Unlocking the Future of LLM Machine Learning: Exploring Innovations and Applications
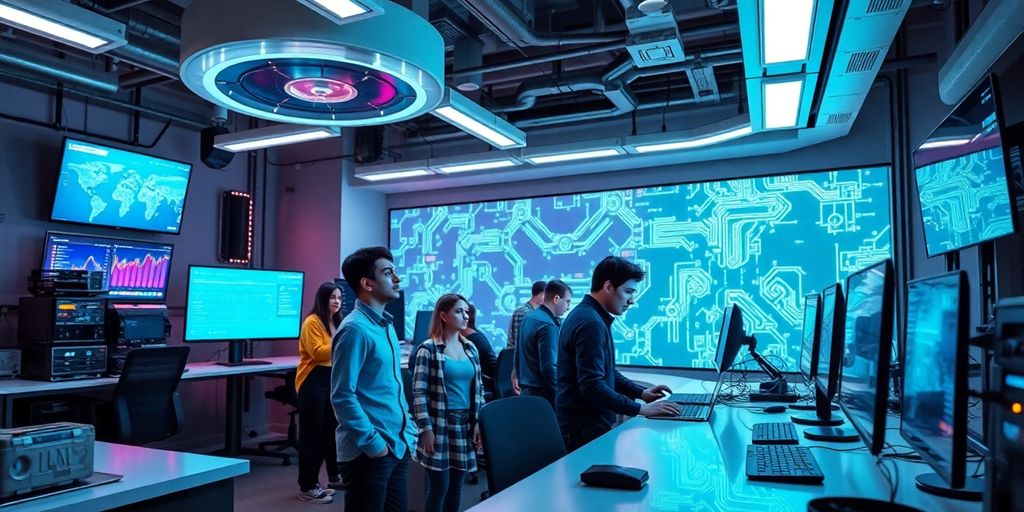
Hey there! Today, we're diving into the world of LLM machine learning. It's a big topic, but basically, it’s all about how these models are changing the game in tech. They're not just about crunching numbers or spitting out data anymore. Nope, they're doing way more than that. From helping doctors to making your customer service chat less frustrating, LLMs are everywhere. They’re getting smarter and more efficient, and who knows what they’ll do next? Let’s break it down and see what's happening with LLMs right now.
Key Takeaways
- LLMs are revolutionizing many fields, from healthcare to customer service.
- Techniques like Retrieval Augmented Generation and Few-Shot Learning are enhancing LLM performance.
- LLMs face challenges like data privacy and ethical concerns, which need addressing.
- Future LLMs might integrate new data types, like wearables, for more personalized experiences.
- Balancing model efficiency and specialization is crucial for future developments.
Innovative Techniques in LLM Machine Learning
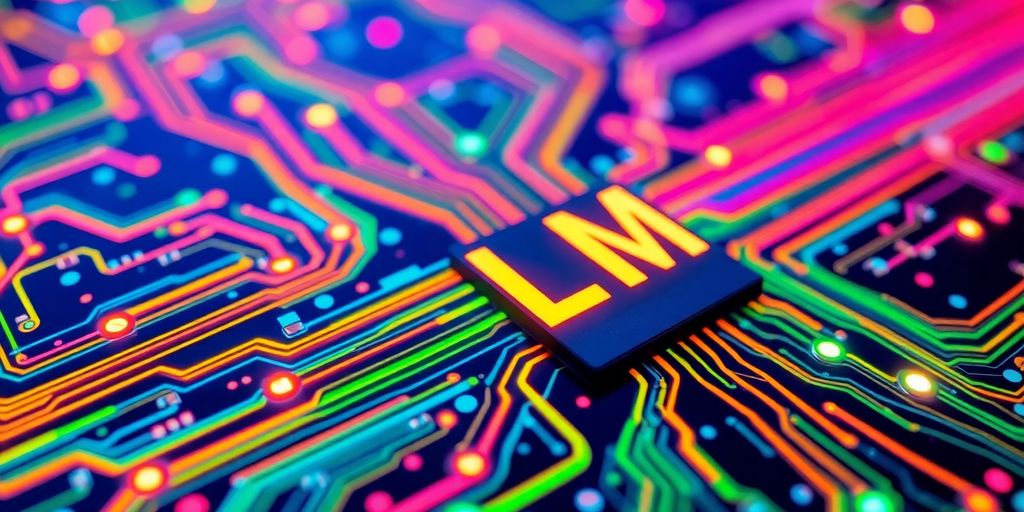
Exploring Retrieval Augmented Generation
Retrieval Augmented Generation (RAG) is changing how large language models (LLMs) handle data. Instead of relying solely on pre-stored information, these models can now pull in external data sources in real-time, creating more accurate and context-aware responses. Imagine asking a question about a recent event and the model fetching the latest data to provide an answer. This approach not only boosts accuracy but also keeps the model's responses fresh and relevant. RAG's ability to integrate live data is a game-changer for applications that need up-to-date information.
Advancements in Few-Shot Learning
Few-shot learning is a technique that allows models to learn new tasks with just a handful of examples. This is particularly useful when dealing with niche subjects or languages with limited data. By utilizing few-shot learning, LLMs can adapt to new domains quickly, without the need for extensive retraining. It's like teaching a dog a new trick after showing it just a couple of times. Few-shot learning is pivotal in making AI more flexible and accessible across diverse fields.
The Role of Quantization in Model Efficiency
Quantization plays a critical role in making LLMs more efficient. By reducing the precision of the numbers used in computations, models can run faster and require less memory, without significantly sacrificing accuracy. This is especially important for deploying LLMs on devices with limited resources, like smartphones. Think of it as packing a suitcase more efficiently, fitting everything you need without the extra bulk. Quantization ensures that even smaller devices can benefit from the power of LLMs, making advanced AI more accessible to everyone.
As LLMs continue to evolve, these innovative techniques not only enhance their capabilities but also broaden their applicability, making them indispensable tools in the AI toolkit.
Applications of LLM Machine Learning Across Industries
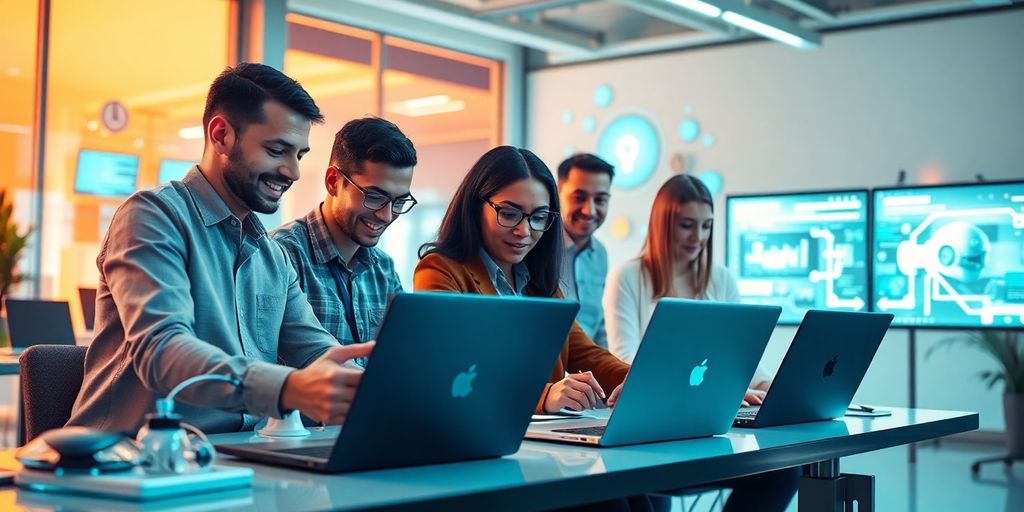
Revolutionizing Customer Service with LLMs
Large Language Models (LLMs) are shaking up customer service like never before. They're not just about answering questions anymore. These models can understand a customer's query, predict their needs, and provide solutions that feel almost human. Imagine a chatbot that doesn't just respond but actually engages with you, understanding the context and nuances of your request. This kind of interaction is becoming a reality, making customer service more efficient and personal.
- 24/7 Availability: LLMs don't need breaks, meaning they can provide constant support.
- Cost Efficiency: Reducing the need for large customer service teams.
- Personalization: Tailoring responses based on user history and preferences.
Enhancing Content Creation and Translation
LLMs are not just transforming how we write but also how we translate. From generating creative content like articles and stories to translating languages with context and cultural nuances, these models are doing it all. They're freeing up human creators to focus on big-picture ideas while handling the nitty-gritty details themselves.
- Creative Writing: Generating drafts for articles, blogs, and even books.
- Translation: Offering translations that go beyond word-for-word, capturing the essence of the language.
- Multilingual Content: Creating content that speaks to a global audience.
LLMs in Healthcare: Transforming Patient Care
In healthcare, LLMs are proving to be game-changers. They're helping with everything from managing patient records to analyzing complex medical data. By sifting through massive amounts of information quickly, they provide insights that can lead to better diagnosis and treatment plans. Efficiency in processing medical data allows healthcare professionals to focus more on patient care rather than paperwork.
- Data Analysis: Quickly analyzing patient records and research data.
- Diagnosis Assistance: Helping doctors identify potential health issues faster.
- Patient Interaction: Offering preliminary advice and information to patients, enhancing the overall care experience.
The integration of LLMs across various industries is not just a trend—it's a transformation. As these models continue to evolve, their ability to mimic human interaction and understanding will only grow, paving the way for more innovative applications.
Challenges and Limitations of LLM Machine Learning
Addressing Data Privacy and Security Concerns
When it comes to large language models (LLMs), data privacy and security are major issues. These models rely on huge datasets that often contain sensitive information. It's tricky to ensure that this data remains secure and private. Companies must take steps to protect data, like anonymizing it and implementing strict access controls. Balancing innovation with privacy is a tightrope walk.
- Data Anonymization: Removing personally identifiable information to protect user privacy.
- Access Controls: Limiting who can view or modify the data.
- Encryption: Ensuring data is secure both at rest and in transit.
The challenge is not just in protecting data but also in maintaining the integrity of the models that use this data. Finding a balance between data utility and privacy is crucial.
Overcoming Contextual Awareness Limitations
LLMs are great at generating text, but they struggle with understanding context. They often miss nuances that humans easily pick up. For instance, an LLM might not grasp sarcasm or cultural references. This lack of awareness can lead to misunderstandings or inappropriate responses. Enhancing contextual understanding is vital for improving LLM interactions.
- Nuance Recognition: Understanding subtle differences in meaning.
- Cultural Sensitivity: Adapting responses to fit cultural contexts.
- Real-time Learning: Continuously updating the model with new data to improve context awareness.
Ethical Considerations in LLM Deployment
Deploying LLMs comes with ethical concerns. These models can perpetuate biases found in their training data, leading to unfair or harmful outcomes. It's essential to address these biases to avoid reinforcing stereotypes. Developers must focus on creating more equitable models by:
- Bias Detection: Identifying and mitigating biases in training data.
- Inclusive Datasets: Ensuring diverse representation in data.
- Transparent Algorithms: Making model decision-making processes clear and understandable.
Ethical AI is not just about avoiding harm but actively promoting fairness and equality in technology.
The Future of LLM Machine Learning: Trends and Predictions
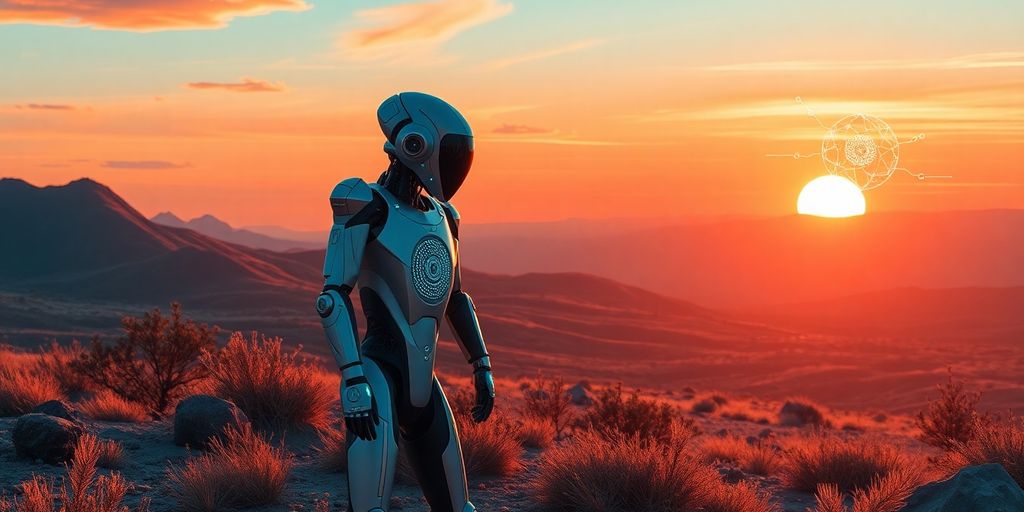
Scaling and Capabilities of Future Models
In the world of machine learning, the scaling hypothesis is gaining traction. It suggests that simply making models bigger and using more computational power leads to better results. This has been proven true for LLMs, and we can expect future models to continue this trend of improvement. But it's not just about size; it's about making models more efficient. Researchers are exploring ways to shrink models without losing their effectiveness, which could make LLMs more widely accessible.
The Impact of Multimodal LLMs
Multimodal LLMs are becoming a big deal. They combine different types of data, like text and images, to make smarter decisions. This approach is not just about understanding language but also about integrating various forms of information. As we move forward, we can expect these models to become more common, opening up new possibilities for applications across industries.
Personalization and User Experience Enhancements
Personalization is the future of LLMs. Imagine a model that understands your preferences and adapts to your needs. This is where LLMs are headed. By learning from user interactions, these models will offer more tailored experiences. This shift will change how we interact with technology, making it more intuitive and responsive to individual users.
As we look to the future, it's clear that LLMs will play a pivotal role in shaping how we interact with technology. Their ability to scale, integrate multiple data types, and personalize experiences will redefine user interactions, making technology more accessible and user-friendly.
The Relationship Between Generative and Predictive AI
Understanding Generative AI in LLMs
Generative AI, especially through Large Language Models (LLMs), is all about creating new content. These models can write essays, generate dialogue, or even compose music. They're designed to mimic human-like creativity by understanding patterns in language and then replicating them. Generative AI shines when it comes to producing text that feels natural and coherent, making it a go-to for applications like chatbots and creative writing tools.
The Role of Predictive AI in Enhancing LLMs
Predictive AI, on the other hand, focuses on forecasting outcomes based on data analysis. It’s about looking at historical data and predicting future trends. This might involve forecasting stock prices or predicting customer behavior. When paired with LLMs, predictive AI can provide context-aware responses. For instance, a chatbot might use predictive AI to anticipate a user's needs based on previous interactions, thus offering a more tailored experience.
Synergies Between Generative and Predictive Models
The combination of generative and predictive AI can lead to more sophisticated AI systems. Imagine an AI that not only generates responses but also predicts what you might need next. This synergy allows for more dynamic and responsive applications, enhancing user interactions across various platforms. In fact, Generative AI is emerging as a key business strategy, revolutionizing content creation and product design.
As AI technology evolves, the line between generative and predictive capabilities blurs, creating opportunities for more integrated and intelligent systems. These advancements promise to transform how we interact with technology, making it more intuitive and responsive to our needs.
Data Quality and Efficiency in LLM Machine Learning
The Importance of High-Quality Training Data
In the world of LLMs, data quality isn't just a buzzword—it's the backbone of the entire system. Imagine feeding a model with confusing or incorrect information; it's like teaching a child with a faulty textbook. High-quality training data is essential for creating models that are not only accurate but also reliable and unbiased. When the data is pristine, the model learns better, performs better, and can even adapt to various tasks more effectively.
- Ensuring high data quality is crucial for accurate, unbiased, and reliable models.
- Data quality significantly affects the performance, accuracy, and reliability of AI models, as discussed here.
- Poor data quality can lead to flawed outcomes, so employing effective data collection and processing techniques is vital.
Techniques for Improving Model Efficiency
Efficiency is the name of the game when it comes to large language models. These behemoths require immense computational power, but there are ways to trim the fat. Techniques like quantization can reduce the model's size without sacrificing much in terms of its output quality.
- Quantization allows models to be deployed on devices with limited computational resources.
- Efficient data processing techniques enhance LLM performance, as highlighted in this post.
- Innovations in LLMs are revolutionizing data engineering by accelerating ETL development and improving data quality, as outlined here.
Balancing Generalization and Specialization
Striking the right balance between generalization and specialization is a bit like walking a tightrope. On one hand, you want your model to be a jack-of-all-trades, capable of handling a wide variety of tasks. On the other, you want it to be a master in specific domains.
- The debate on whether to generalize or specialize is ongoing, with evidence suggesting focused models might excel in niche areas.
- Balancing these aspects remains a key challenge, as discussed in the context of multimodal LLMs.
As we progress, the quality of data and the efficiency of processing it will determine how far LLMs can go. The future is promising, but it requires careful navigation of these core aspects.
Exploring New Modalities in LLM Machine Learning
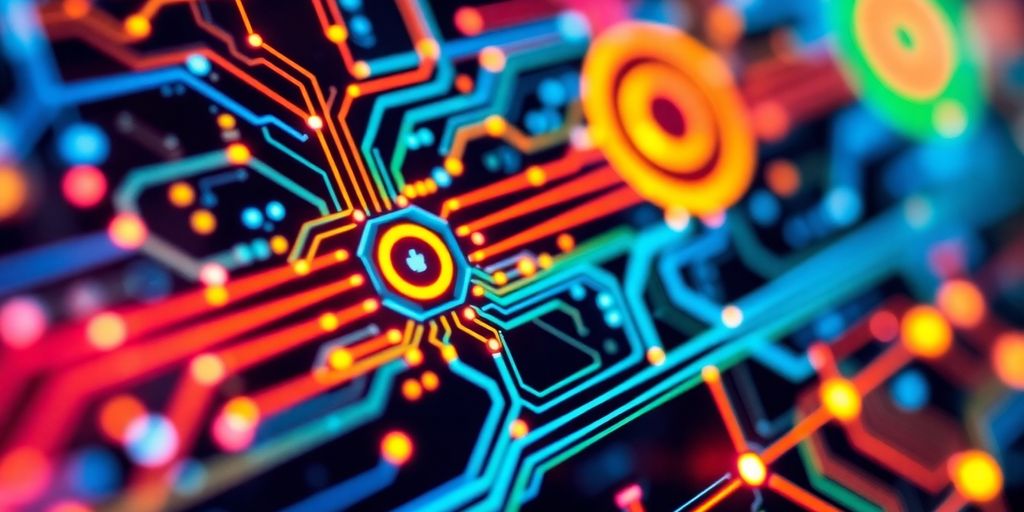
Integrating Wearable Data and Brainwave Patterns
Imagine a world where your smartwatch not only tracks your steps but also communicates with a language model to provide personalized health insights. This is the future we are stepping into. By integrating data from wearable devices and even brainwave patterns, large language models (LLMs) can offer more nuanced and real-time insights into our health and well-being. This could revolutionize healthcare diagnostics, making them more proactive and personalized.
- Wearables as Data Sources: Devices like smartwatches and fitness bands are already collecting vast amounts of data. The challenge lies in effectively integrating this data into LLMs to enhance predictive and diagnostic capabilities.
- Brainwave Integration: Brain-computer interfaces (BCIs) are the next frontier. By analyzing brainwave patterns, LLMs could potentially detect early signs of neurological disorders or even improve mental health treatments.
- Privacy Concerns: With great power comes great responsibility. As we integrate more personal data into LLMs, ensuring data privacy and security becomes paramount.
Multimodal LLMs combine the capabilities of models like GPT-4 to process various types of data, including text, images, and audio, enhancing the ability to understand and generate content across different modalities.
Innovations in Autonomous Vehicle Technology
Autonomous vehicles are no longer a thing of the future—they're here. And LLMs are playing a crucial role in making these vehicles smarter and safer. By processing data from multiple sensors, including cameras, LIDAR, and even audio inputs, LLMs help vehicles understand and navigate their environment more effectively.
- Sensor Fusion: Combining data from various sensors allows for a more comprehensive understanding of the vehicle's surroundings, improving decision-making processes.
- Real-time Processing: LLMs can process and analyze data on the fly, enabling vehicles to react quickly to changing conditions and potential hazards.
- Predictive Capabilities: By learning from past data, LLMs can predict and avoid potential accidents, making autonomous driving safer.
Machine learning and large language models offer businesses a chance to innovate and improve operations, supported by a comprehensive digital guide.
Expanding Horizons with Multimodal LLMs
The future of LLMs isn't just about processing text. It's about creating systems that can understand and interact with the world in a more human-like way. Multimodal LLMs are at the forefront of this transformation, bringing together text, images, audio, and more to create richer and more interactive experiences.
- Cross-Modal Understanding: Multimodal LLMs can interpret and generate responses that incorporate information from various data types, making interactions more natural and intuitive.
- Enhanced User Experiences: By integrating different modalities, these models can offer more personalized and engaging user experiences, whether in virtual assistants, gaming, or educational tools.
- Future Possibilities: As technology advances, we might see LLMs that can process even more complex data types, further blurring the lines between human and machine understanding.
New research focuses on advancing privacy-preserving machine learning, enhancing multimodal model capabilities, and improving large language model pretraining, while also exploring the implications of these advancements.
Conclusion
As we wrap up our exploration of the future of LLM machine learning, it's clear that we're standing on the brink of something big. These models are already changing the game in so many areas, from customer service to healthcare, and the potential for even more innovation is huge. Sure, there are challenges to tackle, like making sure these systems are fair and secure, but the possibilities are exciting. It's not just about making machines smarter; it's about finding new ways to solve problems and improve our lives. So, as we look ahead, let's keep pushing the boundaries and see where this technology can take us.
Frequently Asked Questions
What are Large Language Models (LLMs)?
Large Language Models, or LLMs, are advanced AI systems that understand and generate human-like text by learning from vast amounts of information. They are used in various applications like chatbots, content creation, and language translation.
How do LLMs help in customer service?
LLMs enhance customer service by powering chatbots that understand customer queries and provide relevant responses, improving the overall customer experience.
What are some challenges faced by LLMs?
LLMs face challenges like data privacy concerns, understanding real-world context, and ensuring ethical use. They can sometimes generate biased or incorrect information.
Can LLMs be used in healthcare?
Yes, LLMs can analyze medical records and research papers, helping doctors with insights for patient care and treatment plans.
How do LLMs learn new tasks?
LLMs learn new tasks by being trained on large datasets. They use techniques like Few-Shot Learning to quickly adapt to new information with minimal examples.
What is the future of LLMs?
The future of LLMs includes making them more efficient, reducing their size without losing quality, and expanding their use in various fields like medicine, law, and more personalized applications.