What Are Foundation Models, and Why Do They Matter?
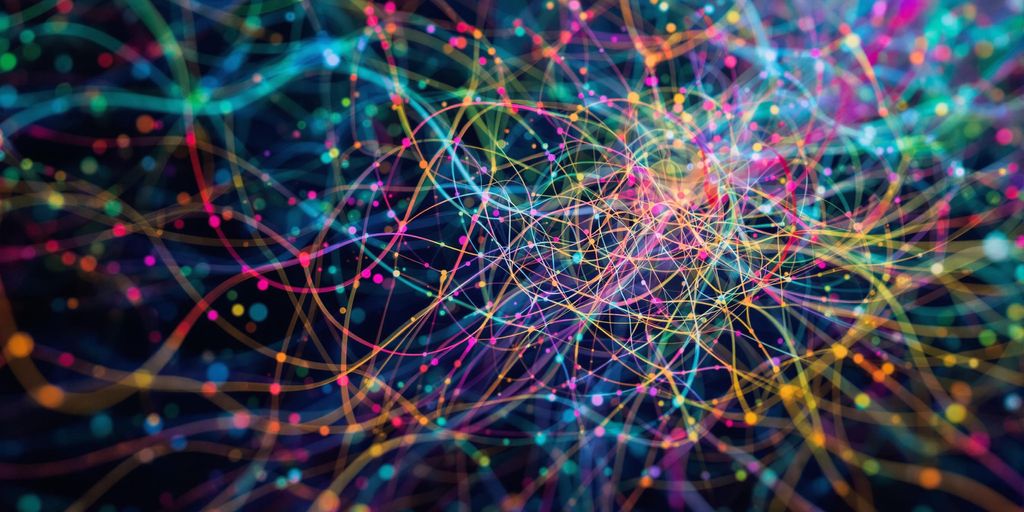
AI foundation models are changing the way we think about artificial intelligence. Unlike older systems that focus on just one task, these models are more like multi-tools—they can handle a variety of jobs with a little extra training. Whether it’s writing, analyzing data, or even creating art, foundation models are making waves across industries. But what exactly are they, and why should you care? Let’s break it down.
Key Takeaways
- AI foundation models are versatile systems trained on massive datasets to perform a wide range of tasks.
- They differ from traditional AI by being adaptable and scalable, making them useful in various industries.
- These models are already transforming fields like healthcare, business, and creative arts.
- Challenges include ethical concerns like bias and the environmental costs of training such large models.
- Understanding and using foundation models effectively can give businesses and individuals a competitive edge.
Understanding the Basics of AI Foundation Models
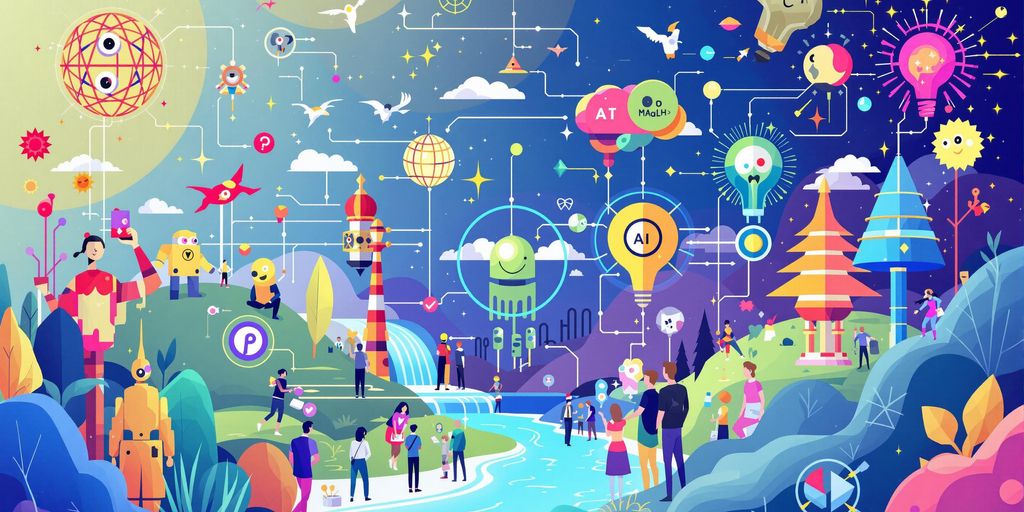
What Defines a Foundation Model?
Foundation models are a new class of artificial intelligence that operate as versatile, general-purpose systems. These models are trained on enormous and varied datasets, enabling them to perform a wide range of tasks without requiring extensive retraining. Think of them as the Swiss Army knife of AI—flexible and adaptable across different industries.
Unlike traditional AI, which is built for one specific job, foundation models are designed to handle multiple tasks. For example, a single model might write essays, translate languages, and analyze medical images. This adaptability stems from their ability to learn foundational knowledge that can be fine-tuned for specific applications.
How Foundation Models Differ From Traditional AI
Foundation models stand apart from traditional AI in several key ways:
- Scale of Training: Foundation models are trained on massive datasets that span multiple domains, whereas traditional AI typically focuses on smaller, task-specific datasets.
- Generalization: These models can adapt to new tasks with minimal additional training, unlike traditional AI, which often needs to start from scratch.
- Efficiency: By serving as a base for various applications, foundation models reduce the need to develop separate models for each task.
For instance, a traditional AI model might excel at identifying spam emails but fail if asked to summarize a text. A foundation model, on the other hand, could handle both tasks with ease after minor adjustments.
The Evolution of Foundation Models
The concept of foundation models has evolved rapidly over the past few years. Initially, AI systems were narrow and specialized, focusing on one task at a time. However, the surge in computational power and the availability of diverse datasets have paved the way for these more advanced systems.
Key milestones in this evolution include:
- The Rise of Large Language Models (LLMs): Models like GPT and BERT demonstrated the potential of training on vast amounts of text data.
- Multimodal Capabilities: Recent advancements have enabled models to process and generate content across different formats, such as text, images, and audio.
- Cross-Industry Adoption: Foundation models are now being used in healthcare, finance, and even creative industries, showcasing their versatility.
The shift from narrow AI to foundation models represents a fundamental change in how we approach artificial intelligence, making it more accessible and impactful across diverse fields.
Key Features of AI Foundation Models
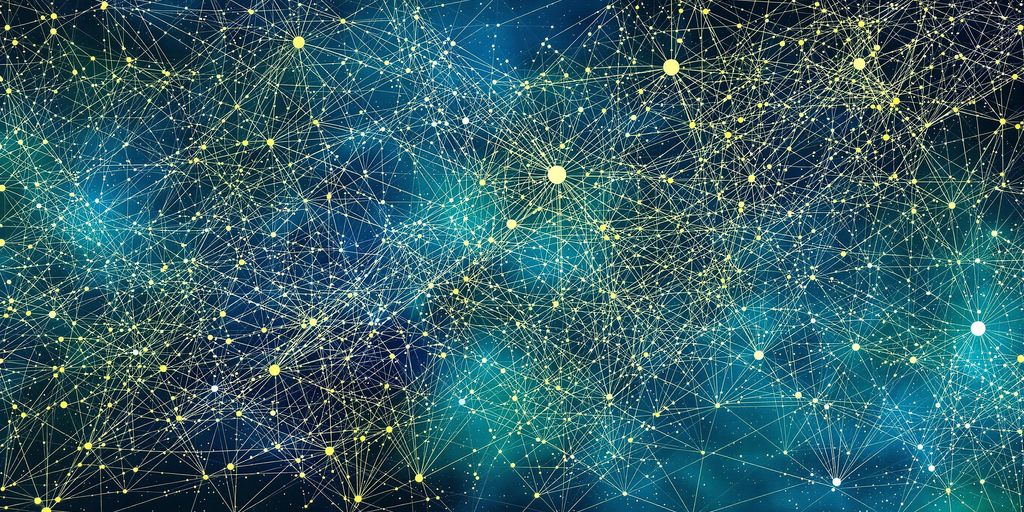
Adaptability Across Tasks
Foundation models are like the Swiss Army knives of AI. They’re designed to handle a range of tasks without needing to start from scratch each time. For instance, a foundation model trained on language can be fine-tuned to write essays, translate text, or even analyze customer reviews. This adaptability makes them incredibly versatile compared to traditional AI models, which are often locked into one specific job.
Key benefits of adaptability:
- Can be applied to multiple domains (e.g., healthcare, business, creative industries).
- Reduces the need for task-specific models, saving time and resources.
- Makes AI more accessible to industries with limited technical expertise.
Scalability and Efficiency
One of the standout features of foundation models is their scalability. These models can be trained on massive datasets, enabling them to process information at a scale traditional models can’t match. This ability to scale also means they’re more efficient when fine-tuned for specific tasks.
For example:
Feature | Traditional Models | Foundation Models |
---|---|---|
Training Dataset Size | Small, task-specific | Massive, diverse |
Task Focus | Narrow | Broad |
Reusability | Limited | High |
Generalization Capabilities
Unlike traditional AI, which often struggles outside its narrow focus, foundation models excel at generalizing knowledge. This means they can adapt to new tasks or datasets with minimal additional training. Think of them as having a strong “general knowledge” base that allows them to quickly learn new skills.
Advantages of generalization:
- Reduces the need for extensive retraining when applied to new tasks.
- Improves performance across a broader range of scenarios.
- Opens up opportunities for innovation in fields previously untouched by AI.
Foundation models represent a shift in how we think about AI—moving from narrowly focused tools to flexible systems capable of tackling diverse challenges.
Applications of AI Foundation Models in Real-World Scenarios
Healthcare Innovations Using Foundation Models
Foundation models are transforming healthcare, offering tools that go beyond traditional AI systems. They can analyze medical imaging, predict patient outcomes, and even assist doctors in creating personalized treatment plans. For instance, these models can identify anomalies in X-rays or CT scans with impressive accuracy. This adaptability is what sets foundation models apart, as they can be fine-tuned for multiple medical applications without starting from scratch.
- Detecting diseases like cancer in early stages using image recognition.
- Assisting in drug discovery by analyzing molecular data.
- Supporting telemedicine by enhancing automated patient interaction systems.
Foundation models are becoming a cornerstone in healthcare, bridging gaps in diagnostics and treatment efficiency.
Transforming Business Operations
Businesses are leveraging foundation models to boost efficiency and streamline operations. These models are used for automating customer service, generating insights from large datasets, and even creating marketing content. Companies save time and resources by using pre-trained models that can adapt to various tasks, from sentiment analysis to inventory management.
- Automating customer support with advanced chatbots.
- Analyzing consumer behavior to tailor marketing strategies.
- Enhancing supply chain management through predictive analytics.
Enhancing Creative Industries
In creative fields, foundation models are a game-changer. They help artists, writers, and designers by generating ideas, editing content, or even creating entirely new works. From writing scripts to designing graphics, these models are pushing the boundaries of what’s possible in art and media.
- Generating high-resolution images or videos based on text prompts.
- Assisting with scriptwriting or storyboarding for films and games.
- Creating music or soundscapes tailored to specific moods or themes.
The creative potential unlocked by foundation models is reshaping the way we think about art and storytelling.
These examples highlight the versatility of foundation models, making them indispensable across industries. For a deeper dive into their role in simulating real-world environments, check out World Foundation Models.
Challenges and Ethical Considerations of AI Foundation Models
Bias and Fairness in Foundation Models
Foundation models often inherit biases from the data they are trained on. If the training data is unrepresentative or skewed, the model may produce outputs that unfairly disadvantage certain groups. For instance:
- Data Bias: Using datasets that don't represent diverse populations can lead to skewed results.
- Development Bias: Decisions made during the creation of the model, like prioritizing certain features over others, can unintentionally embed unfairness.
- Unforeseen Influences: Other factors, such as evolving societal norms, can also impact fairness.
Addressing these biases requires a combination of better data collection practices and ongoing monitoring. Ensuring fairness isn't a one-time fix; it's a continuous process.
Environmental Impact of Large-Scale Training
Training foundation models demands enormous computational resources, which translates to significant energy consumption. This raises concerns about their environmental footprint. Here's what to consider:
- Energy Use: Training a single large-scale model can consume as much energy as a small town over several months.
- Carbon Emissions: Depending on the energy source, this can lead to substantial greenhouse gas emissions.
- Resource Allocation: The high costs and resources needed for training might limit access to only a few large organizations.
Organizations developing these models must explore energy-efficient training methods and prioritize sustainable practices to mitigate these impacts.
Regulatory and Policy Implications
Foundation models are a new frontier in AI, and existing regulations often don't account for their unique challenges. Policymakers face several hurdles:
- Defining Standards: What constitutes a "foundation model" and how should it be regulated?
- Accountability: Who is responsible for errors—model developers, downstream users, or both?
- Global Variability: Different countries may adopt conflicting regulations, complicating global deployment.
Balancing innovation with responsible oversight is tricky but essential. Without clear policies, the risks of misuse or harm could outweigh the benefits.
In conclusion, while foundation models hold great promise, addressing these challenges head-on is critical to ensure they are used responsibly and equitably.
The Future of AI Foundation Models
Emerging Trends in Foundation Model Development
AI foundation models are evolving fast, and the trends shaping their future are worth noting. First, expect more integration of multimodal capabilities, where models can process and understand multiple types of data—like text, images, and audio—simultaneously. This shift will make them even more versatile. Another trend? Smaller, more efficient models. Researchers are working on ways to reduce the size of these systems without sacrificing performance, which could make them accessible to startups and smaller organizations. Lastly, open-source foundation models are gaining traction, allowing broader collaboration and innovation.
Potential for Cross-Industry Disruption
These models aren't just for tech companies anymore. They're shaking up industries like healthcare, finance, and even agriculture. In healthcare, for example, they could analyze patterns in medical data to improve diagnostics and treatment plans. Foundation models are already being explored to enhance decision-making in medicine. Meanwhile, in business, they're automating tasks like customer support and market analysis. The ripple effect? Entire industries may need to rethink their workflows and strategies to stay competitive.
Preparing for a Foundation Model-Driven World
So, how do we get ready for this shift? For starters, education is key. Companies and individuals need to understand what these models can do and where they fall short. Investing in training programs will be essential. On a broader scale, policymakers and industry leaders must collaborate to address challenges like data privacy and ethical use. The goal should be to harness the power of these models responsibly while minimizing risks.
How to Leverage AI Foundation Models Effectively
Best Practices for Fine-Tuning Models
Fine-tuning foundation models allows you to adapt them to your specific needs. Here’s how to do it effectively:
- Understand your data. Ensure you’re working with clean, relevant, and diverse datasets.
- Set clear goals. Define what you want the model to achieve before starting the fine-tuning process.
- Iterate and test. Continuously refine the model and validate its performance on real-world tasks.
Fine-tuning is like customizing a suit—you start with a solid base and make adjustments to fit perfectly.
Integrating Foundation Models Into Existing Systems
Bringing a foundation model into your current workflow can feel overwhelming, but it doesn’t have to be:
- Assess compatibility. Check if the model aligns with your existing software and hardware.
- Start small. Begin with a pilot project to test feasibility and identify potential challenges.
- Train your team. Equip your staff with the skills to work with the new technology.
Collaborating With AI Experts
Sometimes, you need help to get the most out of these powerful tools. Here’s how to work with AI professionals:
- Find the right fit. Look for experts who understand both foundation models and your industry.
- Be clear about expectations. Communicate your goals and limitations upfront.
- Stay involved. Regularly review progress and provide feedback to ensure alignment with your objectives.
Pro Tip: Businesses can leverage generative AI by modernizing data infrastructure and upskilling teams, making it easier to integrate these models into their operations.
Comparing AI Foundation Models to Narrow AI Systems
Strengths and Weaknesses of Foundation Models
Foundation models are like the Swiss Army knives of AI—they’re designed to handle a wide range of tasks, from language translation to image recognition. Their biggest strength lies in their generalization capabilities, meaning they can adapt to many tasks with minimal retraining. This adaptability makes them suitable for industries requiring flexibility, such as healthcare or education. However, their broad nature can also be a drawback. Foundation models require substantial computational resources, both for training and deployment, making them less accessible to smaller organizations. They may also lack precision in niche tasks compared to highly specialized narrow AI systems.
Use Cases for Narrow AI vs. Foundation Models
Narrow AI systems excel in specific, well-defined tasks. For instance:
- Narrow AI: A banking model designed to predict loan defaults works efficiently for that purpose but can’t handle unrelated tasks like customer service.
- Foundation Models: These can be fine-tuned to perform multiple roles, such as analyzing financial risk and acting as a chatbot, with just slight adjustments.
Here’s a quick comparison:
Feature | Narrow AI | Foundation Models |
---|---|---|
Task Focus | Single, specific task | Broad, adaptable across tasks |
Training Data | Limited to specific context | Large-scale, diverse datasets |
Resource Intensity | Lower computational requirements | High computational demands |
Flexibility | Low | High |
Why Foundation Models Are a Game-Changer
Foundation models represent a shift in how AI is used. Instead of building a new model for every task, a single foundation model can be adapted to various applications. This reduces development time and opens up AI to more complex, multi-faceted problems. For example, in healthcare, a foundation model could assist in diagnosing diseases, summarizing medical literature, and even enhancing patient communication—all using the same core model.
Foundation models are not just tools; they’re platforms that enable innovation across industries by providing a flexible starting point for AI solutions. This adaptability is what sets them apart from traditional narrow AI systems.
Conclusion
Foundation models are shaping up to be a big deal in the world of AI. They’re not just another tech buzzword—they’re changing how we think about building and using AI systems. By starting with a strong, flexible base, these models make it easier to tackle all kinds of tasks, from writing essays to analyzing medical scans. Sure, there are challenges, like figuring out how to use them responsibly and making sure they work well for everyone. But as we learn more and refine these tools, the possibilities seem almost endless. Whether you’re in tech, healthcare, or just curious about where AI is headed, foundation models are something to keep an eye on.
Frequently Asked Questions
What is a foundation model?
A foundation model is a type of AI system trained on a massive amount of data to perform a wide range of tasks. Unlike traditional AI, which is designed for one specific job, foundation models can adapt to many different uses with minimal extra training.
How are foundation models different from traditional AI?
Traditional AI focuses on doing one task well, like recognizing faces or filtering spam emails. Foundation models, on the other hand, are built to handle many tasks by learning general knowledge that can be fine-tuned for specific purposes.
Why are foundation models important?
Foundation models are important because they save time and resources. Instead of creating a new model for every task, you can use a foundation model as a starting point and customize it for various applications, from healthcare to creative work.
What are some real-world applications of foundation models?
Foundation models are used in many areas, such as analyzing medical images, improving customer service with chatbots, creating art, and even helping businesses make better decisions by analyzing large datasets.
What challenges do foundation models face?
Some challenges include ensuring fairness and avoiding bias in the data they are trained on, managing the high costs and environmental impact of training large models, and addressing ethical concerns about their use.
How can I use foundation models effectively?
To use foundation models effectively, start by understanding your specific needs. Work with AI experts to fine-tune the model for your task, and make sure to integrate it properly into your existing systems for the best results.