The Role of Predictive Analytics in Smarter Business Decisions
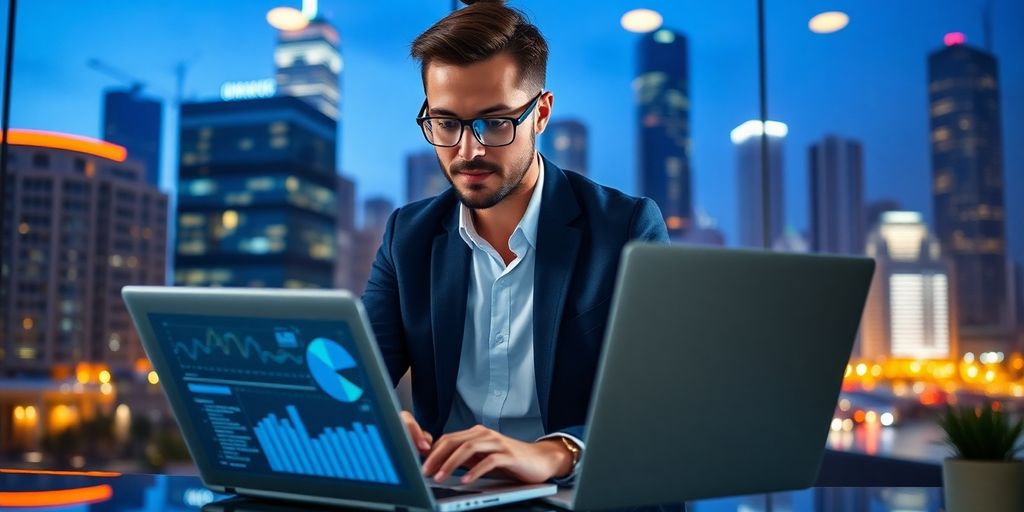
Predictive analytics is changing how businesses make decisions. By using past data to predict future outcomes, companies can make smarter choices, save money, and stay ahead in competitive markets. Whether it's improving customer service, cutting down risks, or planning better strategies, predictive analytics helps businesses work more efficiently and confidently.
Key Takeaways
- Predictive analytics uses historical data to forecast future trends, helping businesses make informed choices.
- It plays a vital role across industries like retail, healthcare, and finance, improving efficiency and outcomes.
- Challenges like data quality, privacy concerns, and organizational resistance must be addressed for effective implementation.
- Businesses can benefit from predictive analytics by reducing risks, improving customer satisfaction, and optimizing operations.
- The future of predictive analytics includes advancements in AI, real-time data usage, and broader applications across sectors.
Understanding Predictive Analytics and Its Business Impact
What Is Predictive Analytics?
Predictive analytics is all about using historical data to make educated guesses about the future. It combines techniques like statistical modeling, machine learning, and data mining to identify patterns and trends. These insights help businesses answer questions like, "What might happen next?" or "How likely is this outcome?" By shifting decision-making from reactive to proactive, predictive analytics empowers businesses to stay ahead of the curve.
Key Components of Predictive Analytics
Predictive analytics has a few building blocks that make it work effectively:
- Data Collection: You need reliable historical data to start. This could be sales numbers, customer feedback, or even weather patterns.
- Modeling: Algorithms and statistical models analyze the data to find relationships and patterns.
- Validation: Before using the predictions, the model needs to be tested to ensure accuracy.
- Deployment: Once validated, the model is applied to real-world situations to guide decisions.
How Predictive Analytics Differs from Traditional Analytics
Traditional analytics focuses on "what happened" by analyzing past events. For example, it might show that sales dropped last quarter. Predictive analytics, on the other hand, asks "what could happen?" It predicts future events like how sales might look next quarter if current trends continue. This forward-looking approach gives businesses a chance to prepare rather than just react.
Applications of Predictive Analytics Across Industries
Retail: Enhancing Customer Experience and Inventory Management
In retail, predictive analytics helps businesses anticipate customer preferences and manage inventory more effectively. By analyzing purchasing trends, retailers can forecast demand for specific products. This means fewer stockouts and less overstock, which translates into cost savings. For example, predicting seasonal demand allows stores to stock up on popular items just in time. This proactive planning ensures customers find what they need, enhancing satisfaction and loyalty.
Key Benefits in Retail:
- Accurate demand forecasting.
- Improved customer personalization.
- Reduced inventory waste.
Healthcare: Improving Patient Outcomes and Resource Allocation
Healthcare providers use predictive analytics to improve patient care and allocate resources efficiently. By studying historical patient data, hospitals can predict admission rates and identify high-risk patients who may need urgent attention. This ensures timely treatment and better resource planning. For example, predicting flu outbreaks can help allocate staff and supplies where they’re needed most.
Predictive analytics is transforming healthcare by enabling early intervention and more precise treatments, ultimately saving lives.
Key Benefits in Healthcare:
- Early identification of high-risk patients.
- Better resource management.
- Enhanced patient outcomes.
Finance: Risk Assessment and Fraud Detection
In the financial sector, predictive analytics is a game-changer for risk management and fraud detection. Banks analyze transaction patterns to identify anomalies that could signal fraud. Similarly, credit risk assessment models evaluate an applicant’s likelihood of repaying loans. This helps financial institutions make safer lending decisions and protect their assets.
Key Applications in Finance:
- Fraud detection via real-time data analysis.
- Creditworthiness evaluation for loan applicants.
- Identifying market trends to inform investment strategies.
Predictive analytics also plays a key role in safeguarding assets by identifying vulnerabilities before they become critical issues.
Benefits of Predictive Analytics for Smarter Decision-Making
Optimizing Operational Efficiency
Predictive analytics takes the guesswork out of managing resources. By analyzing historical data and identifying trends, businesses can streamline their operations. For example, a delivery company can predict when vehicles will need maintenance, avoiding costly breakdowns. Similarly, retailers can use predictive models to adjust inventory, ensuring shelves are stocked with what customers actually want, while cutting down on waste. This kind of precision not only saves money but also boosts productivity.
Enhancing Customer Retention and Satisfaction
Understanding your customers is key to keeping them around. Predictive analytics helps businesses anticipate customer needs and behaviors. For instance, it can flag customers who might be on the verge of leaving, giving companies a chance to engage them with tailored offers or better service. It also enables businesses to personalize experiences, like recommending products based on past purchases. When customers feel valued and understood, they’re more likely to stick around.
Reducing Risks Through Proactive Strategies
Risk management becomes a lot more effective with predictive analytics. By spotting early warning signs, businesses can address potential problems before they escalate. For example, financial institutions can assess credit risk more accurately, while manufacturers can identify equipment likely to fail. These proactive measures not only reduce costs but also protect a company’s reputation. In today’s fast-paced world, being able to act before an issue arises is a game-changer.
Predictive analytics doesn’t just help businesses plan for the future; it gives them the tools to shape it. From saving money to improving customer loyalty, the benefits are clear.
Challenges in Implementing Predictive Analytics
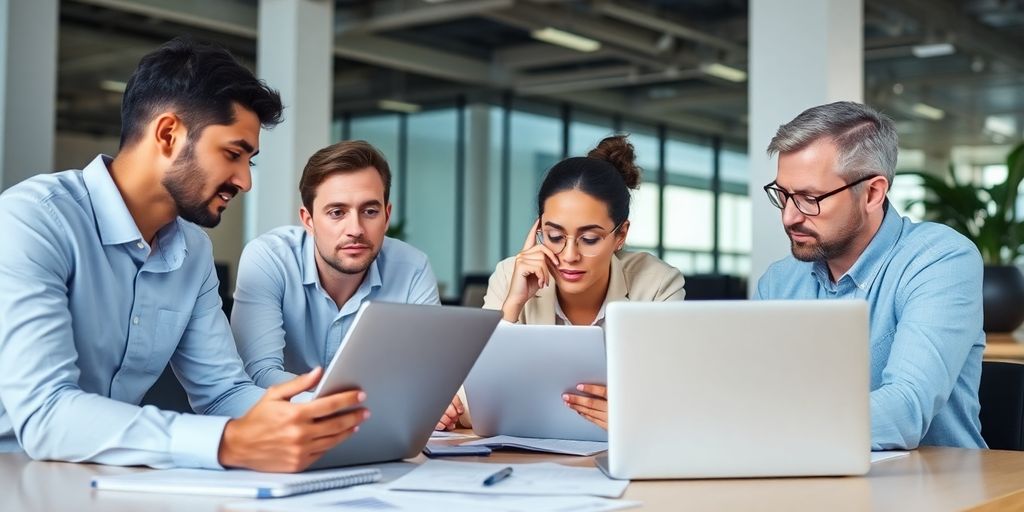
Data Quality and Accessibility Issues
One of the biggest hurdles in predictive analytics is dealing with poor data quality. If the data is incomplete, outdated, or inconsistent, the predictions will likely be unreliable. Businesses often struggle to collect and integrate data from various sources, which can create gaps or redundancies. For example, if customer data from a retail store doesn’t align with online purchase data, the predictive model might generate flawed insights. Ensuring data accuracy and accessibility is critical but can be time-consuming and costly.
Overcoming Organizational Resistance
Implementing predictive analytics isn’t just about technology; it’s also about people. Employees and stakeholders may resist change, particularly if they don’t understand the value of these tools. Some fear that predictive models might replace their jobs, while others simply don’t trust data-driven decisions over their own experience. To address this, businesses need to foster a culture of transparency and education. Hosting workshops or training sessions can help teams see the benefits and learn how to work alongside these tools effectively.
Addressing Ethical and Privacy Concerns
Using personal or sensitive data for predictive analytics raises ethical and privacy questions. Industries like healthcare and finance, for instance, must navigate strict regulations to ensure compliance. Misuse of data can lead to public backlash or legal consequences. Companies must establish clear policies on data usage, anonymize sensitive information, and regularly audit their practices. Striking the right balance between innovation and ethical responsibility is essential to maintain trust with customers and stakeholders.
Predictive analytics holds immense promise, but its success depends on addressing these challenges head-on. From ensuring high-quality data to building organizational trust and safeguarding privacy, businesses must take a comprehensive approach to make the most of this powerful tool.
Steps to Successfully Integrate Predictive Analytics in Business
Building a Data-Driven Culture
Creating a data-driven culture starts with leadership. Decision-makers need to emphasize the importance of data in shaping strategies and solving problems. This shift in mindset ensures that data becomes a core part of everyday operations. Encourage teams to rely on data insights rather than gut feelings when making choices.
- Communicate the value of data across all departments.
- Establish clear goals for analytics projects.
- Reward data-informed decision-making to reinforce the behavior.
Selecting the Right Tools and Technologies
Not all tools are created equal, so it’s crucial to pick ones that align with your business needs. Scalable cloud-based platforms like Python or Tableau can simplify analytics processes. If your team lacks expertise, consider collaborating with Data Analytics Consulting Services to ensure smooth implementation.
Tool/Technology | Purpose | Example Use Case |
---|---|---|
Python | Advanced data analysis | Building predictive models |
Tableau | Visualizing data insights | Creating dashboards for trends |
Cloud Platforms | Scalable data storage | Managing large datasets efficiently |
Training Teams to Leverage Predictive Models
Even the best tools are useless without skilled people to operate them. Invest in training programs to help your team understand predictive analytics. This includes data scientists, analysts, and even non-technical staff who need to interpret results.
- Identify skill gaps within your team.
- Offer training sessions or workshops.
- Encourage ongoing learning to keep up with evolving technologies.
Integrating predictive analytics isn’t just about tools or data—it’s about empowering your team to think ahead. When everyone understands the "why" and "how" behind analytics, businesses see real results.
The Future of Predictive Analytics in Business
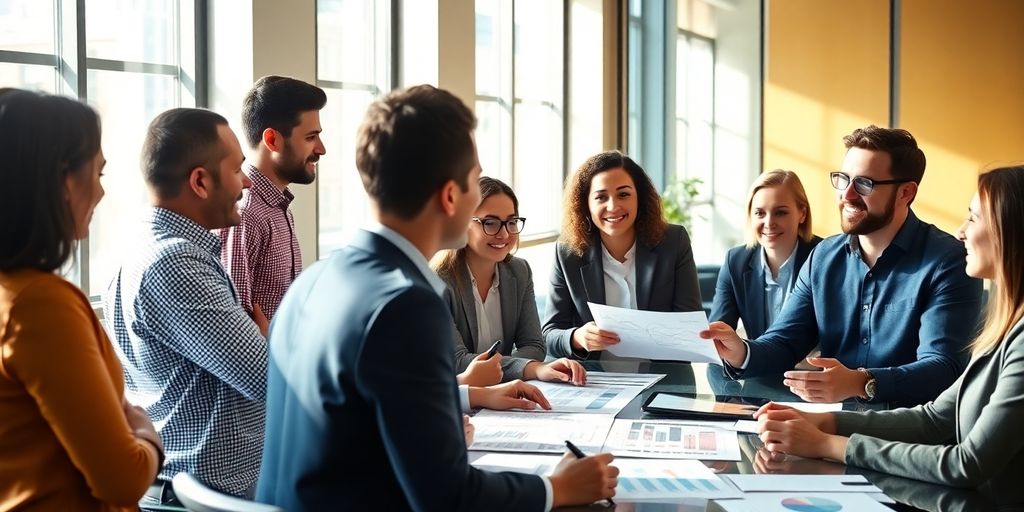
Advancements in AI and Machine Learning
Artificial intelligence and machine learning are reshaping predictive analytics by making models smarter and more accurate. AI-driven predictive tools can now process complex datasets faster than ever, delivering insights that were once impossible to achieve. These advancements mean businesses can make predictions with greater confidence, whether it’s forecasting demand or identifying risks. For instance, neural networks and deep learning algorithms are being fine-tuned to uncover hidden patterns, enabling better decision-making.
Real-Time Data and IoT Integration
The integration of predictive analytics with real-time data streams and IoT devices is a game-changer. Imagine a supply chain system that adjusts on the fly based on sensor data from warehouses or trucks. That’s the power of IoT paired with predictive analytics. Businesses can now react instantly to changes, reducing downtime and improving efficiency. Key industries like manufacturing and retail are already leveraging this to optimize operations and reduce costs.
Emerging Trends in Predictive Analytics Applications
The applications of predictive analytics are expanding into areas like personalized medicine, smart cities, and even climate change modeling. Here are some emerging trends:
- Ethical AI: Ensuring that predictive models are free from bias and comply with regulations.
- Explainable Models: Developing tools that make predictions easier to understand for non-technical users.
- Cost-Effective Solutions: As technology becomes more accessible, even small businesses can adopt predictive analytics.
The future of predictive analytics isn’t just about better tools; it’s about smarter strategies. Businesses that embrace these technologies will likely lead their industries, while those that don’t may struggle to keep up.
Conclusion
Predictive analytics is no longer just a buzzword—it's a real game-changer for businesses of all sizes. By using data to look ahead, companies can make smarter decisions, avoid risks, and find new opportunities. Sure, there are challenges, like making sure the data is good and the tools are set up right, but the benefits far outweigh the hurdles. Whether it's predicting customer needs, improving operations, or staying ahead of the competition, predictive analytics is helping businesses work smarter, not harder. As technology keeps advancing, the possibilities for what predictive analytics can do are only going to grow. If you're not already using it, now's the time to start thinking about how it could fit into your business.
Frequently Asked Questions
What is predictive analytics?
Predictive analytics is a method that uses past data to predict future events. It helps businesses make smarter decisions by identifying patterns and trends in the data.
How does predictive analytics differ from traditional analytics?
Traditional analytics focuses on understanding past events, while predictive analytics looks ahead to forecast what might happen in the future.
What industries can benefit from predictive analytics?
Industries like retail, healthcare, finance, manufacturing, and energy use predictive analytics to improve customer experience, reduce risks, and optimize operations.
What are the main challenges in using predictive analytics?
Common challenges include poor data quality, high implementation costs, privacy concerns, and resistance to change within organizations.
How can businesses start using predictive analytics?
Businesses can begin by building a data-driven culture, choosing the right tools, and training their teams to use predictive models effectively.
What is the future of predictive analytics?
The future includes advancements in artificial intelligence, real-time data integration, and new applications in areas like IoT and personalized customer experiences.