How Quantum Computing Could Supercharge AI
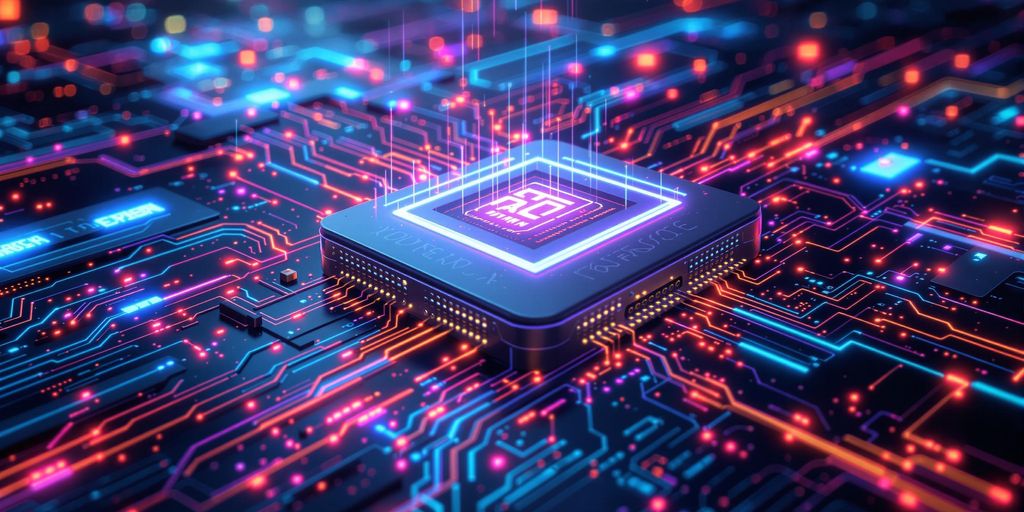
Quantum AI is a fascinating blend of two cutting-edge fields: quantum computing and artificial intelligence. While still in its early days, this combination promises to tackle some of the biggest challenges in AI, from solving complex optimization problems to processing massive datasets. Imagine a world where AI systems can learn and adapt faster than ever before, all thanks to the unique powers of quantum physics. This article explores how quantum computing could revolutionize AI, opening doors to possibilities we’re just beginning to understand.
Key Takeaways
- Quantum AI could significantly speed up data processing and optimization tasks, making AI systems more efficient.
- Quantum neural networks may provide new ways to model and understand complex data.
- Hybrid quantum-classical systems offer a practical approach to combining the strengths of both technologies.
- Quantum algorithms could lead to more energy-efficient AI solutions, reducing computational demands.
- The integration of quantum computing in AI could enhance security and pave the way for groundbreaking applications in fields like cryptography and drug discovery.
The Role of Quantum Speedup in AI Advancements
Understanding Quantum Parallelism
Quantum computers have a knack for doing many things at once, thanks to the principles of superposition and entanglement. Unlike classical computers, which process tasks sequentially, quantum systems can juggle multiple calculations simultaneously. This ability to work in parallel could revolutionize AI by drastically speeding up complex computations. For instance, training AI models that currently take days or weeks might one day be completed in mere hours.
Applications in Large-Scale Data Processing
Handling massive datasets is a cornerstone of AI, and quantum computing is uniquely suited for this challenge. Its parallel processing capabilities allow it to sift through and analyze large volumes of data much faster than traditional systems. Imagine AI applications in areas like climate modeling or genomics, where the datasets are enormous—quantum computing could make these tasks not only feasible but also efficient.
- Faster data categorization and filtering
- Enhanced pattern recognition in unstructured data
- Real-time processing for dynamic datasets
Impact on Optimization Algorithms
Optimization problems are everywhere in AI, from fine-tuning neural networks to improving supply chain logistics. Quantum algorithms, like the Quantum Approximate Optimization Algorithm (QAOA), offer a new way to tackle these challenges. By finding better solutions faster, these algorithms could lead to AI systems that are not just quicker but also smarter.
Quantum speedup isn't just about doing the same tasks faster; it's about enabling entirely new possibilities in AI.
Quantum Neural Networks: A New Frontier in AI
Combining Quantum Mechanics with Neural Architectures
Quantum Neural Networks (QNNs) aim to merge the principles of quantum computing with the structure of traditional neural networks. Unlike classical neural networks, which rely on binary computation, QNNs utilize quantum bits (qubits) that can represent multiple states simultaneously. This allows for parallel processing of data, making them potentially faster and more efficient in handling complex computations. The ability to process and analyze massive datasets simultaneously could revolutionize AI applications like natural language processing and image recognition.
Potential for Enhanced Data Representation
QNNs introduce new ways to model and represent data. With quantum superposition and entanglement, these networks can encode relationships and patterns in data that classical systems might miss. For tasks like pattern recognition or predictive modeling, this could mean more accurate and nuanced outcomes. Some expected benefits include:
- Improved accuracy in AI models due to richer data encoding.
- Faster convergence during training, reducing computational time.
- Handling of high-dimensional data with greater ease.
Challenges in Developing Quantum Neural Networks
Developing QNNs isn’t without its hurdles. Quantum hardware is still in its infancy, with issues like error rates and qubit stability posing significant challenges. Additionally, creating algorithms that fully exploit quantum mechanics while integrating seamlessly with classical systems is a complex task. Key challenges include:
- Limited availability of scalable quantum hardware.
- High costs associated with quantum computing resources.
- The need for specialized expertise to design and implement QNNs.
The journey to fully functional QNNs is a marathon, not a sprint. While the potential is enormous, overcoming these challenges will require collaboration across disciplines and years of dedicated research.
Hybrid Quantum-Classical Systems for AI
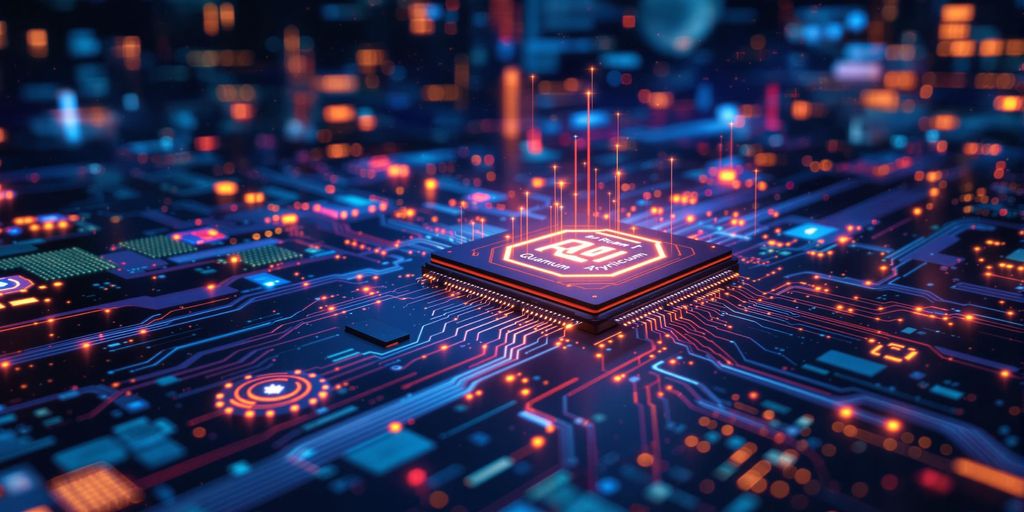
How Hybrid Models Work
Hybrid quantum-classical systems combine the best of both worlds: the unique capabilities of quantum processors and the reliability of classical computing. Quantum processors are used for tasks that benefit from quantum mechanics, such as solving complex optimization problems or simulating quantum systems. Meanwhile, classical processors handle the bulk of standard computations, keeping the system efficient and scalable. This division of labor ensures that each type of processor focuses on tasks it handles best, making the overall system more effective.
Advantages of Combining Quantum and Classical Computing
Here are some key benefits of hybrid systems:
- Scalability: By offloading specific tasks to quantum processors, classical systems can handle larger workloads without becoming overwhelmed.
- Cost-Effectiveness: Quantum hardware is expensive and not yet widely available. Hybrid systems reduce costs by using classical processors for most tasks.
- Flexibility: These systems can adapt to different types of problems, switching between quantum and classical resources as needed.
Feature | Quantum Processors | Classical Processors |
---|---|---|
Best for | Quantum mechanics-based tasks | Standard computations |
Speed | Faster for specific problems | Consistent and reliable |
Availability | Limited | Widely accessible |
Real-World Applications of Hybrid Systems
Hybrid quantum-classical systems are already making waves in several fields:
- Drug Discovery: Accelerating the identification of potential drug compounds by simulating molecular interactions.
- Financial Modeling: Optimizing portfolios and analyzing complex financial systems more efficiently.
- Logistics: Solving challenging routing and scheduling problems, like supply chain management.
Hybrid systems are a stepping stone toward fully quantum-powered AI, bridging the gap between today’s technology and the quantum future.
For more on how hybrid quantum-classical algorithms are implemented, explore their role in enhancing computational efficiency and tackling complex problems.
Energy Efficiency in Quantum AI Systems
Reducing Computational Steps with Quantum Algorithms
Quantum computers have this knack for tackling problems with fewer steps compared to their classical counterparts. Fewer steps mean less energy used, which is a big deal when you’re running energy-hungry AI models. For example, tasks like optimizing large datasets or training neural networks, which usually eat up tons of power, could see massive energy savings thanks to quantum algorithms. It’s not just about being faster—it’s about doing more with less.
Implications for Sustainable AI
As AI keeps growing—think bigger datasets, more complex models—the energy demands are skyrocketing. Quantum computing could be a game-changer here. By slashing the number of operations needed, quantum systems could pave the way for AI that’s not just powerful but also sustainable. Imagine companies hitting their sustainability targets while still pushing the boundaries of AI. That’s the kind of future quantum could help build.
Energy Savings in High-Demand Applications
High-performance computing is notorious for guzzling energy, especially in areas like weather modeling, financial forecasting, or even training advanced AI like GPT models. Quantum computing can step in here. By handling these intensive tasks more efficiently, it could cut down energy use significantly. For instance, quantum computers might only need a fraction of the physical resources to achieve the same or even better outcomes, making them a solid option for energy-conscious industries.
The promise of quantum AI isn’t just about speed or power—it’s about rethinking how we use energy in computing. As the tech evolves, it could reshape what’s possible in AI while keeping the planet in mind.
Quantum AI in Security and Cryptography
Quantum-Resistant Cryptographic Techniques
As quantum computers advance, they pose a significant threat to traditional encryption methods. Algorithms like RSA and ECC, which are widely used today, could be easily broken by quantum capabilities. To counter this, researchers are developing quantum-resistant cryptographic techniques. These methods aim to safeguard sensitive data by creating encryption protocols that even quantum computers can’t crack. This shift is crucial for maintaining the integrity of digital communication and data storage in the quantum era.
Enhancing AI Security with Quantum Computing
Quantum AI systems can bolster cybersecurity by analyzing massive amounts of data in real-time. This capability enables the detection of subtle patterns and anomalies that might indicate cyber threats. For instance, Quantum AI systems enhance cybersecurity by identifying potential vulnerabilities and mitigating risks faster than traditional methods. This combination of AI and quantum computing ensures more robust defenses against increasingly sophisticated cyber attacks.
Addressing Threats to Current Encryption Methods
The rise of quantum computing also forces us to rethink existing encryption. While quantum-resistant algorithms are being developed, there’s an urgent need to transition away from vulnerable systems. Steps include:
- Evaluating current encryption protocols for quantum vulnerability.
- Implementing hybrid cryptographic systems that combine classical and quantum-safe methods.
- Educating organizations about the timeline and risks associated with quantum decryption capabilities.
The transition to quantum-safe encryption isn’t just a technical challenge—it’s a race against time to secure global digital infrastructure before quantum computers become mainstream.
Simulating Complex Systems with Quantum AI
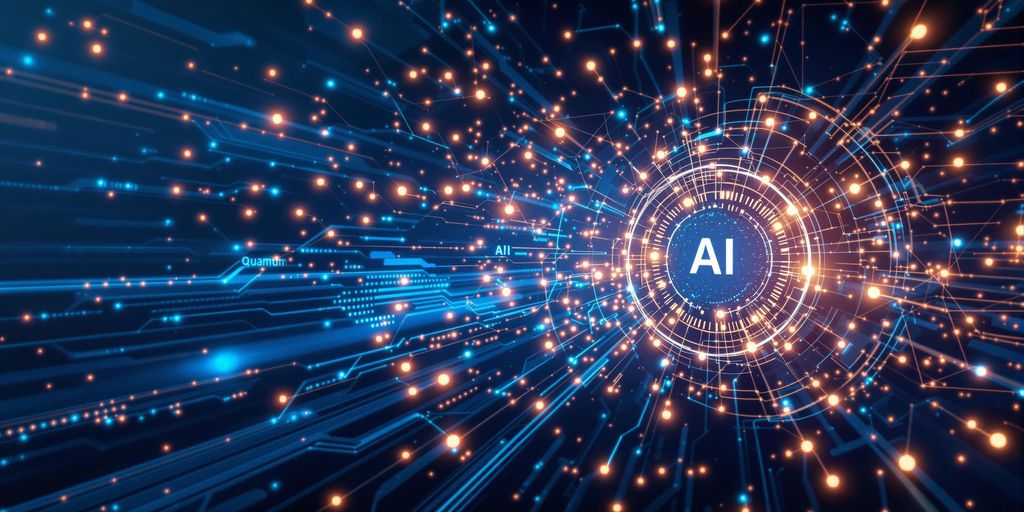
Applications in Quantum Chemistry and Drug Discovery
Quantum computers are redefining the way we approach molecular simulations. These machines can mimic the behavior of quantum systems, something traditional computers struggle with. This opens doors to breakthroughs in quantum chemistry and drug discovery that were previously unimaginable.
- Predict molecular interactions with unprecedented accuracy.
- Accelerate the design of new drugs by simulating their effects at the molecular level.
- Solve chemical equations that are too complex for classical systems.
For example, researchers are already using quantum simulations to explore how proteins fold, a key step in understanding diseases and creating targeted therapies.
Advancements in Materials Science
Materials science is another field set to benefit massively from quantum AI. By simulating atomic structures and interactions, quantum computers can:
- Identify new materials with unique properties, such as superconductors.
- Optimize existing materials for energy efficiency or durability.
- Predict how materials will behave under extreme conditions.
These advancements could lead to innovations in everything from renewable energy technologies to aerospace engineering.
AI-Driven Insights from Quantum Simulations
When you combine AI with quantum simulations, the possibilities expand even further. AI algorithms can analyze the vast amounts of data generated by quantum computers, uncovering patterns and insights that humans might miss. This synergy enables:
- Faster identification of optimal solutions.
- Improved predictive models for complex systems.
- Enhanced decision-making in fields ranging from climate modeling to financial forecasting.
Quantum simulation isn’t just about solving today’s problems; it’s about unlocking solutions we haven’t even imagined yet. The collaboration between AI and quantum computing is poised to reshape how we understand and interact with the natural world.
By leveraging quantum simulation, researchers are pushing the boundaries of what’s scientifically possible, paving the way for discoveries that could transform industries and improve lives.
The Path to Artificial Superintelligence with Quantum AI
Role of Quantum Computing in Achieving Superintelligence
Quantum computing is poised to be a game-changer in the quest for artificial superintelligence (ASI). Unlike artificial general intelligence (AGI), which aims to match human cognitive abilities, ASI seeks to surpass them entirely. Quantum computers, with their unparalleled processing power, could accelerate this journey by solving problems classical systems can't even approach. For instance, they can handle vast amounts of data simultaneously, making them ideal for training highly complex AI models that require extraordinary computational resources.
Some areas where quantum computing could play a pivotal role include:
- Advanced Decision-Making Models: Quantum algorithms can process probabilities in ways classical systems can't, enabling AI to make more nuanced decisions.
- Real-Time Learning: With quantum speedups, AI systems could adapt and learn from new data almost instantaneously.
- Simulating Human-Like Thought Processes: Quantum systems could mimic the complexity of human neural networks more effectively, pushing the boundaries of machine cognition.
Ethical Considerations and Guardrails
As we edge closer to ASI, ethical concerns become unavoidable. How do we ensure that superintelligent AI aligns with human values? What safeguards can prevent misuse? These questions are more pressing than ever.
Key areas to address include:
- Accountability: Establishing clear systems to hold AI developers and users responsible for outcomes.
- Transparency: Ensuring that quantum-enhanced AI systems operate in a way that is understandable and explainable.
- Global Cooperation: Crafting international regulations to manage the risks and benefits of ASI fairly.
The journey to ASI isn't just a technological challenge—it's a societal one. Without robust ethical frameworks, the risks could outweigh the benefits.
Future Prospects for Quantum-Enhanced AI
Looking ahead, the fusion of quantum computing and AI could unlock possibilities we can't yet imagine. Researchers are already exploring hybrid systems that combine quantum and classical computing to leverage the best of both worlds. These systems could pave the way for:
- Breakthroughs in Medicine: From drug discovery to personalized treatment plans.
- Revolutionized Industries: From finance to logistics, quantum AI could optimize processes at scales previously thought impossible.
- New Forms of Creativity: AI systems could generate art, music, and literature that challenge our understanding of creativity itself.
The road to ASI is long and uncertain, but one thing is clear: quantum computing will be a cornerstone of this journey.
Conclusion
Quantum computing and AI are like two puzzle pieces that could fit together to create something extraordinary. While quantum computing is still in its early days, the potential it holds for transforming AI is hard to ignore. From speeding up data processing to solving problems that seem impossible today, the possibilities are endless. Sure, there are challenges—like making quantum systems more stable and accessible—but the progress being made is exciting. As these technologies evolve, they could open doors to innovations we can’t even imagine yet. It’s not a question of if quantum computing will impact AI, but when and how much. The future is looking pretty interesting, don’t you think?
Frequently Asked Questions
What is quantum computing?
Quantum computing is a new type of computing that uses the principles of quantum mechanics, like superposition and entanglement, to process information. Unlike regular computers, which use bits, quantum computers use qubits that can represent multiple states at once.
How can quantum computing improve artificial intelligence?
Quantum computing can speed up AI tasks by processing large datasets and solving complex problems faster. It can also enhance optimization and data analysis, making AI systems more efficient.
What are quantum neural networks?
Quantum neural networks combine quantum computing with neural network designs. They aim to process and represent data in new ways, potentially leading to smarter and more adaptable AI systems.
Why are hybrid quantum-classical systems important?
Hybrid systems use both quantum and classical computing to tackle tasks. Quantum processors handle specialized problems, while classical computers manage the rest, making the system more practical and scalable.
Can quantum computing make AI more energy-efficient?
Yes, quantum computing can solve problems with fewer steps, reducing energy use. This is especially helpful for AI tasks that need a lot of computational power.
Is quantum computing secure for AI applications?
Quantum computing can both pose risks and improve security. It can break traditional encryption but also create quantum-resistant methods to protect AI systems and sensitive data.