination
Examples of Biased Decision-Making
Algorithmic bias occurs when AI systems produce unfair or unequal results due to flaws in their design or training data. For instance, a risk assessment algorithm used in U.S. courts was found to be biased against Black defendants. It wrongly labeled them as high-risk more often than white defendants, leading to harsher sentencing. Another case involved Amazon's AI-driven hiring tool, which discriminated against women because it was trained on resumes predominantly submitted by men.
Key examples include:
- A healthcare algorithm in 2019 that prioritized white patients over Black patients for critical treatments.
- Facial recognition systems misidentifying darker-skinned individuals more frequently than lighter-skinned ones.
- AI systems in hiring penalizing candidates based on gender or unrelated factors like zip codes.
Impact on Marginalized Communities
The consequences of algorithmic bias disproportionately affect marginalized groups. These biases can perpetuate systemic inequalities and limit opportunities for those already at a disadvantage. In healthcare, biased algorithms can mean life-or-death decisions are made unfairly. In hiring, it can block access to jobs for qualified candidates. And in criminal justice, it can lead to wrongful convictions or excessively harsh penalties.
When AI is biased, it doesn’t just mirror societal inequalities—it amplifies them, often on a much larger scale.
Efforts to Mitigate Bias in AI
Addressing algorithmic bias is challenging but not impossible. Steps to reduce bias include:
- Identifying and auditing all algorithms in use.
- Ensuring training datasets are diverse and representative of real-world populations.
- Regularly testing AI systems for unintended biases and errors.
Some organizations have already started implementing these measures. For example, researchers redesigned a biased healthcare algorithm, reducing its racial bias by 84%. This shows that while bias in AI is a serious issue, it can be tackled with deliberate effort and accountability.
AI Failures in Healthcare
Misdiagnoses and False Positives
AI has shown promise in diagnosing diseases, but its track record isn't spotless. One glaring issue is misdiagnosis, where AI systems either fail to detect conditions or flag healthy individuals as sick. For instance, some algorithms designed to identify skin cancer have struggled to distinguish between malignant conditions and benign skin anomalies. This kind of error can lead to unnecessary treatments, causing stress and financial burden for patients.
Challenges in Medical Data Training
The quality of AI in healthcare hinges on the data it learns from. Many systems rely on datasets that fail to represent diverse populations. For example, if an AI is trained primarily on data from one demographic, it may perform poorly when applied to others. This issue surfaced in a healthcare program that prioritized care for healthier white patients over sicker Black patients because it equated healthcare spending with illness severity. Clearly, biased data can have life-altering consequences.
Ethical Implications of AI Errors
When AI makes mistakes in healthcare, the ethical stakes are high. Who bears responsibility for a misdiagnosis—the developers, the medical professionals, or the AI itself? And what about transparency? Patients often don’t know how these systems arrive at their conclusions. This lack of clarity erodes trust and raises questions about accountability in life-and-death decisions.
The potential for AI in healthcare is immense, but its failures remind us that technology must be rigorously tested and ethically managed before it can truly transform patient care.
Breakdowns in Autonomous Systems
Self-Driving Car Accidents
Self-driving cars are often hailed as the future of transportation, but they’ve hit more than a few speed bumps along the way—sometimes literally. In one tragic incident in 2016, a Tesla Model S on autopilot failed to recognize a white truck against a bright sky, leading to a fatal crash. The system and the driver both missed the visual cue, highlighting a critical gap in AI's ability to process unpredictable real-world conditions.
A few key issues that contribute to these accidents include:
- Sensor Limitations: Cameras and radar systems sometimes struggle with adverse weather or unusual lighting conditions.
- Decision-Making Errors: AI systems can misinterpret road signs, pedestrians, or other vehicles’ actions.
- Overconfidence in AI: Drivers may rely too heavily on automation, assuming the system is foolproof.
Drone Malfunctions and Risks
Drones, whether for delivery or surveillance, face their own set of challenges. Malfunctions can lead to crashes, property damage, or even injuries. Common causes of drone failures include:
- GPS Signal Loss: Without a stable connection, drones can lose their way and crash.
- Battery Issues: Sudden power loss mid-flight is a frequent problem.
- Software Glitches: Errors in navigation algorithms can send drones off course.
These risks aren’t just technical; they’re also regulatory. How do you hold a machine accountable if it causes harm?
Lessons from Automation Failures
Every failure is a lesson waiting to be learned. From these incidents, the industry has started focusing on better redundancy systems and clearer safety protocols. For example, autonomous ships are now being designed with advanced decision-making capabilities to handle unpredictable scenarios like uncharted debris or erratic behavior from other vessels.
Automation isn’t perfect, but each failure pushes the technology closer to reliability. The key is balancing innovation with accountability, ensuring safety doesn’t take a backseat to progress.
AI Missteps in Customer Service
Chatbot Miscommunications
Customer service chatbots are supposed to make life easier, but sometimes they do the opposite. Imagine asking a chatbot for help, only to have it misunderstand your question entirely. One well-known incident involved a Chevrolet chatbot agreeing to sell a car for just one dollar due to a poorly designed system. Another case saw a delivery company’s chatbot swearing at a frustrated customer who had been trying to track a lost package. These examples highlight how poorly trained AI can cause major headaches instead of solving problems.
Voice Recognition Errors
Voice recognition systems are another area where AI tends to stumble. These tools rely heavily on understanding accents, dialects, and even background noise. When they fail, it can lead to embarrassing and frustrating situations. A fast-food drive-thru experiment by McDonald’s ended in disaster when the AI repeatedly misunderstood orders, adding hundreds of Chicken McNuggets to a customer’s bill. This failure not only annoyed customers but also went viral on social media, damaging the brand's reputation.
Impact on Brand Reputation
When AI fails in customer service, the fallout can be severe. Viral stories of chatbot blunders or voice recognition mishaps often lead to a loss of customer trust. Companies then have to invest significant resources to rebuild their image. Whether it’s a chatbot making legally binding mistakes or swearing at customers, these incidents show how crucial it is for businesses to carefully test their AI systems before rolling them out.
The Role of Black Swan Events
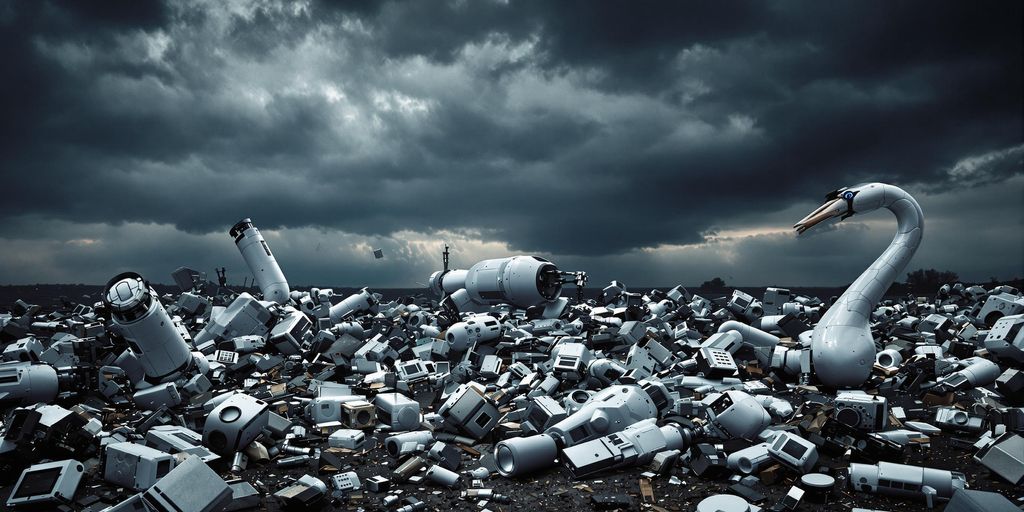
Unpredictable Scenarios in AI
Black swan events are those unexpected, rare occurrences that can completely disrupt systems—AI is no exception. These events are hard to predict, often because they stem from factors no one considered during the AI's design or deployment. For example, an AI system trained on historical data might fail catastrophically when faced with a scenario that’s never occurred before. This unpredictability makes it nearly impossible to prepare for every edge case.
Companies often assume their AI systems will operate within expected parameters, but when something truly novel happens—like a sudden shift in user behavior or an unforeseen interaction between systems—things can go wrong fast. Think about algorithms in stock trading; one unexpected market anomaly could trigger a cascade of errors, leading to real-world financial chaos.
Case Studies of Rare Failures
- Stock Market Flash Crashes: High-frequency trading algorithms have caused sudden, steep drops in stock prices due to unforeseen interactions between automated systems.
- Unintended Consequences in Healthcare: AI tools designed to prioritize patients for treatment have, in rare cases, excluded those most in need due to biases in training data.
- Autonomous Vehicle Anomalies: Self-driving cars have occasionally misinterpreted road conditions or signage in ways that human drivers would not, leading to accidents.
These examples highlight how even well-designed AI systems can fail when confronted with situations they weren’t built to handle.
Preparing AI for the Unexpected
Organizations can’t predict every black swan event, but they can take steps to limit the fallout:
- Diverse Training Data: Incorporate data from as many scenarios as possible to reduce blind spots.
- Stress Testing: Regularly test AI systems in simulated environments with extreme or unexpected conditions.
- Fail-Safe Mechanisms: Build in safeguards that allow systems to shut down gracefully when anomalies are detected.
Black swan events remind us that no matter how advanced AI becomes, it will never be infallible. Accepting this reality is the first step toward building systems that are resilient in the face of the unknown.
By acknowledging the potential for rare, high-impact failures, companies can adopt strategies to minimize risks and ensure their AI systems are as robust as possible. For more on this, consider how artificial general intelligence (AGI) could introduce entirely new challenges to this equation.
Transparency and Trust Issues
Lack of Explainability in AI
AI systems are often described as "black boxes." This means that even the engineers who create them may not fully understand how they arrive at certain decisions. This lack of clarity can erode trust, especially in high-stakes areas like healthcare or criminal justice. For example, if an AI system denies someone a loan or misdiagnoses a patient, people want to know why. Without clear explanations, it’s hard to hold these systems accountable or improve them.
Public Perception of AI Failures
When AI fails, the public’s reaction can be harsh. People expect technology to work flawlessly, and when it doesn’t, it can lead to skepticism or fear. Think about incidents like self-driving car accidents or biased hiring algorithms—they quickly make headlines and spark outrage. This negative perception can slow down adoption and innovation, as people become wary of trusting AI in their daily lives.
Building Trust Through Accountability
To build trust, companies need to step up their game in terms of transparency. Here are some ways they can do it:
- Document AI Development Stages: Keeping detailed records of how an AI system is built and trained can help identify weak spots.
- Provide Clear Explanations: Whenever possible, explain how AI decisions are made in simple terms.
- Admit and Address Failures: Acknowledging mistakes and showing steps to fix them can go a long way in rebuilding trust.
Trust isn’t built overnight, especially when it comes to something as complex as AI. But with the right measures, companies can show they’re serious about accountability and transparency.
AI in Criminal Justice
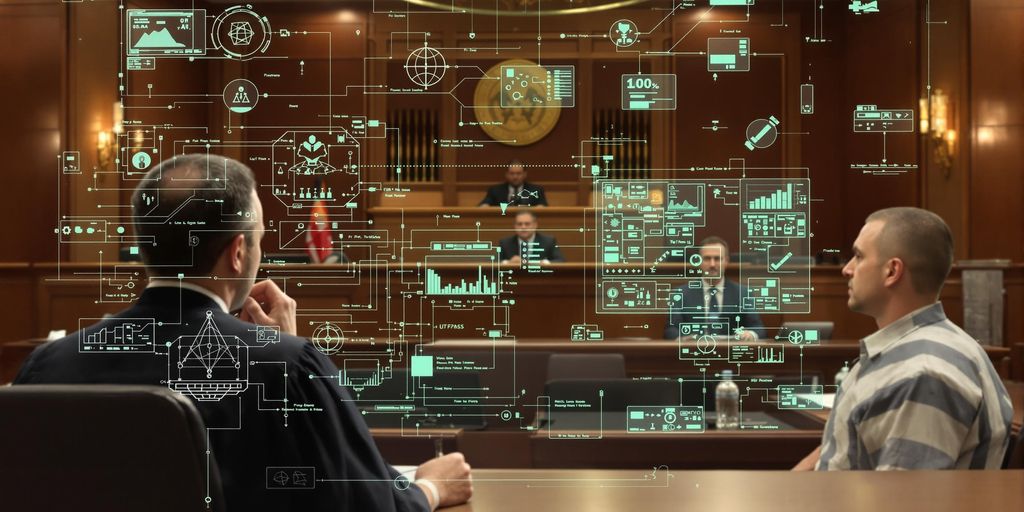
Bias in Risk Assessment Tools
AI-driven risk assessment tools are often used in courts to predict the likelihood of reoffending. However, these tools have shown significant biases. For example, one algorithm used in the U.S. was found to incorrectly label Black defendants as high-risk more often than white defendants. This led to harsher sentences and perpetuated systemic inequities. The lack of fairness in these tools raises questions about their reliability in high-stakes decisions.
Wrongful Convictions Due to AI
AI technology has also been implicated in wrongful convictions. A notable example is the case of Michael Williams, who was convicted based on data from an AI-powered gunshot detection system called ShotSpotter. The data was later found to be inaccurate, and Williams spent nearly a year in prison before his case was dismissed. This highlights the dire consequences of relying on unvalidated AI systems in the criminal justice system.
"When AI fails in legal contexts, it doesn’t just make mistakes—it changes lives, often for the worse."
Reforming AI Use in Legal Systems
To address these issues, reforms are urgently needed. Here are some steps that could improve the situation:
- Implement rigorous testing and validation processes for AI tools before their use in courts.
- Increase transparency in how AI algorithms make decisions, ensuring they can be scrutinized.
- Mandate regular audits to identify and correct biases in AI systems.
- Establish legal frameworks to hold developers accountable for AI errors.
By taking these measures, we can start to mitigate the risks posed by AI in criminal justice and work toward systems that are both fair and reliable.
Economic and Social Consequences
Job Displacement from AI Errors
AI is often hailed as a tool for efficiency, but when it fails, the consequences can ripple across industries. Job displacement caused by AI errors isn't just a hypothetical—it’s a growing reality. For instance, automation systems that inaccurately assess worker productivity may lead to unjust layoffs. When these systems make mistakes, real people lose their livelihoods.
- Misjudged performance metrics can result in wrongful terminations.
- Industries like manufacturing and logistics are especially vulnerable.
- The psychological toll on workers facing AI-driven job insecurity is immense.
Economic Costs of AI Failures
AI errors can be incredibly expensive for businesses. Take Zillow’s failed AI-based home pricing model as an example. By overestimating property values, the company faced significant financial losses and had to scale back its operations. Similarly, errors in AI-driven stock trading algorithms have led to market disruptions, costing millions in mere minutes.
Incident | Economic Impact |
---|---|
Zillow's pricing model | Hundreds of millions |
Flash crashes in trading | Billions in market loss |
Manufacturing shutdowns | Millions per day |
Social Backlash Against AI Technologies
When AI fails, public trust takes a hit. This backlash isn’t just limited to the tech itself—it extends to the companies deploying it. People question whether these tools are ready for widespread use, and skepticism grows. In some cases, communities have protested against AI surveillance systems, citing concerns over privacy and misuse.
The more AI stumbles in high-impact areas, the harder it becomes to convince society of its benefits. Trust is fragile and once broken, it’s tough to rebuild.
Conclusion
AI is a powerful tool, but it’s far from perfect. When it fails, the consequences can be small, like a wrong recommendation, or massive, like biased decisions affecting real lives. These examples remind us that while AI can do amazing things, it’s still a work in progress. Developers, companies, and even users need to stay cautious and keep asking questions about how these systems work. At the end of the day, AI is only as good as the people building and monitoring it. Let’s keep learning from its mistakes and push for a future where it works better for everyone.
Frequently Asked Questions
What is algorithmic bias in AI?
Algorithmic bias happens when AI systems make unfair decisions because they were trained on data containing biases. For example, this could lead to discrimination in hiring or loan approvals.
Can AI make mistakes in healthcare?
Yes, AI can misdiagnose diseases or give false positives. This happens when the data used to train the AI isn’t diverse or accurate enough, which can lead to serious health risks.
What are some risks with self-driving cars?
Self-driving cars can fail due to sensor errors, software glitches, or unexpected road situations. These failures can cause accidents and raise safety concerns.
How do AI chatbots sometimes fail?
AI chatbots can misunderstand questions or give irrelevant answers. This happens when they are not trained well or face unusual queries, which can frustrate users.
Why is AI transparency important?
Transparency helps people understand how AI makes decisions. Without it, trust in AI systems can decrease, especially when they make mistakes or unexpected choices.
What are black swan events in AI?
Black swan events are rare, unpredictable situations that AI systems are not prepared for. These events can cause AI to fail in unexpected and sometimes harmful ways.