Exploring the Impact of Machine Learning in Medicine: A Comprehensive PDF Guide
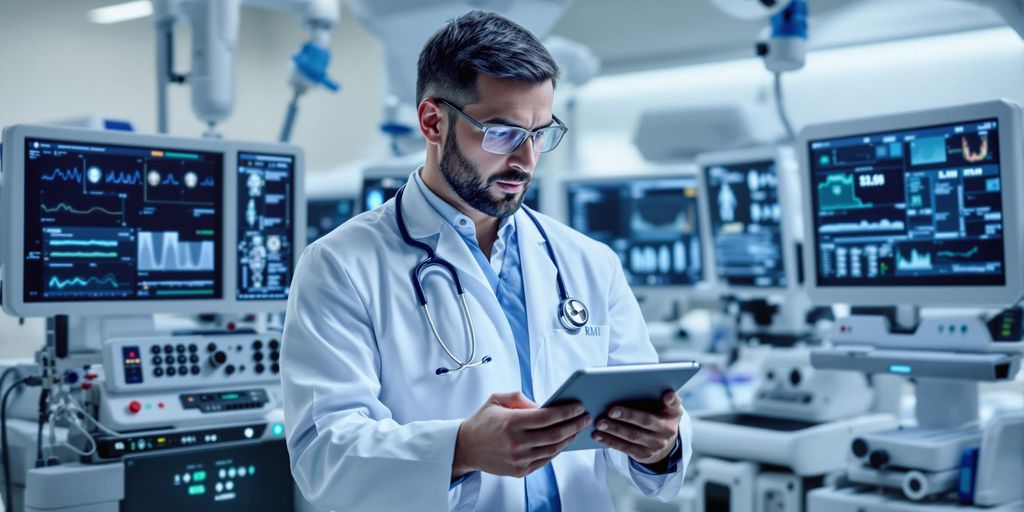
Machine learning is changing the way we look at medicine. From diagnosing diseases to finding new drugs, it's making a big difference. This guide dives into how machine learning is being used in medicine today and what it could mean for the future. Whether you're a doctor, a patient, or just curious, there's something here for you. Let's explore the world of machine learning in medicine together.
Key Takeaways
- Machine learning is revolutionizing medical diagnostics and treatments.
- Ethical considerations are crucial when integrating AI into healthcare.
- Personalized medicine is becoming more achievable with machine learning.
- AI is speeding up drug discovery and development processes.
- The future of telemedicine is being shaped by machine learning technologies.
The Evolution of Machine Learning in Medicine
Historical Milestones in Medical AI
Machine learning in medicine has come a long way since its inception. Back in the 1950s and 60s, the idea of using computers for medical diagnosis was just taking shape. Fast forward to the early 2000s, and we saw the development of advanced deep learning models that really kicked things into high gear. These models allowed for more accurate predictions and diagnoses, transforming how doctors approached patient care.
Key Innovations in Machine Learning
The journey of machine learning in healthcare is marked by several key innovations. From natural language processing to image recognition, these technologies have reshaped medicine in ways we couldn't have imagined a few decades ago. For instance, AI-powered tools can now analyze medical images with incredible precision, often spotting anomalies that human eyes might miss. This has not only improved diagnostic accuracy but also sped up the process significantly.
Impact on Modern Medical Practices
Today, machine learning is not just an add-on; it's a core component of modern medical practice. Whether it's enhancing data handling or personalizing patient care, AI systems are integral to the healthcare ecosystem. They help doctors make more informed decisions by providing insights that were previously inaccessible. As these technologies continue to evolve, their role in medicine will only grow, offering new ways to improve patient outcomes and streamline operations.
Transforming Diagnostics with Machine Learning
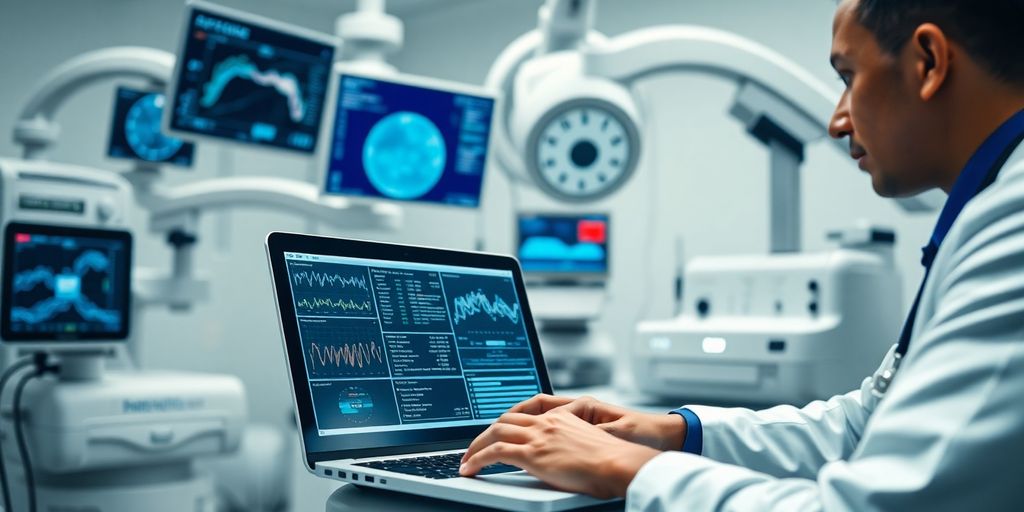
Enhancing Accuracy in Disease Detection
Machine learning is changing how we spot diseases by using algorithms that can look at medical images and data in ways humans can't. These algorithms can identify patterns that might go unnoticed by the human eye, leading to earlier and more accurate diagnoses. This means doctors can catch diseases earlier, which can make treatments more effective. For example, in radiology, machine learning can help identify tumors in scans more accurately than traditional methods.
Predictive Analytics in Patient Care
Predictive analytics uses data to foresee potential health issues before they become real problems. By analyzing a patient's history and current data, machine learning models can predict the likelihood of diseases like diabetes or heart disease. This helps doctors make informed decisions about prevention and treatment plans. For instance, predictive models can alert doctors to patients who might be at risk of a heart attack, allowing for preventative measures to be taken.
Case Studies of Successful Implementations
There are tons of examples where machine learning has made a big difference in diagnostics. In one case, a hospital used machine learning to predict sepsis in patients, reducing mortality rates significantly. Another example is the use of AI to analyze retinal images for signs of diabetic retinopathy, which has improved early detection rates. These case studies show how powerful machine learning can be in making healthcare more effective and efficient.
Machine learning isn't just a tool for doctors; it's a partner in improving patient outcomes. As technology advances, its role in healthcare will only grow, offering new ways to diagnose and treat patients more effectively.
Machine Learning in Personalized Medicine
Tailoring Treatments to Individual Patients
Machine learning is reshaping how we approach personalized medicine by analyzing extensive datasets to tailor treatments to each individual. By examining genetic information, lifestyle factors, and medical history, algorithms can predict the most effective therapies for patients. This shift means treatments are no longer one-size-fits-all but are customized to fit the unique needs of each person.
- Data Analysis: Machine learning processes vast amounts of data, identifying patterns that human experts might miss.
- Predictive Models: These models can forecast how patients will respond to different treatments, allowing for more precise interventions.
- Adaptive Therapies: As new data is collected, treatments can be adjusted in real-time to improve outcomes.
Genomic Data and Machine Learning
The integration of genomic data into machine learning models is a game-changer for medicine. By understanding the genetic basis of diseases, we can develop targeted therapies that address the root cause rather than just symptoms. For instance, in cancer treatment, machine learning can help identify mutations and suggest drugs that specifically target those changes.
Genomic Data Use | Machine Learning Contribution |
---|---|
Identifying mutations | Algorithms pinpoint specific genetic alterations linked to diseases |
Drug matching | Suggests drugs based on genetic profiles |
Risk assessment | Evaluates genetic risk factors for preventive measures |
Challenges in Personalization
While the potential of personalized medicine is vast, several challenges need addressing:
- Data Privacy: Ensuring patient data is protected while being used for machine learning is crucial.
- Bias in Algorithms: There's a risk of bias in data, which can lead to unequal treatment recommendations.
- Integration into Healthcare: Incorporating these technologies into everyday healthcare settings requires significant changes in infrastructure and training.
"Machine learning in personalized medicine offers incredible promise, but it's essential to navigate the complexities of data privacy and algorithmic fairness to truly transform healthcare."
Ethical Considerations in Medical AI
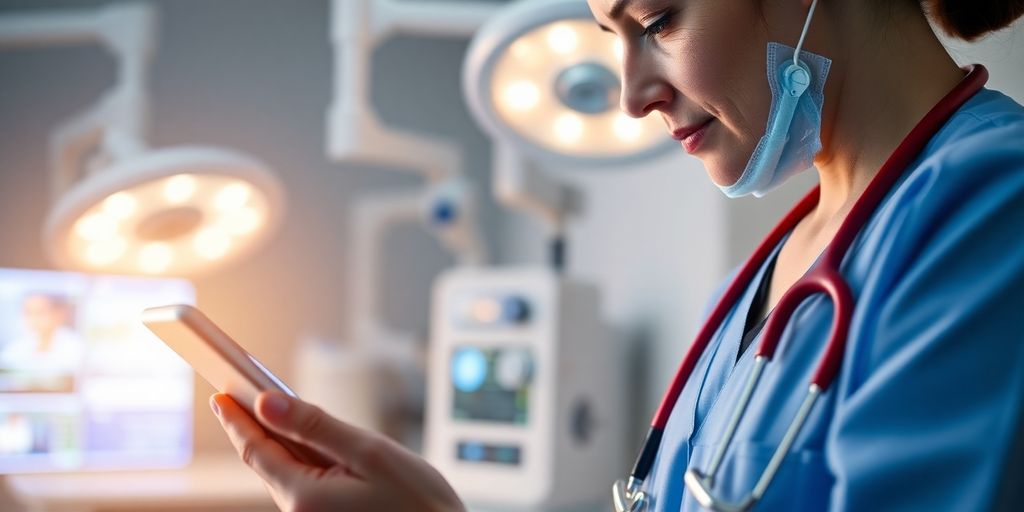
Balancing Innovation with Patient Privacy
In the world of healthcare, artificial intelligence (AI) is a game-changer. But with great power comes great responsibility. Protecting patient privacy is not just a legal obligation but a moral one. As AI systems handle more sensitive data, ensuring that personal information remains confidential becomes a top priority. This involves robust encryption methods, strict access controls, and regular audits to prevent unauthorized access. Integrating AI in healthcare requires a balanced approach that respects patient autonomy and maintains trust.
Addressing Bias in Machine Learning Models
AI models, like humans, can be flawed. When trained on biased data, they can perpetuate existing disparities in healthcare. This is a big deal because it can lead to unequal treatment outcomes. Tackling this issue requires a multi-step approach:
- Diverse Data Collection: Ensure datasets are representative of all patient demographics.
- Bias Detection Tools: Implement tools to identify and correct biases in AI algorithms.
- Continuous Monitoring: Regularly update and assess AI systems to mitigate bias.
By addressing bias in AI, we can strive for fairer and more accurate healthcare solutions.
Regulatory Frameworks and Compliance
The rapid evolution of AI in medicine calls for equally swift updates in regulatory frameworks. Current laws often lag behind technological advancements, leaving gaps in oversight. To bridge these gaps, a comprehensive regulatory framework is essential. This framework should:
- Clearly define accountability for AI-driven decisions.
- Set standards for AI system validation and testing.
- Ensure transparency in AI operations and decision-making processes.
Developing such frameworks is crucial to balancing innovation with responsibility and ensuring AI systems are safe and effective.
AI in healthcare is like a double-edged sword. It offers immense potential to improve patient care but also poses significant ethical challenges that need careful consideration.
Machine Learning in Medical Imaging
Advancements in Radiology and Imaging
The world of medical imaging has seen a significant shift with the introduction of machine learning. In radiology, algorithms now assist in interpreting complex images faster than ever before. This not only speeds up diagnosis but also reduces the workload on radiologists. The use of deep learning techniques allows for the detection of minute details in scans, which might be overlooked by the human eye. This is particularly beneficial in identifying early-stage diseases, where early intervention can be life-saving.
Automating Image Analysis
Automation in image analysis is a game-changer. Machine learning models, trained on thousands of images, can now analyze new scans with remarkable accuracy. This reduces the chance of human error and ensures consistency in results. Moreover, automation allows for the processing of large volumes of data, which is crucial in hospitals with high patient turnover. With these systems in place, radiologists can focus more on complex cases that require human judgment.
Improving Diagnostic Speed and Accuracy
Speed and accuracy are critical in medical diagnostics. Machine learning enhances both by providing tools that can quickly process and analyze imaging data. This technology can prioritize urgent cases, ensuring that patients receive timely care. Furthermore, as these systems learn and improve, they continue to enhance their accuracy, providing even more reliable diagnostics over time. The potential for machine learning to transform patient outcomes is immense, particularly in emergency settings where every second counts.
"The integration of machine learning into medical imaging is not just about faster diagnosis; it's about making healthcare more accessible and efficient for everyone."
The future of machine learning in medical imaging is bright, with continuous advancements promising to further revolutionize how we diagnose and treat diseases.
The Role of Machine Learning in Drug Discovery
Accelerating the Drug Development Process
Machine learning (ML) is like a turbo boost for drug development. It speeds up the whole process by analyzing tons of data that would take humans forever to sift through. With ML, researchers can quickly identify which compounds might work as drugs and which ones won't, saving a lot of time and money. This efficiency is a game-changer, especially when you consider how long and costly traditional drug development can be.
AI-Driven Drug Repurposing
Repurposing existing drugs for new uses is another area where AI shines. By using AI models, scientists can predict how an existing drug might work against different diseases. This approach not only cuts down the time needed to find new treatments but also reduces the risk since these drugs have already been tested for safety. It's like finding a new use for an old tool, making it incredibly efficient.
Challenges in Integrating AI with Pharmacology
Despite all the benefits, integrating AI into pharmacology isn't without its hurdles. One major challenge is ensuring that AI models are accurate and reliable, which is crucial for predicting how drugs will behave in the human body. Another issue is the sheer complexity of biological systems, which can make it difficult for AI to model accurately. Plus, there's always the concern about data privacy and security when dealing with sensitive health information. Overcoming these challenges is key to fully harnessing the potential of AI in drug discovery.
"The integration of machine learning techniques into small-molecule therapeutics development is anticipated to significantly enhance the delivery and efficacy of treatments, as suggested by various studies."
In summary, while AI and ML are transforming the landscape of drug discovery, it's essential to address these challenges to maximize their potential benefits.
Machine Learning and Telemedicine
Enhancing Remote Patient Monitoring
Machine learning is reshaping how doctors keep an eye on patients from afar. With the rise of smart devices and wearables, doctors can now track vital signs in real-time. This tech means fewer hospital visits, saving time and money. Imagine wearing a wristband that checks your heart rate and sends alerts if something's off. For people with chronic conditions, this is a game-changer.
- Continuous monitoring with wearables
- Real-time data analysis
- Alerts for abnormal readings
AI in Virtual Health Consultations
Ever tried a virtual doctor visit? It's like FaceTime, but with your doctor. AI is making these visits smarter by analyzing symptoms and suggesting possible conditions. This doesn't replace doctors but helps them make better decisions. For instance, AI can remind doctors of rare diseases that match the symptoms.
- Symptom analysis
- Decision support for doctors
- Increased accessibility to healthcare
Overcoming Barriers to Telehealth Adoption
While telehealth is great, it's not perfect. Some folks struggle with tech, and not everyone has fast internet. Plus, there's the trust issue—some people just prefer seeing a doctor in person. To fix this, we need better tech support and education. Governments and companies are working on this, making telehealth more user-friendly and reliable.
- Improving internet access
- Tech support for users
- Building trust in telehealth systems
"Telemedicine is not just a trend; it's a necessary evolution in healthcare, especially for those in remote areas."
Machine learning is changing the face of telemedicine, making it more accessible and efficient. From remote monitoring to virtual consultations, AI is playing a crucial role in shaping the future of healthcare.
Machine Learning in Surgery and Robotics
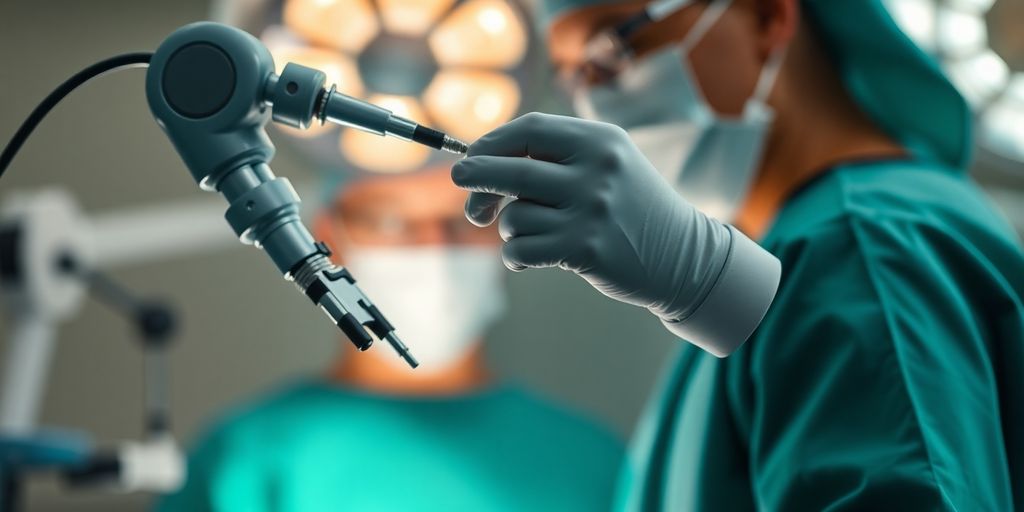
AI-Assisted Surgical Procedures
Artificial intelligence is stepping into the operating room, making waves with its ability to assist in surgical procedures. AI systems can analyze complex surgical data in real-time, offering surgeons valuable insights and suggestions. For instance, a research team has trained a robot to perform basic surgical tasks using videos of surgeries, leveraging machine learning architectures similar to ChatGPT. This approach is reshaping how surgeries are performed, providing a new layer of precision and support to human surgeons.
Robotic Surgery Innovations
The integration of machine learning with robotic systems is leading to groundbreaking innovations in surgery. Imitation learning, for example, allows robots to learn surgical tasks by watching videos, rather than requiring manual programming for each movement. This advancement streamlines the training process and boosts the efficiency of robotic surgery. Furthermore, reinforcement learning enables robots to master intricate procedures, such as suturing and tissue manipulation, enhancing their capabilities in the surgical field.
Training Surgeons with AI Technologies
AI technologies are not just transforming surgeries but also the way surgeons are trained. With the help of AI, surgical training is becoming more interactive and personalized. Virtual reality simulations powered by AI provide a safe environment for surgeons to practice and hone their skills without the risks associated with real-life surgeries. AI's potential to enhance automation in robotic surgery is also easing the workload for physicians, allowing them to focus more on patient care and less on repetitive tasks.
The future of surgery is not just human or robotic; it's a harmonious blend of both, where AI acts as a catalyst for precision and efficiency.
Future Trends in Machine Learning for Medicine
Emerging Technologies and Innovations
Machine learning is set to revolutionize healthcare with innovations that were once the stuff of science fiction. From advanced algorithms that crunch massive datasets to uncover patterns in patient outcomes, to AI-driven tools that predict disease outbreaks, the future is bright. AI's ability to process and analyze unstructured data is a game-changer in early disease detection, as noted by Dr. Russell Hunter in his insights on healthcare trends. This isn't just about cutting-edge tech; it's about transforming patient care.
The Future of AI in Healthcare
AI is not here to replace doctors but to make their jobs easier. Imagine a world where administrative tasks are handled by AI, freeing up doctors to focus more on patients. According to an article on AI's role in healthcare, this shift is already underway, with AI assisting in diagnosis and treatment planning. The goal is to enhance, not replace, the human touch in medicine.
Preparing the Medical Workforce for AI
As AI becomes more integrated into healthcare, there's a pressing need for medical professionals to get up to speed. Training programs are evolving to include AI literacy, ensuring that future doctors are not just tech-savvy but also comfortable working alongside AI technologies. This is crucial for identifying and addressing gaps in patient care, as highlighted in a piece on AI and healthcare quality. It's about equipping the workforce with the tools they need to thrive in a tech-driven world.
AI in medicine isn't just a trend—it's a transformation. As these technologies evolve, the focus remains on improving patient outcomes and streamlining healthcare processes. The future is not about AI taking over, but about creating a harmonious balance between technology and the irreplaceable human element in healthcare.
Machine Learning in Public Health
Predicting and Managing Epidemics
Machine learning is reshaping how we predict and manage epidemics. By analyzing vast amounts of data, ML models can identify patterns and predict outbreaks before they happen. During the COVID-19 pandemic, ML was used to predict outbreak hotspots, track the virus, and optimize vaccine distribution. These models help public health officials make informed decisions, potentially saving countless lives.
AI in Health Policy and Planning
Incorporating AI into health policy and planning can lead to more effective and efficient public health strategies. AI tools are being used to analyze health trends and predict future needs, allowing policymakers to allocate resources more effectively. For instance, machine learning strategies are enhancing disease outbreak surveillance, aiding public health leaders in designing better monitoring systems for future outbreaks.
Improving Population Health Outcomes
Machine learning is not just about managing diseases; it's also about improving overall population health. By analyzing patterns in large datasets, ML can identify risk factors and suggest interventions. In South Florida, researchers are leveraging ML and mathematical models to reduce the transmission of mosquito-borne diseases, showcasing how technology can enhance public health. This approach can lead to targeted interventions and better health outcomes for communities.
Machine learning is more than just a tool; it's a partner in public health. Its ability to process and analyze data at unprecedented scales offers insights that were previously unattainable, paving the way for a healthier future.
Integrating Machine Learning into Medical Education
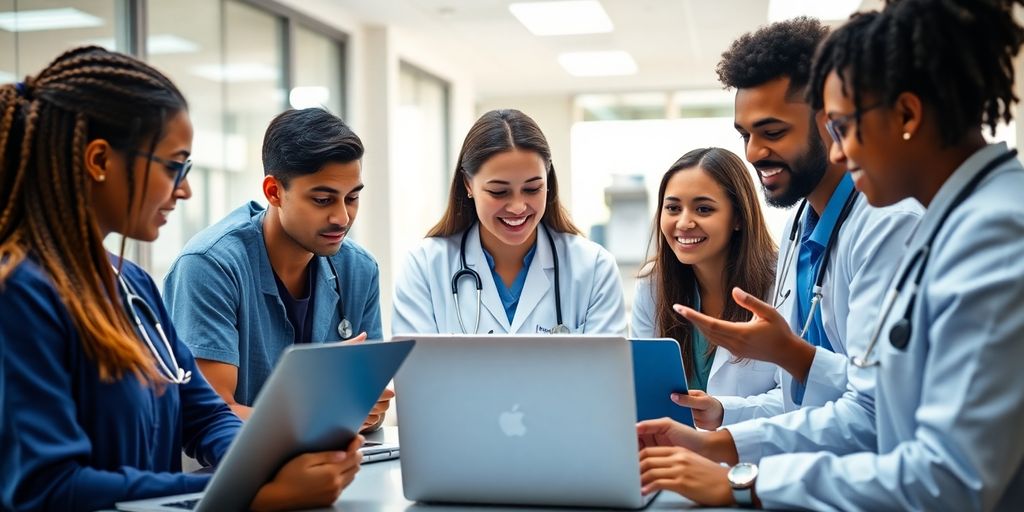
AI Tools for Medical Training
Incorporating AI into medical training isn't just a trend—it's becoming a necessity. With the rapid advancement of technology, medical schools are finding innovative ways to include AI tools in their curriculum. These tools are helping students understand complex topics through interactive simulations and virtual reality. AI-driven platforms can simulate rare medical conditions, providing students with hands-on experience that traditional textbooks can't offer. Schools like Harvard Medical School are already integrating AI into their programs to better prepare students for the future.
Curriculum Development for Future Doctors
Developing a curriculum that includes AI is challenging but essential. Medical educators need to balance traditional teaching methods with new technology. This means integrating AI into subjects like diagnostics, treatment planning, and patient management. Educators must also focus on teaching students about the ethical implications of AI in medicine, ensuring they understand both the potential and the risks. A proposed framework suggests three tiers of AI expertise: basic, advanced, and expert skills, which aim to enhance medical training and improve patient care.
Challenges and Opportunities in AI Education
Integrating AI into medical education presents both challenges and opportunities. One major challenge is keeping the curriculum up to date with the fast-paced changes in AI technology. There's also the question of how to assess students' proficiency in AI tools. However, the opportunities are significant. AI can personalize learning, tailoring educational experiences to individual students' needs. As AI continues to evolve, it will play a crucial role in shaping the future of medical education, offering a more personalized learning experience for students.
"The integration of AI in medical education is not about replacing traditional methods but enhancing them. It's about preparing future doctors to work alongside AI, using it as a tool to improve patient outcomes."
In conclusion, the integration of machine learning into medical education is a complex but necessary evolution. It requires careful planning and execution to ensure that future doctors are equipped with the skills they need to thrive in an AI-driven world.
Conclusion
Machine learning in medicine is like this wild ride we're all on. It's changing things up, making some stuff easier and some stuff, well, a bit more complicated. But hey, that's progress, right? We've seen how it can help doctors make better decisions, speed up diagnoses, and even predict health issues before they become a big deal. But let's not forget, it's not all sunshine and rainbows. There are challenges, like making sure the tech is fair and doesn't mess up. And yeah, there's the whole thing about keeping patient info safe. But overall, it's exciting to think about where this could go. Maybe one day, it'll be as common as a stethoscope in a doctor's office. Until then, we'll keep watching and learning. Who knows what the future holds?
Frequently Asked Questions
What is machine learning in medicine?
Machine learning in medicine is when computers are used to help doctors and healthcare workers understand and predict health problems by looking at lots of data.
How does machine learning improve diagnostics?
Machine learning helps doctors find diseases more accurately by analyzing medical images and patient data to spot patterns that might be missed by humans.
Can machine learning be used in surgery?
Yes, machine learning can assist in surgery by guiding robotic tools and helping surgeons make precise cuts, making surgeries safer and faster.
What are the ethical concerns with AI in medicine?
Ethical concerns include ensuring patient privacy, preventing bias in AI decisions, and making sure AI tools are used safely and fairly.
How does AI personalize medicine?
AI personalizes medicine by analyzing a person's unique genetic information and health data to suggest the best treatment options just for them.
Is AI replacing doctors?
No, AI is not replacing doctors. It is a tool that helps doctors make better decisions and provide better care to patients.
What role does machine learning play in drug discovery?
Machine learning speeds up drug discovery by predicting which drug compounds might work best for a disease, saving time and resources.
How is AI used in telemedicine?
AI in telemedicine helps doctors monitor patients remotely, analyze data from wearable devices, and provide virtual consultations to patients.