Exploring the Future of Multimodal LLM: Innovations and Applications
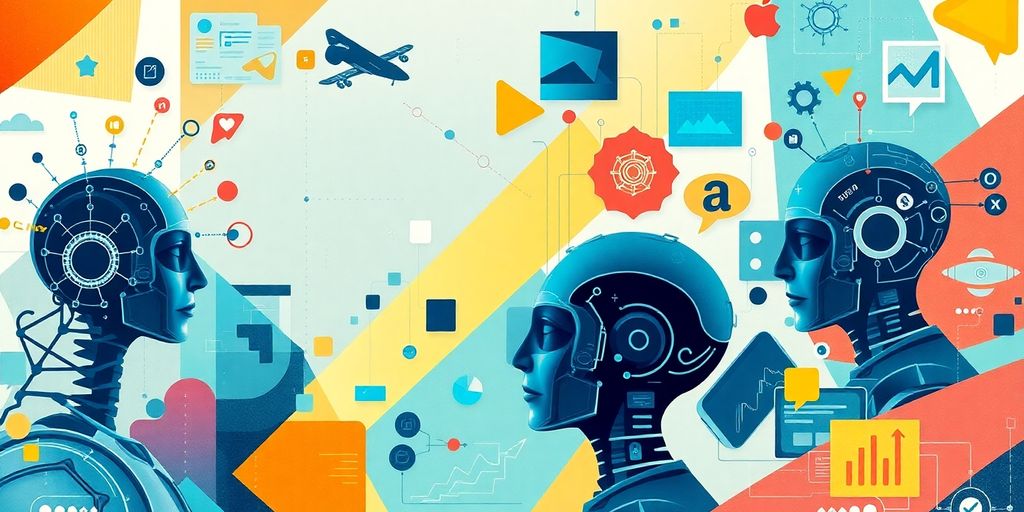
Alright, so here's the deal with multimodal large language models (LLMs). They're like the Swiss Army knives of AI, handling text, images, audio, and video all in one go. It's a big leap from the old days when AI was stuck in one lane, like just text or just images. Now, these models are doing crazy stuff like translating languages while analyzing images at the same time. The tech's growing fast, and it's opening up tons of new ways to use AI in real life. But, of course, it's not all sunshine and rainbows—there are still some bumps in the road.
Key Takeaways
- Multimodal LLMs combine text, images, audio, and video into one model, making them super versatile.
- These models are changing the game in fields like translation, image analysis, and audio processing.
- Despite their potential, integrating different types of data presents challenges, like increased complexity.
- The tech is rapidly evolving, with new applications popping up in various industries.
- There's still work to be done, especially in making these models more efficient and easier to interpret.
Understanding Multimodal LLMs
Defining Multimodal LLMs
Multimodal Large Language Models (MLLMs) are like the Swiss army knives of AI, designed to handle and produce content from various data types like text, images, audio, and video. These models are not just about understanding one kind of data; they can juggle multiple types at once, making them incredibly versatile. Imagine a system that can watch a video, listen to the audio, and read the subtitles all at the same time, then summarize the entire content for you. That's what MLLMs aim to do.
Key Components of Multimodal LLMs
To work their magic, MLLMs rely on three main components:
- Multimodal Input Encoder: This part takes in all sorts of data, whether it's text, images, or sounds, and converts it into a language the model can understand.
- Feature Fusion Mechanism: This is where the real magic happens. It combines the different data types into a single, unified understanding. Think of it like blending fruits to make a smoothie.
- Multimodal Output Decoder: Finally, this component takes the blended data and turns it into a response or action, like generating a descriptive text or a voice command.
Challenges in Multimodal Integration
Despite their potential, MLLMs face several hurdles:
- Aligning Different Modalities: Each data type has its own quirks, and getting them to work together seamlessly is tricky.
- Computational Demands: These models are power-hungry, often needing a lot of computing resources.
- Interpretability: Understanding how these models make decisions is tough, which is a big deal if they're used in critical fields like healthcare.
Multimodal LLMs are paving the way for a new era of AI, where machines can interact with the world in a more human-like manner. But as with any new technology, they come with their own set of challenges and growing pains.
Innovations in Multimodal LLM Technology
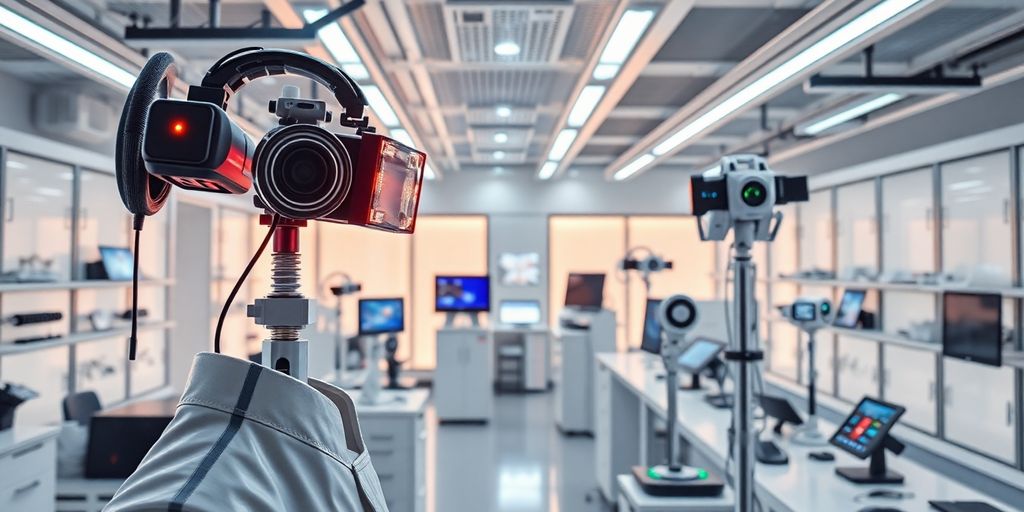
Advancements in Multimodal Fusion
Multimodal fusion is where the magic happens in multimodal LLMs. It’s about taking different types of data—like text, images, and audio—and blending them into one coherent understanding. One common approach is using simple linear layers, but attention mechanisms are gaining ground because they can handle more complex interactions. Of course, this comes with higher computational demands, but the payoff is a richer interaction between data types. This fusion is what makes multimodal systems smarter and more context-aware, a key aspect of multimodal AI that enhances human-technology interaction.
Role of Self-Supervised Learning
Self-supervised learning (SSL) is a big deal in the world of AI. It’s like teaching a model to learn by itself using the data it has, which is super useful for tasks like speech recognition and image analysis. Models like HuBERT and SoundStream have shown that SSL can solve many tricky problems in speech processing. These models use techniques like masked prediction training to improve their understanding of audio and speech. The integration of SSL with multimodal LLMs means these models can now handle speech, text, and images together, opening up new possibilities for applications that require a mix of modalities.
Impact of Large Context Windows
Large context windows are changing the game for LLMs. Imagine being able to process and understand huge amounts of data at once—that’s what these windows allow. They let models like Gemini 2.0, showcased at CES 2025, take in vast amounts of information, making them capable of more complex reasoning and understanding. This is particularly useful in areas like healthcare and education, where understanding large datasets quickly can lead to better outcomes. The ability to handle large context windows makes these models not just smarter but also more efficient, paving the way for new innovations in AI.
Applications of Multimodal LLMs
Enhancing Language Translation
Multimodal Large Language Models (MLLMs) are changing how we approach language translation. By integrating text with visual cues, these models can offer translations that are not only accurate but also contextually rich. Imagine translating a restaurant menu where a picture of the dish is analyzed alongside the text. This dual approach helps in capturing nuances that might be lost in text-only translations. This advancement makes translations more relatable and precise, especially in culturally rich contexts.
Improving Image and Video Analysis
The capability of MLLMs to interpret both images and text simultaneously opens up new avenues in media analysis. For example, these models can analyze a news video by understanding the spoken words, facial expressions, and on-screen text all at once. This comprehensive analysis allows for a deeper understanding of the content, making it useful for applications like automated content moderation or creating detailed summaries of video content. Visual data combined with textual information leads to more accurate and insightful analysis.
Revolutionizing Audio Processing
In the world of audio, MLLMs are breaking new ground by integrating sound with other data types. Whether it's transcribing a podcast while also summarizing its key points or enhancing speech recognition systems by understanding the speaker's emotions through tone and context, these models are making audio processing more sophisticated. They can even assist in creating more interactive and personalized audio experiences, like smart assistants that respond not just to what you say, but how you say it.
Multimodal LLMs are not just about processing different types of data; they're about creating a synergy that enhances the understanding and interaction with information. This is a leap forward in making technology more intuitive and responsive to human needs.
Challenges and Limitations of Multimodal LLMs
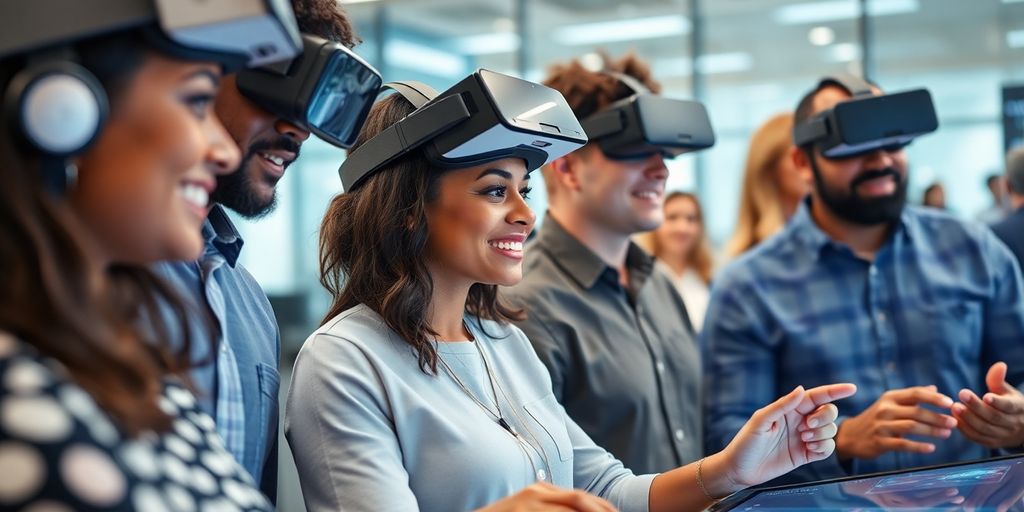
Computational Complexity Issues
Multimodal LLMs are power-hungry beasts. Integrating different types of data—like text, images, and audio—demands a ton of computing power. This high computational need can make these models slow and expensive to run. As the models grow in size and complexity, they require even more resources, which can become a bottleneck for widespread use. Balancing performance and resource use is a tricky task.
Interpretability Concerns
Understanding how multimodal LLMs make decisions is like trying to see through a foggy window. These models often act like a "black box," making it tough to figure out why they make certain decisions. This lack of transparency can be a big problem, especially when these models are used in critical areas like healthcare or finance. Users need to trust these systems, and without clear explanations, that trust is hard to build.
Data Privacy and Security
Handling multiple types of data raises serious privacy and security concerns. With different data types being processed together, the risk of data breaches or misuse increases. Ensuring that sensitive information is protected while still allowing the model to function effectively is a major challenge. Moreover, as these models become more integrated into daily life, the potential for misuse of personal data grows, making robust security measures essential.
Multimodal LLMs offer exciting possibilities but come with their own set of hurdles. Tackling these challenges requires innovation and a careful balance between technological advancement and ethical responsibility.
Future Directions for Multimodal LLMs
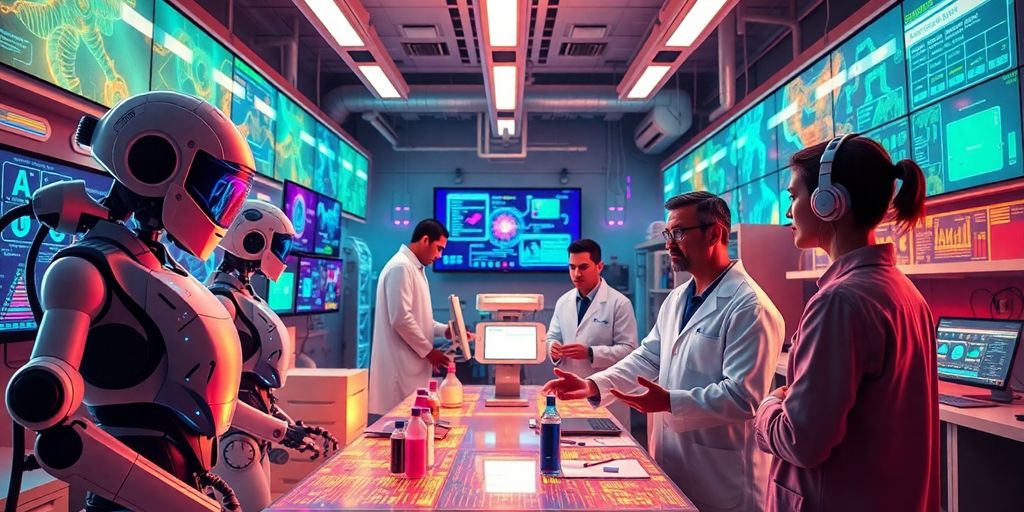
Balancing Generalization and Specialization
The debate rages on: should multimodal LLMs aim to be jack-of-all-trades or masters of specific domains? As these models grow, there's a strong pull towards creating systems that can handle a wide array of tasks. But evidence suggests that more focused models might excel in niche areas. Striking the right balance between broad capabilities and specialized expertise remains a key challenge.
Exploring New Modalities
The future is all about expanding horizons. Multimodal LLMs are already juggling text, images, and audio, but what's next? Imagine integrating data from wearables or even brainwave patterns. This could open up wild new possibilities, from enhancing healthcare diagnostics to driving innovations in autonomous vehicles.
Enhancing Model Efficiency
As these models grow, so does their hunger for computational power. The need to make them leaner and meaner is more pressing than ever. Researchers are exploring ways to cut down on the resources needed without losing performance. This might involve smarter algorithms or even new hardware solutions. It's about making these powerful tools accessible to more people and applications.
The Impact of Multimodal LLMs on Society
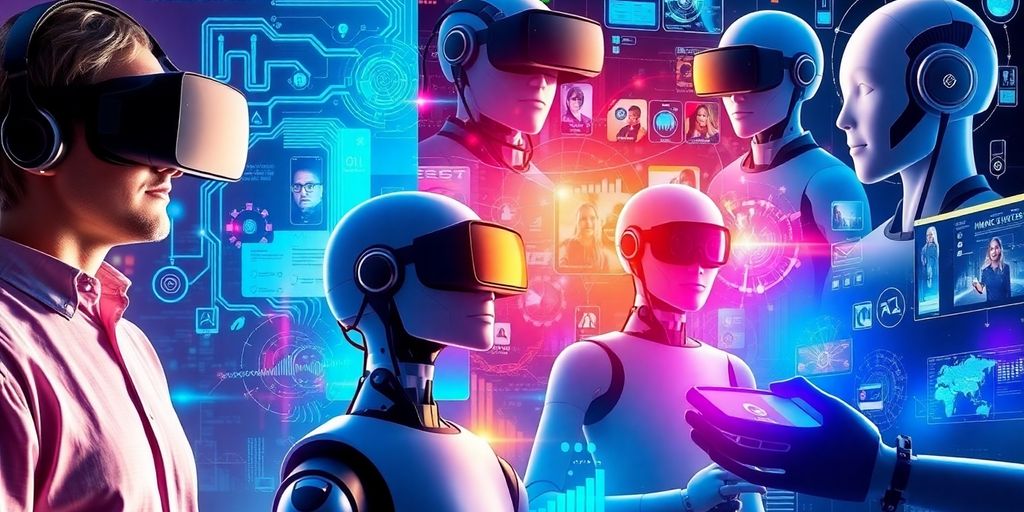
Transforming Education and Learning
Multimodal large language models (LLMs) are set to transform education by creating more interactive and personalized learning experiences. Imagine a classroom where students can interact with AI tutors that understand not just text but also images and sounds. These AI systems can tailor lessons to individual learning speeds and styles, making education more accessible and engaging. Teachers could use these tools to create rich multimedia content that captures students' attention and promotes deeper understanding.
- Personalized Learning: AI tutors adjust content based on student progress.
- Interactive Lessons: Incorporating videos and audio to enhance engagement.
- Accessibility Improvements: Supporting students with different learning needs.
Influencing Media and Entertainment
In the realm of media and entertainment, multimodal LLMs are opening up new avenues for creativity and content creation. Filmmakers and game developers can leverage these technologies to create immersive experiences that seamlessly blend visual and auditory elements. For example, AI can generate realistic dialogue and soundscapes for virtual environments, enhancing storytelling.
- Interactive Storytelling: AI-driven narratives that respond to audience input.
- Enhanced Graphics: Real-time rendering of complex scenes.
- Sound Design: AI-generated soundtracks tailored to the mood of the scene.
Shaping Healthcare and Medicine
The transformative power of LLMs in healthcare is undeniable. These models are revolutionizing diagnostics and patient care by integrating data from various sources like medical images, patient histories, and genetic information. Doctors can use multimodal AI to make more accurate diagnoses and develop personalized treatment plans.
- Improved Diagnostics: Combining imaging, text, and lab results for better accuracy.
- Patient Monitoring: Real-time analysis of patient data for timely interventions.
- Research Advancements: Accelerating drug discovery and development.
As we look to the future, the integration of multimodal LLMs into these sectors promises not just innovation but also a more connected and efficient society. The potential applications are vast, and as technology progresses, the impact on our daily lives will be profound.
Comparative Analysis of Multimodal LLMs
Performance Across Different Tasks
When it comes to evaluating the performance of multimodal large language models (MLLMs), it's crucial to understand how these models handle various tasks. MLLMs are designed to process and integrate data from multiple modalities, such as text, images, and audio. This integration allows them to perform tasks like image captioning, where they generate text descriptions for visual content, or video analysis, where they combine audio and visual data for a comprehensive understanding.
One notable benchmark for assessing MLLMs is the MLLM-CompBench, which evaluates their ability to reason across different modalities. This benchmark helps to highlight the strengths and weaknesses of various models in handling complex multimodal tasks. Generally, larger models with more parameters tend to perform better, but they also require more computational resources.
Strengths and Weaknesses
MLLMs offer significant advantages over traditional single-modality models. By combining different types of data, they can provide richer, more detailed insights. For instance, in the context of video analysis, MLLMs can utilize both visual and audio cues to deliver more accurate results. However, this complexity also introduces challenges.
A major weakness of MLLMs is their interpretability. The integration of diverse data sources often results in a "black box" effect, making it difficult to understand how the model arrives at specific conclusions. This lack of transparency can be problematic in critical areas like healthcare, where understanding the decision-making process is essential.
Future Research Opportunities
Looking ahead, there are several exciting research opportunities for MLLMs. One area of interest is improving the interpretability of these models. By developing methods to better understand how different modalities contribute to the final output, researchers can enhance the trustworthiness of MLLMs, especially in sensitive fields.
Another promising direction is exploring new modalities beyond text, images, and audio. As technology advances, incorporating emerging data types could further expand the capabilities of MLLMs. Additionally, balancing the trade-off between model size and efficiency remains a key challenge. Smaller, more specialized models may offer superior performance in specific domains, while larger models continue to provide broad generalization capabilities.
In summary, MLLMs represent a significant leap forward in AI technology. They enable more nuanced interactions with data, pushing the boundaries of what machines can achieve. As we continue to explore their potential, it's crucial to address the challenges they present to fully harness their capabilities.
Conclusion
So, there you have it. Multimodal LLMs are not just a passing trend; they're shaping up to be a big part of our tech future. These models are doing some pretty wild stuff, like handling text, images, and sounds all at once. It's like having a Swiss Army knife for data. Sure, there are challenges, like making sure everything works smoothly together and doesn't eat up too much computer power. But the potential is huge. Imagine AI that can understand and interact with the world almost like we do. That's where we're headed. It's an exciting time, and who knows what we'll see next. Maybe AI that can write its own blog posts? Well, let's not get ahead of ourselves. For now, it's clear that the future of multimodal LLMs is bright and full of possibilities.
Frequently Asked Questions
What are Multimodal LLMs?
Multimodal LLMs are advanced AI systems that can understand and process different types of information like text, images, audio, and video all at once.
How do Multimodal LLMs work?
They combine different types of data using special techniques to understand and generate content. This helps them perform tasks that involve multiple types of information.
Why are Multimodal LLMs important?
These models are important because they can handle complex tasks that involve different kinds of data, making them useful in many fields like healthcare, entertainment, and more.
What challenges do Multimodal LLMs face?
They face challenges like needing a lot of computing power and having trouble explaining how they make decisions. They also need to be better at protecting data privacy.
Where can Multimodal LLMs be used?
They can be used in areas such as language translation, image and video analysis, and audio processing, helping to improve these technologies.
What is the future of Multimodal LLMs?
The future involves making these models more efficient and exploring new ways to use them, possibly in new fields and applications.