Revolutionizing Molecular Prediction: MIT's Breakthrough in Computational Chemistry
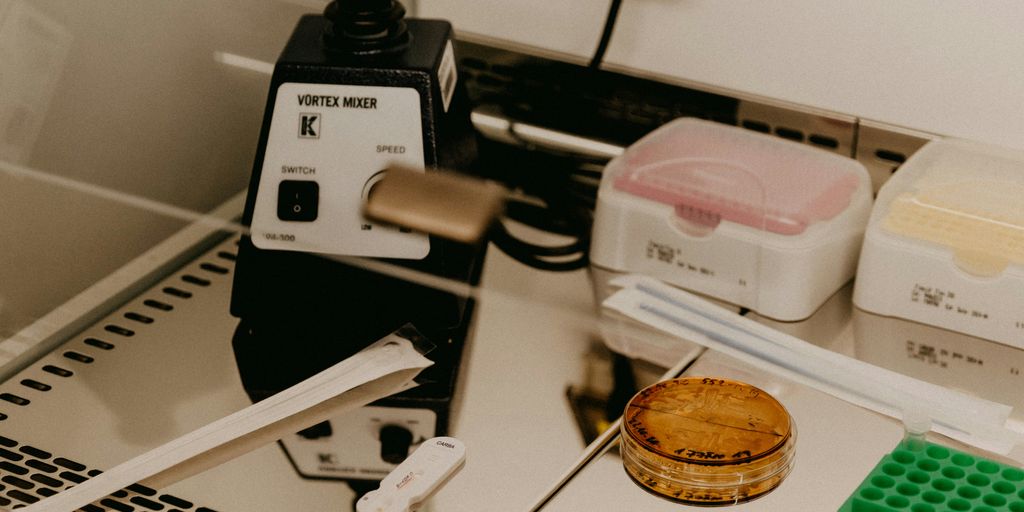
Recent advancements in computational chemistry at MIT have introduced innovative techniques that significantly enhance the prediction of molecules and materials. Led by Professor Ju Li, this research promises to transform materials design by leveraging machine learning and quantum mechanics, paving the way for new discoveries in various scientific fields.
Key Takeaways
- MIT researchers have developed a novel neural network architecture to improve molecular predictions.
- The new method combines coupled-cluster theory with machine learning for enhanced accuracy.
- This approach allows for the analysis of larger molecules, potentially revolutionizing materials science.
The Evolution of Materials Science
Historically, the quest for material design has been a challenging endeavor, with early scientists engaging in alchemical experiments. However, the advent of the periodic table and modern computational methods has drastically changed the landscape of materials science. In recent years, machine learning has emerged as a powerful tool, enabling researchers to better understand molecular structures and properties.
The Breakthrough Technique
The research team at MIT has shifted from traditional density functional theory (DFT) to a more sophisticated method known as coupled-cluster theory (CCSD(T)). This technique is recognized as the gold standard in quantum chemistry, providing highly accurate results. However, its computational demands have limited its application to small molecules.
To overcome these limitations, the team utilized machine learning to train a neural network based on initial CCSD(T) calculations. This innovative approach allows the neural network to perform rapid calculations while extracting a wealth of information about molecular properties, including:
- Dipole and quadrupole moments
- Electronic polarizability
- Optical excitation gaps
Multi-Task Learning Approach
The newly developed model, termed the Multi-task Electronic Hamiltonian network (MEHnet), stands out for its ability to evaluate multiple electronic properties simultaneously. This multi-task approach simplifies the analysis process, as it eliminates the need for separate models for different properties.
Testing and Validation
In tests involving known hydrocarbon molecules, the MEHnet model demonstrated superior performance compared to traditional DFT methods, closely aligning with experimental data. This validation underscores the model's potential for broader applications in materials science.
Future Implications
The implications of this research are vast. By enabling the analysis of larger molecules, the MEHnet model could facilitate the discovery of new materials for various applications, including:
- Drug design
- Semiconductor devices
- Advanced battery technologies
As the researchers continue to refine their model, they aim to extend its capabilities to analyze thousands of atoms, potentially revolutionizing the field of materials science.
Conclusion
The integration of machine learning with quantum chemistry represents a significant leap forward in the prediction of molecular properties. As this research progresses, it holds the promise of unlocking new avenues for material innovation, ultimately impacting numerous industries and scientific disciplines.