The Next Generation of AI Chips—Speed and Scalability
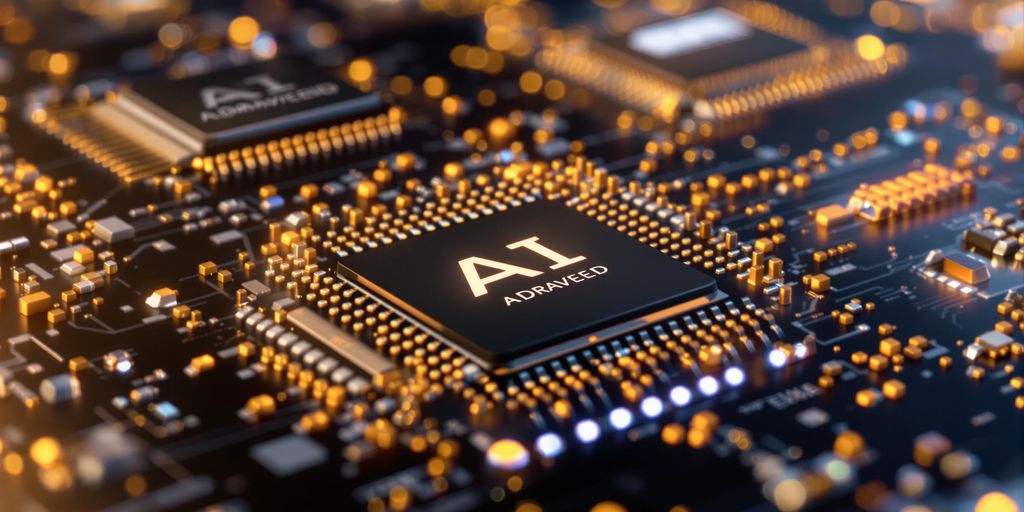
AI chips are reshaping the tech world, setting the stage for faster, more efficient computing. These tiny powerhouses are the backbone of modern AI systems, driving everything from advanced gaming to cloud infrastructure. As the demand for smarter and speedier AI grows, so does the race to innovate in chip technology.
Key Takeaways
- AI chips are crucial for the performance of modern AI systems, enabling faster computations.
- Energy efficiency is a major focus in AI chip development, reducing power consumption while boosting output.
- Big players like NVIDIA, Intel, and AMD are leading the charge in advancing AI chip capabilities.
- Emerging startups are introducing specialized solutions to address specific AI workloads.
- The future of AI chips includes innovations like memory-computation fusion and task-specific ASIC designs.
Revolutionizing AI Chips for Modern Workloads
Energy Efficiency in AI Chip Design
When it comes to AI chips, energy efficiency is a big deal. These chips are being pushed to handle massive workloads, but they can't guzzle power like there's no tomorrow. Modern AI chips are designed to deliver high performance without burning through energy. This is especially important as AI systems become more widespread, from data centers to edge devices.
Some key techniques driving energy efficiency include:
- Smaller transistors: Shrinking the size of transistors on a chip reduces energy leakage and boosts processing power.
- Specialized architectures: Chips tailored for AI tasks, like ASICs, can handle computations more efficiently than general-purpose processors.
- Dynamic power management: Adjusting power usage based on workload intensity helps conserve energy without sacrificing speed.
Overcoming Bottlenecks in Data Processing
AI workloads generate a ton of data. Processing all that information quickly is like trying to drink from a firehose—it’s tough. The latest AI chips are tackling this by focusing on faster data movement and smarter processing.
Here’s what’s being done to break through bottlenecks:
- High-bandwidth memory (HBM): Chips are integrating memory closer to the processing units, cutting down on delays.
- Parallel processing: Instead of working on one task at a time, AI chips now handle multiple tasks simultaneously.
- Better connectivity: Improved communication between different parts of the chip ensures data flows smoothly.
The Role of Government-Backed Research
Government funding is playing a big role in pushing AI chip technology forward. Think of it as a team effort: universities, private companies, and government agencies all working together to create the next big thing in computing.
Recent collaborations, like those between research institutions and the U.S. government, have led to breakthroughs in chip design. These projects aim to make chips that are not only faster but also more compact and energy-efficient.
Government-backed initiatives often focus on long-term challenges, like developing chips that can handle future AI workloads or creating manufacturing processes for smaller, more powerful transistors. These efforts are crucial for keeping up with the growing demand for AI computing power.
For more on the importance of AI chips in modern computing, the focus on energy efficiency and scalability is setting the stage for what’s next in the industry.
Top Players in the AI Chip Industry
NVIDIA's Leadership in AI Acceleration
NVIDIA has been the go-to name in AI chips for years, and for good reason. Their GPUs power everything from gaming rigs to massive AI models. Their CUDA platform is a game-changer, making it easier for developers to build and optimize AI applications. The company's latest H100 GPUs are designed specifically for handling generative AI workloads, offering unmatched speed and efficiency. As AI grows, NVIDIA continues to set the benchmark for performance and innovation.
Intel's Innovations in AI Hardware
Intel is no stranger to pushing boundaries, and their AI hardware lineup is no exception. Their Xeon processors and Habana AI accelerators are tailored for both training and inference tasks. Intel also focuses on integrating AI capabilities directly into CPUs, which simplifies deployment for businesses. With their next-gen chips, they aim to make AI more accessible while maintaining solid performance metrics.
AMD's Contributions to AI Development
AMD has been quietly making waves in the AI space. Their Radeon Instinct accelerators are designed with scalability in mind, making them a strong contender for AI workloads. AMD also emphasizes energy efficiency without compromising on power, which is crucial for data centers. As they continue to refine their hardware, AMD is carving out a niche in a market dominated by giants like NVIDIA and Intel.
Emerging Startups Transforming AI Chips
Cerebras Systems and Its AI Accelerator
Cerebras Systems, stationed in Sunnyvale, California, is making waves with what it calls the world’s fastest AI accelerator. This compact, 15-rack system is tailored to speed up large language models and is already being used globally in areas like enterprise AI, military intelligence, and cutting-edge research. Their approach emphasizes embedding AI functionality directly into hardware, creating a leap in performance. Backed by notable investors such as Sam Altman and Alpha Wave Ventures, Cerebras likens today's AI landscape to the early days of the internet—ripe for disruption and innovation.
Astera Labs' Connectivity Solutions
Astera Labs is tackling one of the most persistent challenges in AI: data bottlenecks. Their Scorpio X-Series Fabric Switches optimize connectivity within AI servers, ensuring CPUs, GPUs, and other components work in harmony. By streamlining memory access and data flow, their solutions help high-powered AI chips operate at near-maximum efficiency. These advancements are not just technical; they’re pivotal for industries relying on faster and more reliable AI systems.
The Rise of Specialized AI Chip Startups
Across the globe, smaller startups are challenging industry giants like NVIDIA and AMD. These companies are focusing on niche applications, such as energy-efficient chips and chips designed for specific AI workloads. This specialization allows them to carve out unique markets and push the boundaries of what AI hardware can achieve. Innovators in this space are proving that even small players can make a big impact by addressing gaps left by larger corporations.
The future of AI chips isn’t just about speed; it’s about solving real-world problems with smarter, more targeted solutions. Startups are leading the charge, rethinking the way AI hardware integrates with modern workloads.
The Future of AI Chip Architectures
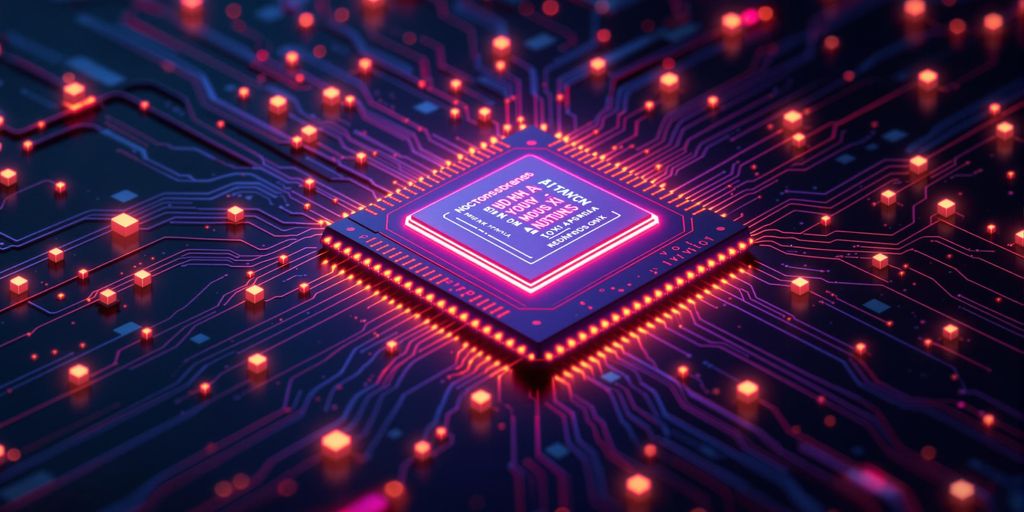
Fusion of Memory and Computation
The fusion of memory and computation is a game-changer in the AI chip world. By integrating memory directly into processing units, these chips reduce the need to constantly shuttle data back and forth. This not only speeds up operations but also cuts down on energy use. Imagine a chip that can think and remember at the same time—it’s like having a multitasking genius in your pocket. These designs are especially handy in edge devices, where space and power are limited but performance matters.
ASIC Chips for Specific AI Tasks
ASICs (Application-Specific Integrated Circuits) are becoming the go-to choice for specific AI workloads. Why? They’re designed to handle one task exceptionally well. Think of them as the specialists in a world of generalists. For example, ASICs excel in tasks like image recognition or language translation, where speed and efficiency are key. By 2030, it’s expected that most AI workloads will lean on ASICs because they’re just that good at what they do.
Programmable Chips for Scalability
On the other hand, we have programmable chips, which are all about flexibility. These chips can adapt to different tasks, making them ideal for companies that need to scale their AI operations. A startup today might use them for basic machine learning, but as they grow, the same chips could handle more complex tasks like deep learning. It’s like having a Swiss Army knife for AI—versatile and ready for anything.
The future of AI chip architectures is about balancing specialization with adaptability. While ASICs dominate specific tasks, programmable chips ensure scalability and flexibility for evolving needs.
Chip Type | Best For | Key Advantage |
---|---|---|
Fusion Chips | Edge devices, low power | Speed and energy-saving |
ASIC Chips | Specific AI tasks | High efficiency |
Programmable | Scalable AI workloads | Flexibility |
In the coming years, we’ll likely see a mix of these technologies working together, each playing to its strengths. Nvidia's Blackwell architecture is a great example of how innovation in this space is moving forward, blending speed and adaptability into its designs.
AI Chips and Their Impact on Industries
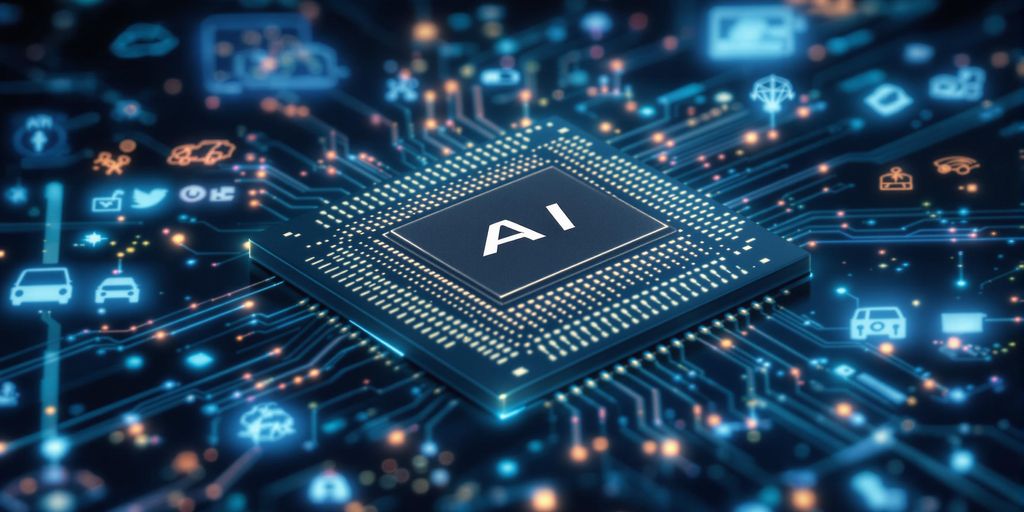
Advancing Generative AI Applications
AI chips are reshaping how generative AI tools operate, making them faster and more efficient. These chips enable complex models to generate high-quality content in real time. Whether it’s creating realistic images or crafting human-like text, the speed and precision AI chips bring to the table are unmatched. For example, industries like media and entertainment are already leveraging these advancements to automate creative processes, cutting down production time and costs.
AI Chips in Cloud Infrastructure
The backbone of modern cloud services relies heavily on AI chips. These chips handle the massive computational demands required for running AI models in data centers. Companies are now building specialized hardware to optimize cloud performance. Here’s how AI chips are transforming cloud infrastructure:
- Reducing latency for cloud-based AI services.
- Lowering energy consumption in data centers.
- Increasing the scalability of AI workloads.
Transforming Gaming with AI Hardware
Gaming is another industry seeing a huge shift thanks to AI chips. From powering realistic in-game physics to creating smarter non-player characters (NPCs), these chips are redefining player experiences. Gamers can expect more immersive worlds and faster load times as AI chips continue to evolve. Additionally, developers are now using AI hardware to create games more efficiently, automating tasks like texture generation and level design.
The global AI boom is driving a significant transformation in the semiconductor industry, leading to an unprecedented demand for specialized AI chips. This demand is not just reshaping technology but also redefining how industries function at their core.
Challenges in Scaling AI Chip Technologies
Balancing Performance and Cost
Scaling AI chip technologies isn't cheap. Companies face the tough challenge of balancing high performance with affordability. While advanced chips deliver incredible computational power, they often come with skyrocketing production costs. This creates a dilemma for manufacturers who want to innovate but also need to keep their products accessible. Finding that sweet spot between performance and cost is no easy feat.
- Advanced manufacturing processes, like those under 7nm, are expensive to develop.
- Specialized AI chips like ASICs are efficient but lack flexibility, limiting their cost-effectiveness.
- The high cost of materials and fabrication often drives up prices for end-users.
Addressing Power Consumption Issues
AI chips are power-hungry beasts. As these chips become more powerful, they demand more energy, which leads to higher operational costs and environmental concerns. The industry is grappling with how to make chips more energy-efficient without sacrificing performance.
Problem | Impact |
---|---|
High energy consumption | Increased operational costs |
Heat generation | Need for advanced cooling systems |
Environmental concerns | Larger carbon footprint |
The push for energy-efficient designs is more important than ever, especially as AI workloads grow in complexity. Companies that fail to address power consumption could face significant sustainability challenges.
Ensuring Compatibility Across Systems
Another hurdle is making sure AI chips can work seamlessly with different systems. Compatibility issues often arise when integrating new chips into existing infrastructures. For example, older hardware might not support the latest chips, leading to inefficiencies or the need for costly upgrades.
- Variability in system requirements makes standardization difficult.
- Legacy systems often require custom solutions, increasing development time.
- Software optimizations are needed to fully leverage new hardware capabilities.
Learn more about Nvidia's challenges in scaling its AI technologies.
Breakthroughs in AI Chip Performance
Doubling Throughput with New Designs
AI chips are evolving rapidly, with innovative designs that significantly boost throughput. New architectures are enabling chips to handle twice the data in the same amount of time. This is achieved by optimizing data pathways and integrating advanced memory technologies. For instance, smaller node sizes, a trend in the industry, are playing a critical role in improving both performance and efficiency.
Feature | Traditional Chips | Advanced AI Chips |
---|---|---|
Node Size (nm) | 14-28 | 5-7 |
Data Throughput (TB/s) | ~1 | ~2+ |
Power Consumption (W) | ~300 | ~150 |
Reducing Latency in AI Workloads
Latency has always been a bottleneck in AI processing. The latest AI chips address this by integrating specialized accelerators and reducing the distance data needs to travel within the chip. Techniques like fusing memory and computation are slashing delays, making real-time AI applications more feasible. This isn’t just about speed—it’s about enabling tasks that were previously impossible due to lag.
Enhancing Training and Inference Efficiency
The efficiency of AI training and inference has seen remarkable improvements. Chips are now tailored to specific tasks, such as using ASICs (Application-Specific Integrated Circuits) for dedicated workloads. This specialization reduces energy consumption and increases the speed of both training and inference.
- Training Improvements:
- Inference Gains:
These advancements are not just technical milestones—they're reshaping what AI can achieve, from powering generative AI to driving innovations in industries like gaming and cloud infrastructure.
By 2030, the AI chip market is projected to exceed $501.97 billion, driven by these breakthroughs and the adoption of smaller, more efficient nodes. AI chip market trends show how this evolution is shaping the future of technology.
Conclusion
The race to build the next generation of AI chips is heating up, and it’s clear that the future of artificial intelligence depends on these advancements. Companies big and small are pushing boundaries, creating faster, more efficient, and scalable solutions to meet the growing demands of AI workloads. Whether it’s improving energy efficiency, reducing costs, or tackling bottlenecks, the innovation happening today will shape how AI evolves tomorrow. As we look ahead, one thing is certain: the chips powering AI are just as important as the algorithms they run. The journey is just beginning, and the possibilities are endless.
Frequently Asked Questions
What is an AI chip?
An AI chip is a special type of computer chip designed to handle tasks related to artificial intelligence, like recognizing images or understanding speech. These chips are faster and more efficient than regular computer chips for such tasks.
Why are companies like NVIDIA and Intel important in the AI chip industry?
NVIDIA and Intel are leaders in creating advanced AI chips. They provide the hardware that powers many AI systems, helping industries like gaming, healthcare, and cloud computing to grow and innovate.
What makes startups like Cerebras Systems unique in the AI chip field?
Startups like Cerebras Systems focus on creating new and innovative AI chips. For example, Cerebras has developed one of the fastest AI processors, which helps speed up tasks like training AI models.
How do AI chips help reduce energy use?
AI chips are designed to be very efficient, using less power while still performing complex tasks. This helps save energy, especially when running large AI systems.
What challenges do AI chip makers face?
AI chip makers face challenges like balancing performance with cost, reducing power use, and making sure their chips work well with other systems.
How do AI chips impact industries like gaming and cloud computing?
AI chips make gaming more realistic with better graphics and smarter game characters. In cloud computing, they help process data faster, making services like virtual assistants and online tools work better.