Revolutionizing Patient Care: The Impact of Machine Learning in Medicine
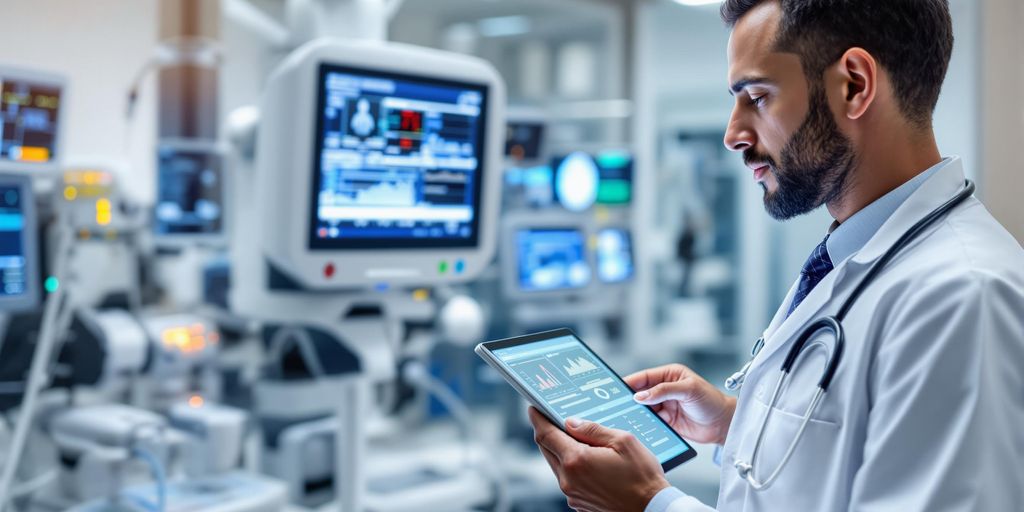
Machine learning is shaking things up in medicine. It's not just about robots and computers; it's about making real changes in how doctors treat patients and how hospitals run. From helping doctors make better decisions to predicting health issues before they happen, machine learning is becoming a big deal in healthcare. It's like having a super smart assistant that never sleeps, always ready to help out. But as with anything new, there are bumps in the road. Figuring out how to use this tech responsibly and making sure it helps everyone equally is a big part of the conversation.
Key Takeaways
- Machine learning can improve diagnostic accuracy, helping doctors make better decisions faster.
- Predictive analytics can lead to earlier interventions, potentially saving lives.
- Administrative tasks in healthcare can be streamlined, allowing more focus on patient care.
- Personalized treatment plans are more achievable with machine learning, tailoring care to individual needs.
- Ethical considerations, like data privacy and algorithm bias, are crucial in implementing machine learning in medicine.
The Role of Machine Learning in Modern Medicine
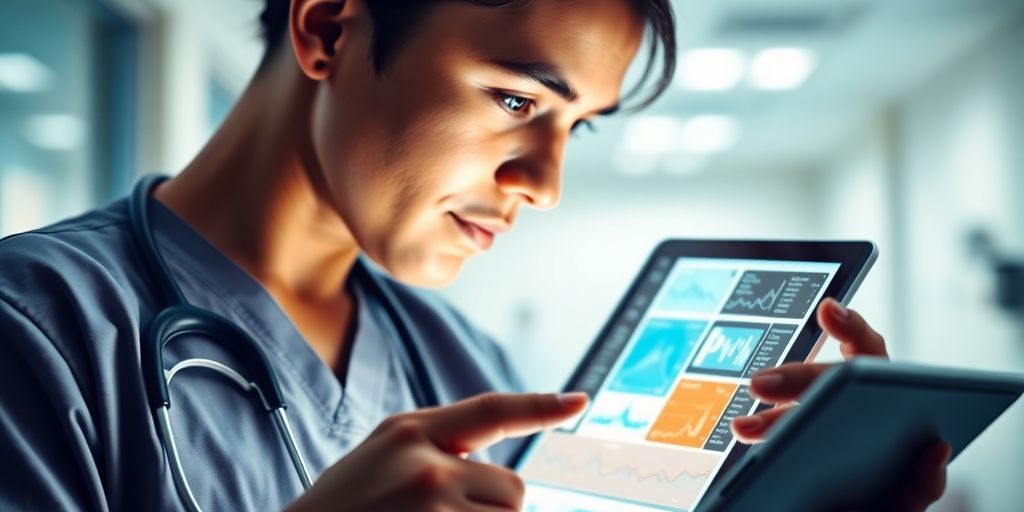
Enhancing Diagnostic Accuracy
Machine learning is really changing how we think about diagnosing diseases. It's like having a super-smart assistant that can sift through tons of data and spot things we might miss. For example, these algorithms can look at medical images and find subtle signs of problems way earlier than we could. This means patients get diagnosed faster and can start treatment sooner, which is a big deal. AI applications in healthcare improve efficiency by providing clinicians with immediate access to data, thereby enhancing patient safety during medication management AI applications in healthcare improve efficiency.
Predictive Analytics in Patient Care
Imagine being able to predict if someone might get sick before it even happens. That's what predictive analytics is doing with the help of machine learning. By analyzing historical data, these models can identify patterns that suggest a patient is at risk. This kind of foresight allows doctors to take preventive steps, which can be life-saving. It's like having a crystal ball, but way more scientific and reliable.
Streamlining Administrative Tasks
Let's face it, paperwork and admin tasks can be a real drag for healthcare workers. Machine learning is stepping in to help with that too. By automating routine tasks like scheduling and billing, it frees up time for healthcare professionals to focus on what they do best—caring for patients. Plus, it reduces human errors in these processes, making everything run smoother and more efficiently. AI and machine learning are increasingly utilized in pathology and medicine to improve diagnostic accuracy, streamline clinical processes, and tailor patient care AI and machine learning are increasingly utilized in pathology and medicine.
"Incorporating machine learning into medicine isn't just about technology; it's about reimagining how we deliver care. It's like giving the entire healthcare system a much-needed upgrade."
Transforming Patient Outcomes with Machine Learning
Personalized Treatment Plans
With the advent of machine learning, the approach to developing treatment plans has transformed dramatically. Every patient is unique, and machine learning helps in crafting personalized treatment plans by analyzing a vast array of data. From genetic profiles to lifestyle factors, machine learning algorithms can sift through this information to suggest the most effective treatments. This kind of precision medicine ensures that therapies are tailored to the individual, potentially increasing the success rate of treatments.
Real-Time Monitoring and Alerts
Machine learning plays a pivotal role in real-time patient monitoring. By analyzing data from wearable devices and other medical equipment, these algorithms can detect anomalies and alert healthcare providers instantly. This immediate response capability is especially crucial for managing chronic conditions, where timely interventions can prevent complications. Patients with conditions like heart disease or diabetes benefit immensely from this continuous monitoring, as it enables proactive care.
Improving Chronic Disease Management
Chronic diseases require ongoing management, and machine learning offers innovative solutions to enhance this process. By predicting disease progression, these technologies allow for early intervention strategies, which can significantly improve patient outcomes. Machine learning models can identify patterns and risk factors in patient data, helping healthcare providers to adjust treatment plans proactively. This not only improves the quality of life for patients but also reduces the overall burden on healthcare systems.
Machine learning isn't just a tool; it's a partner in healthcare, offering insights that were previously unattainable. By integrating these technologies into patient care, we're not only improving outcomes but also redefining the future of medicine.
Machine learning is transforming healthcare by enhancing patient outcomes, improving care quality, and speeding up healthcare delivery. As these systems become more sophisticated, they promise to bring even more advancements in patient care.
Machine Learning in Medical Imaging and Diagnostics
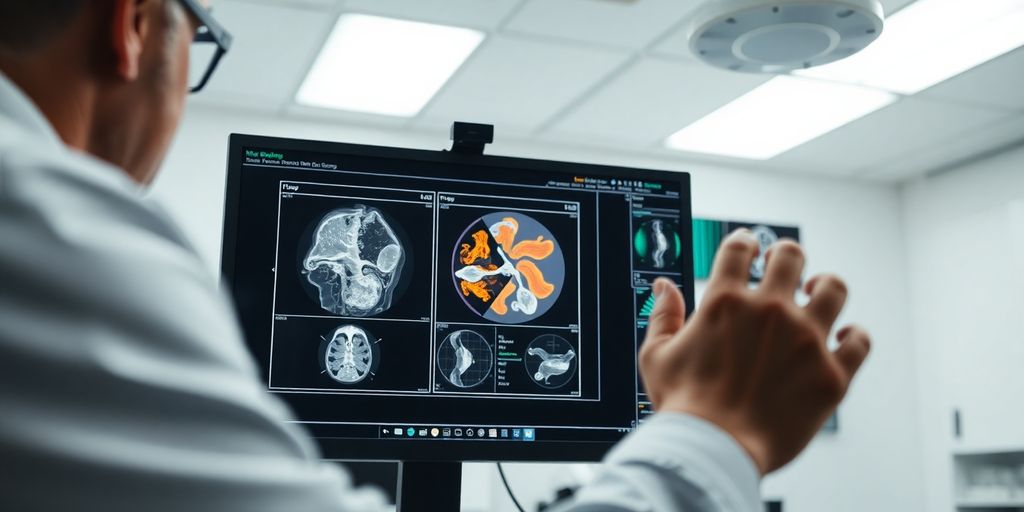
Advancements in Radiology
Radiology has seen a massive transformation thanks to machine learning. Algorithms, especially convolutional neural networks (CNNs), are now able to analyze and interpret complex medical images. These tools help radiologists spot abnormalities like tumors or fractures with accuracy that sometimes surpasses human capabilities. This doesn't mean radiologists are out of a job. Instead, they get to focus on more complex cases, leaving routine checks to the machines. This collaboration between humans and machines is a game-changer, speeding up diagnosis and reducing errors.
Early Disease Detection
One of the most exciting things about machine learning is its ability to catch diseases early. By analyzing patterns in medical images, algorithms can identify signs of diseases like cancer before they become apparent to the naked eye. This early detection is crucial, as it can lead to treatments that are more effective and less invasive. Studies have shown that AI systems can sometimes detect conditions like breast cancer more accurately than traditional methods, leading to fewer false positives and negatives.
Reducing Diagnostic Errors
Diagnostic errors are a significant concern in healthcare, often leading to delayed treatment or even harm. Machine learning is stepping in to help reduce these errors. By providing a second opinion or confirming a diagnosis, AI systems can enhance the accuracy of medical evaluations. This doesn't just improve patient outcomes; it also builds trust in the healthcare system. When patients know that their diagnosis is backed by both a doctor and advanced technology, it can be incredibly reassuring.
"The integration of machine learning in medical imaging is not about replacing doctors but about providing them with better tools to make informed decisions."
Ethical Considerations in Machine Learning Applications
Addressing Bias in Algorithms
Machine learning in healthcare is a game-changer, but it comes with its own set of issues. One big problem is bias. The data these algorithms learn from can be skewed, leading to unfair treatment. For example, if historical data shows a certain group getting less care, the algorithm might continue this trend. It's crucial to fix these biases to make sure everyone gets the same quality of care. Here are some ways to tackle this:
- Regular audits of algorithms to check for bias.
- Diverse data sets that include all demographics.
- Ongoing training for developers on ethical AI practices.
Ensuring Data Privacy and Security
When it comes to healthcare, keeping patient information safe is non-negotiable. Machine learning systems need a lot of data, and this can make them targets for data breaches. Protecting this data is a must. Encryption and strict access controls are just the start. Healthcare providers should:
- Implement robust cybersecurity measures.
- Regularly update and patch systems.
- Educate staff on data handling and security protocols.
Transparency in AI Decision-Making
AI in healthcare needs to be like an open book. Doctors and patients should understand how decisions are made. If an AI suggests a treatment, knowing why it did so builds trust. This transparency can be achieved by:
- Developing user-friendly interfaces that explain AI decisions.
- Involving healthcare professionals in the AI development process.
- Encouraging feedback from users to improve AI systems.
Machine learning holds great promise for healthcare, but it must be used responsibly. By addressing bias, ensuring privacy, and maintaining transparency, we can build trust and improve outcomes for everyone.
Machine Learning and Drug Discovery
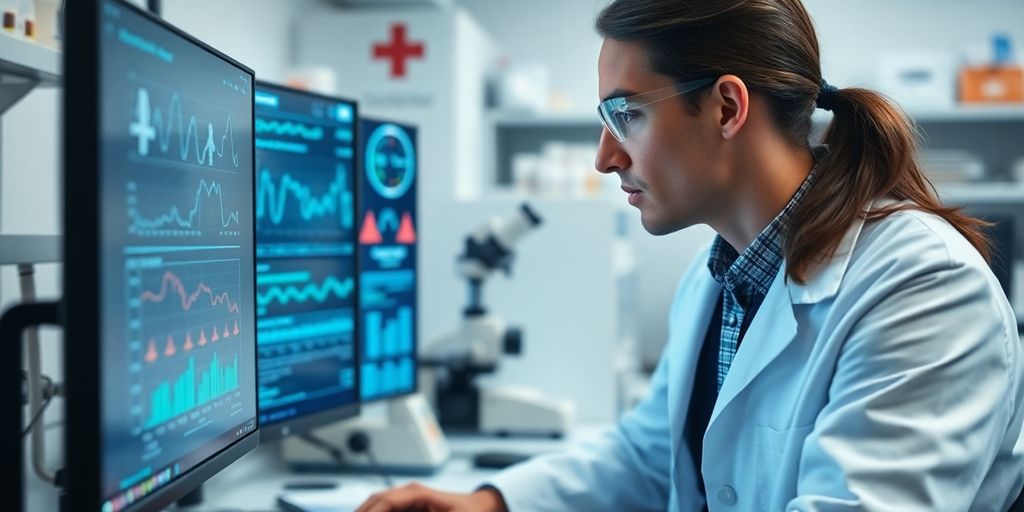
Imagine a world where finding new drugs doesn't take years. With AI in drug discovery, that's becoming a reality. Machine learning sifts through heaps of data, spotting potential drug candidates faster than ever. It looks at molecular structures and predicts how they might work in the body. This means less time in the lab and more time getting treatments to those who need them.
Predicting Drug Efficacy
Predicting how well a drug works before it's even tested in humans? That's the magic of machine learning. By analyzing past data, these algorithms can forecast a drug's success. They consider everything from genetic data to previous drug trials. This tech is making drug development not only quicker but smarter, ensuring only the most promising drugs move forward.
Personalized Medicine Approaches
One-size-fits-all medicine is on its way out. Thanks to machine learning, treatments are becoming as unique as the patients themselves. By examining a person's genetic makeup and health records, AI can suggest tailored treatments. This personalized approach promises better outcomes and fewer side effects. In the future, your prescription might be as unique as your fingerprint.
Machine learning is reshaping drug discovery, making it faster and more precise. It's not just about cutting costs; it's about saving lives with innovative treatments.
Challenges and Limitations of Machine Learning in Medicine
Data Quality and Integration Issues
Machine learning in healthcare heavily depends on having access to high-quality data. But let's be real, healthcare data is often a mess. It's fragmented, stored in all sorts of formats, and has errors or missing bits. This makes it tough for machine learning models to spit out accurate results. Ensuring data is clean, complete, and integrated is a huge hurdle. Without this, even the smartest algorithms can't do much.
Overcoming Technical Barriers
Implementing machine learning isn't just about having a fancy algorithm. There's a whole bunch of technical stuff to sort out. From choosing the right software and hardware to making sure everything talks to each other properly—it's a lot. Plus, there's the issue of making these systems user-friendly for healthcare professionals who might not be tech-savvy.
Balancing Human and AI Collaboration
AI can do some amazing things, but it can't replace human judgment. Doctors and nurses bring empathy, intuition, and experience that machines just can't match. The challenge is figuring out how to let AI handle the data crunching while humans focus on the personal touch. It's about finding that sweet spot where tech and human skills complement each other without stepping on toes.
Machine learning holds incredible promise for healthcare, but it's not a magic wand. Addressing these challenges head-on is crucial for realizing its full potential in improving patient care.
The Future of Machine Learning in Healthcare
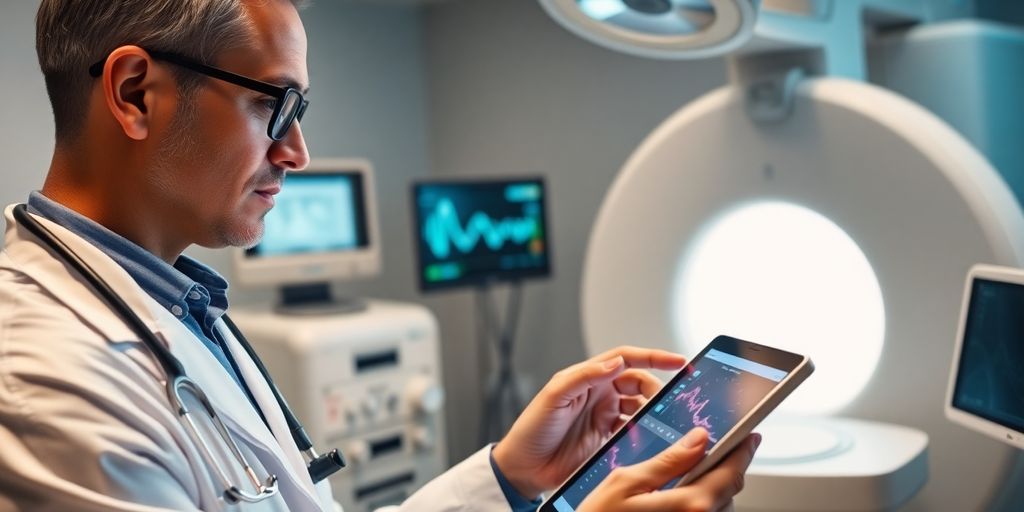
Emerging Trends and Innovations
Machine learning in healthcare is like a snowball rolling downhill, getting bigger and faster. It's reshaping the landscape with cutting-edge innovations. We’re seeing AI tools that predict patient outcomes, suggest personalized treatments, and even assist in surgeries. Imagine a world where your doctor uses an AI assistant to help make decisions. Crazy, right? But it's happening. And it’s not just about fancy gadgets; it's about improving patient care.
Potential for Global Health Impact
Machine learning isn’t just a game-changer for big hospitals in wealthy countries. Nope, it’s got the potential to revolutionize healthcare globally. In rural areas where doctors are scarce, AI can provide diagnostic support. Think about it: a villager in a remote area getting accurate health assessments using a smartphone app powered by AI. It’s like something out of a sci-fi movie, but it’s becoming a reality. The impact on global health could be massive, reducing healthcare disparities and improving access to quality care.
Integrating AI with Traditional Practices
Blending AI with traditional medicine is like mixing peanut butter and jelly—it just works. Doctors aren’t being replaced; they’re getting a sidekick. AI helps with data analysis, freeing up doctors to focus on patient care. It’s about collaboration, not competition. This integration is crucial for the future, ensuring that AI complements rather than competes with human expertise. The real magic happens when tech and tradition come together, enhancing the overall healthcare experience for patients and professionals alike.
Machine Learning's Impact on Healthcare Costs
Reducing Operational Expenses
Machine learning is shaking things up in healthcare, especially when it comes to cutting costs. Hospitals and clinics are using AI to streamline everything from scheduling to billing. This tech helps them figure out where they can save money without skimping on patient care. For instance, AI can predict when a machine might break down, so maintenance happens before it's an emergency. This proactive approach saves a ton of cash and keeps operations smooth. Plus, AI enhances early diagnosis in healthcare, leading to significant cost savings by improving the accuracy and speed of medical image analysis.
Optimizing Resource Allocation
Ever wonder how hospitals decide where to send their resources? Machine learning helps with that. By analyzing data, AI can suggest the best way to use staff, equipment, and even hospital beds. This means fewer wasted resources and more efficient care. It's like having a super-smart assistant that knows exactly what needs to go where and when. In fact, AI and machine learning can significantly lower healthcare costs by enabling early detection and prevention, ultimately leading to improved health outcomes.
Improving Efficiency in Care Delivery
Efficiency in healthcare is crucial, and machine learning is making it happen. AI tools can analyze patient data in real-time, providing doctors with insights that help them make quicker, more informed decisions. This not only speeds up care but also reduces the length of hospital stays, which is a big deal since Length of Hospital Stay (LOHS) is the primary factor driving inpatient costs. The result? Patients get better faster, and hospitals save money. It's a win-win for everyone involved.
Machine Learning in Enhancing Patient-Doctor Relationships
Improving Communication Channels
In today's fast-paced world, effective communication between doctors and patients is more important than ever. Machine learning is playing a pivotal role in enhancing communication by providing AI-generated answers to common medical queries. This not only saves time but also ensures that patients receive accurate information promptly. AI tools can analyze large volumes of data to extract relevant information, making it easier for doctors to communicate complex medical concepts in a way that patients can understand.
Empowering Patients with Information
Access to information is a key factor in empowering patients. Machine learning algorithms can tailor information to individual patient needs, improving health literacy and engagement. For instance, generative AI tools are enhancing patient health literacy by simplifying complex medical data into more digestible formats. This empowers patients to make informed decisions about their health and fosters a more collaborative relationship with their healthcare providers.
Building Trust Through Technology
Trust is the cornerstone of any doctor-patient relationship. By addressing blind spots and reducing biases, machine learning models can facilitate more transparent and fair decision-making processes. This builds trust by ensuring that patients feel heard and understood. Additionally, these models assist physicians by providing data-driven insights that can enhance the quality of care. They serve as valuable tools in healthcare, helping to bridge the gap between technology and human interaction.
Machine learning is not just about algorithms and data; it's about enhancing the human element in healthcare. By improving communication, empowering patients, and building trust, AI technologies are reshaping the patient-doctor relationship for the better.
Machine Learning in Public Health Initiatives
Predictive Modeling for Disease Outbreaks
Machine learning is changing how we predict and respond to disease outbreaks. By analyzing trends and patterns in health data, AI models can anticipate outbreaks before they happen. This means health officials can act faster, potentially saving lives. Predictive modeling uses vast amounts of data from various sources, including social media and travel records, to forecast where and when diseases might spread. This proactive approach is crucial for managing public health and avoiding widespread crises.
Enhancing Population Health Management
Managing the health of entire populations is a massive task, but machine learning is making it more manageable. AI tools can analyze patient data to identify those at risk of chronic diseases. This helps in targeting interventions more effectively, ensuring that resources are used where they're needed most. By focusing on prevention rather than treatment, health systems can improve outcomes and reduce costs. Machine learning also supports AI-driven methods to detect social isolation in vulnerable groups, offering targeted solutions to improve community health.
Data-Driven Policy Making
The use of machine learning in policy making allows for more informed decisions based on data analysis rather than intuition. By examining health trends, policymakers can craft strategies that are more likely to succeed. This includes understanding the health impacts of climate change and extreme weather events, where AI is enhancing clinicians' ability to predict and mitigate these effects. Data-driven policies can lead to more efficient use of resources, better health outcomes, and a more resilient public health infrastructure.
In the future, machine learning could revolutionize public health by providing insights that were previously impossible to obtain. As these technologies advance, they offer the potential to not only predict but prevent health crises, leading to healthier populations worldwide.
Machine Learning in Personalized Medicine
Tailoring Treatments to Genetic Profiles
Every person is different, and their response to medical treatments can vary widely. With the help of machine learning, doctors can now analyze a patient's unique genetic makeup to tailor treatments specifically for them. This approach, known as precision medicine, is changing the way we think about healthcare. By looking at genetic profiles, medical history, and even lifestyle factors, machine learning helps doctors choose the most effective treatment plans. It's like having a personalized roadmap to better health.
Optimizing Therapeutic Outcomes
Machine learning doesn't just stop at selecting treatments; it also plays a big role in optimizing therapeutic outcomes. By continuously monitoring patient data, such as vital signs and symptoms, these algorithms can suggest adjustments to treatment plans in real-time. This means healthcare providers can make informed decisions quickly, adapting therapies to meet the evolving needs of their patients. It's a dynamic process that aims to improve the effectiveness of treatments and enhance patient recovery.
Minimizing Adverse Reactions
One of the significant benefits of using machine learning in medicine is its ability to predict and minimize adverse drug reactions. By analyzing a wide range of data, including genetic information and clinical records, machine learning models can identify potential risks before they become a problem. This proactive approach helps in selecting safer medications and dosages for patients, thereby reducing the likelihood of negative side effects. It's a step forward in ensuring patient safety and improving overall treatment success.
Machine learning is revolutionizing personalized medicine by providing insights that were previously unattainable. By harnessing the power of data, healthcare providers can now offer treatments that are not only more effective but also safer for each individual.
Machine Learning and the Evolution of Medical Education
Integrating AI into Curriculums
Incorporating AI into medical curriculums is no longer optional—it's becoming a necessity. Harvard Medical School is leading this charge by integrating artificial intelligence into its curriculum. This move aims to prepare future doctors for a world where AI is as common as stethoscopes. AI tools help students understand complex data and improve diagnostic skills. Schools are now using AI to simulate patient interactions, providing a risk-free environment for students to learn and make mistakes.
Preparing Future Healthcare Professionals
Training future healthcare professionals involves more than just teaching them about diseases and treatments. It's about preparing them for a tech-driven future. AI and machine learning are transforming medicine, offering new ways to diagnose and treat patients. Innovations like GPT-4 are at the forefront of this transformation, promising to revolutionize how doctors approach patient care. Students must learn to work alongside these technologies, understanding their strengths and limitations.
Continuous Learning and Adaptation
The pace at which AI is evolving means that learning is never complete. Medical professionals must commit to continuous education to keep up with new technologies. Generative AI is influencing education and healthcare practices, making it crucial for professionals to stay updated. This continuous learning ensures that healthcare providers can offer the best care by leveraging the latest advancements in AI and machine learning.
In the dynamic landscape of healthcare, the integration of AI in medical education is not just about learning new tools—it's about reshaping the very essence of how we train future doctors. It's about fostering a mindset that embraces change and innovation, ensuring that the next generation of healthcare professionals is ready to tackle the challenges of tomorrow.
Conclusion
Machine learning is shaking up the medical world in ways we couldn't have imagined a few years back. It's like having a super-smart assistant that never sleeps, always ready to help doctors make better decisions. From spotting diseases early to crafting personalized treatment plans, machine learning is making healthcare more precise and efficient. But it's not all smooth sailing. There are bumps in the road, like ensuring data privacy and tackling biases in algorithms. Still, the potential benefits are huge, and as technology keeps advancing, the future of patient care looks promising. It's a brave new world for medicine, and machine learning is leading the charge.
Frequently Asked Questions
What is machine learning in medicine?
Machine learning in medicine uses computers to learn from data and help doctors make better decisions. It can look at lots of information quickly to find patterns that help in diagnosing diseases or choosing treatments.
How does machine learning improve patient care?
Machine learning helps doctors by providing accurate diagnoses, predicting health issues, and suggesting personalized treatments. This means patients get better care that's tailored to their needs.
Can machine learning help with early disease detection?
Yes, machine learning can find early signs of diseases by analyzing medical records and test results. This helps doctors catch illnesses sooner and start treatment earlier.
What role does machine learning play in medical imaging?
Machine learning can analyze medical images like X-rays or MRIs to spot problems that might be hard for human eyes to see, making diagnoses faster and more accurate.
Are there ethical concerns with machine learning in healthcare?
Yes, there are concerns about privacy, data security, and bias in machine learning systems. It's important to ensure that these technologies are fair and protect patient information.
How does machine learning help in drug discovery?
Machine learning speeds up drug discovery by analyzing data to find new drug candidates and predict how effective they might be, making the process faster and less costly.
What challenges does machine learning face in medicine?
Challenges include ensuring data quality, integrating new technologies with existing systems, and addressing ethical issues like bias and privacy.
What is the future of machine learning in healthcare?
The future includes more personalized medicine, better disease prediction, and improved healthcare delivery through smarter systems that work alongside healthcare professionals.