Exploring the Disadvantages of AI in Healthcare: Challenges and Risks
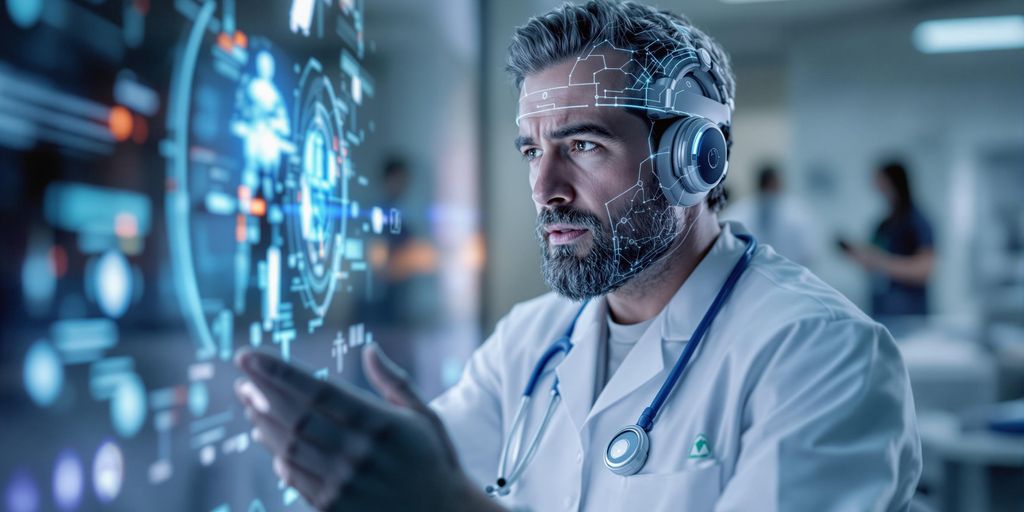
Artificial intelligence (AI) is becoming a big part of healthcare, and while it can do a lot of good, it's not all sunshine and rainbows. There are some serious downsides that we need to think about. From privacy issues to the risk of over-relying on machines for diagnosis, AI in healthcare brings a bunch of challenges and risks. It's important to take a closer look at these issues so we can figure out how to deal with them.
Key Takeaways
- AI in healthcare can lead to privacy concerns as sensitive patient data might be at risk.
- There's a chance of AI systems being biased, which can affect treatment outcomes.
- Overreliance on AI for diagnosis might result in undervaluing human judgment.
- The cost of implementing AI technologies can be a barrier for smaller healthcare facilities.
- AI can potentially increase health disparities if access to technology isn't equal.
Understanding the Ethical Implications of AI in Healthcare
Patient Privacy Concerns
AI in healthcare can be a double-edged sword, especially when it comes to patient privacy. The need for large datasets to train AI systems means that a lot of patient information is collected. This can make people uneasy, worrying that their personal health details might be shared without their permission. Imagine an AI predicting a condition you didn’t even know you had, just by analyzing how you use your computer mouse. That might sound like science fiction, but it’s a reality we’re facing. The big question is, who gets to see this information? Banks? Insurance companies? Keeping this data safe and private is a major challenge.
Informed Consent Challenges
Informed consent is another tricky area. When AI systems are involved in healthcare, patients need to know exactly what’s happening with their data and how decisions about their care are being made. But let’s be honest, AI can be a bit of a "black box." It’s not always clear how it reaches its conclusions, which makes explaining it to patients tough. Patients deserve to know the risks and limitations of AI in their treatment plans. It’s about transparency and trust.
Bias in AI Algorithms
Bias in AI is a big deal. These systems learn from data, and if that data is biased, the AI will be too. This can lead to unfair treatment of certain groups. For example, if an AI is trained mostly on data from one demographic, it might not work as well for others. This is a problem because healthcare should be fair and equal for everyone. Tackling bias means using diverse datasets and continuously checking for fairness. It’s not just about getting the right answers; it’s about getting them for everyone.
"Even if AI systems learn from accurate, representative data, there can still be problems if that information reflects underlying biases and inequalities in the health system."
The Risk of Overreliance on AI Diagnostics
Potential for Misdiagnosis
Incorporating AI into healthcare diagnostics can be a double-edged sword. While AI systems offer impressive accuracy, they are not infallible. Mistakes can happen, and when they do, the consequences might be severe. An AI might misinterpret a scan or overlook subtle signs that a human doctor would catch, leading to potential misdiagnosis. This risk is amplified when clinicians rely too heavily on AI outputs without cross-verifying them with traditional methods or their own expertise.
Undervaluing Human Expertise
The human touch in healthcare is irreplaceable. AI might analyze vast amounts of data quickly, but it lacks the nuanced understanding that comes from years of human experience. When AI becomes the primary decision-maker, there's a risk that the invaluable insights of seasoned professionals could be sidelined. It's crucial to maintain a balance where AI supports, rather than replaces, the judgment of healthcare professionals.
Impact on Clinical Decision-Making
AI has the potential to revolutionize clinical decision-making, but it also poses risks. Overreliance on AI can lead to a passive approach in decision-making, where healthcare providers might defer entirely to AI recommendations. This could stifle critical thinking and reduce the clinician's role to that of a mere executor of AI-driven decisions. It's essential for medical professionals to remain actively engaged in the decision-making process, using AI as a tool rather than a crutch.
"In the end, while AI can enhance diagnostic processes, it should never replace the critical thinking and empathy that human doctors bring to patient care."
Data Security and Privacy Risks in AI Healthcare Systems
Vulnerability to Cyber Attacks
AI in healthcare is a double-edged sword. While it offers amazing tools for improving patient outcomes, it also opens the door to cyber threats. Healthcare systems are prime targets for hackers because they hold a ton of sensitive data. With AI systems, the risk multiplies as these systems often lack the robust security measures needed to fend off attacks. Cybercriminals can exploit these weaknesses, leading to unauthorized access and data theft. It's like a digital goldmine for them. To counter these threats, healthcare providers must invest in stronger security protocols and regular system audits.
Data Breach Consequences
When a data breach hits a healthcare facility, the aftermath can be devastating. Imagine your personal health information out in the open; it's a nightmare. Breaches not only compromise patient privacy but also shake trust in healthcare providers. The fallout includes financial losses, legal battles, and damaged reputations. Patients may hesitate to share crucial information, fearing it might not be safe, which can hinder effective care. Healthcare organizations need to prioritize data protection to avoid such scenarios.
Ensuring Patient Confidentiality
Patient confidentiality is the backbone of healthcare ethics. Maintaining this confidentiality in the age of AI is challenging but vital. AI systems must be designed to protect personal health information from unauthorized access and misuse. This involves implementing strict access controls and encryption methods. Patients deserve peace of mind knowing their data is secure. Healthcare providers must ensure that AI technologies comply with privacy laws and regulations to maintain trust and uphold ethical standards.
"In the race to harness AI's potential, we must not overlook the importance of protecting patient data. It's not just about innovation; it's about trust and responsibility."
Addressing the Bias in AI Healthcare Applications
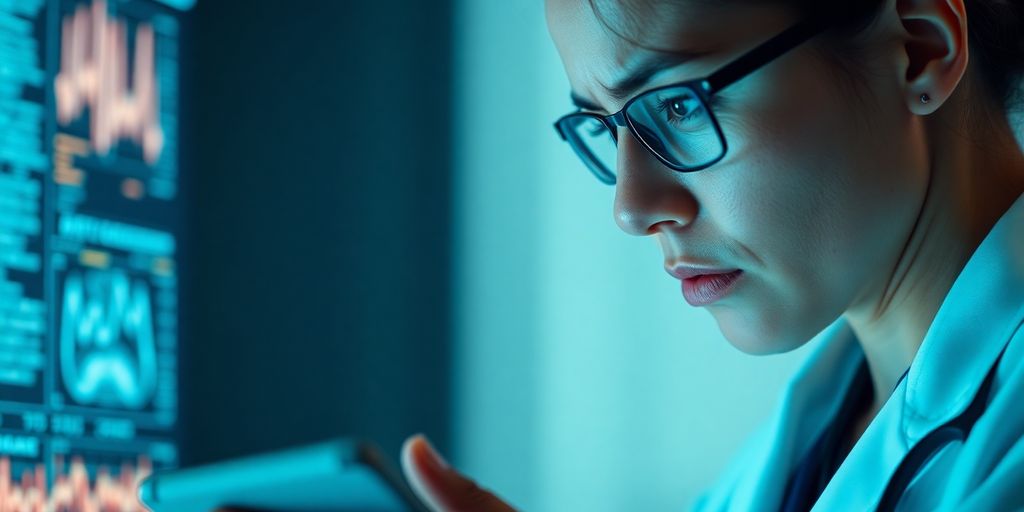
Sources of Bias in AI
Bias in AI systems stems from the data they are trained on. If the data reflects existing societal prejudices, the AI can inadvertently perpetuate these biases. For instance, if an AI is trained on records predominantly from one demographic, it may not perform well for others. This is a significant issue in healthcare, where diverse datasets are crucial for accurate predictions and diagnoses. The reliance on historical data can mean that outdated or biased practices are encoded into the AI, leading to skewed results.
Impact on Minority Populations
The impact of biased AI on minority groups can be profound. AI systems might offer less accurate diagnoses or treatment recommendations to these populations, exacerbating existing health disparities. For example, an AI trained primarily on data from urban hospitals might not perform well in rural settings, where patient demographics and health issues can differ significantly. This can lead to unequal healthcare outcomes, further entrenching systemic inequities.
Strategies for Mitigating Bias
To counteract bias, several strategies can be employed:
- Diversifying Training Data: Ensuring that AI systems are trained on datasets that reflect a wide range of demographics and conditions.
- Regular Audits: Conducting frequent checks to identify and correct biases in AI algorithms.
- Algorithmic Transparency: Making the decision-making processes of AI systems more transparent to understand how conclusions are reached.
It's vital that the healthcare industry addresses these biases proactively. Ignoring them not only risks significant clinical consequences but also undermines the trust and effectiveness of AI applications in healthcare.
The Economic Impact of AI on Healthcare Employment
Job Displacement Concerns
AI's entry into healthcare is like a double-edged sword. On one hand, it promises efficiency and cost-cutting, but on the other, it raises fears of job displacement. As AI systems become more adept at handling routine tasks, there's a real worry about traditional roles being phased out. For instance, jobs that depend heavily on repetitive tasks, like data entry or basic diagnostics, might be at risk. However, it's important to note that while some jobs might disappear, new roles could emerge, especially in areas like AI management and maintenance.
Cost of AI Implementation
Implementing AI in healthcare isn't cheap. There's a significant upfront cost involved in acquiring the technology, training staff, and maintaining new systems. Despite these initial costs, AI can lead to long-term savings by reducing errors and improving efficiency. For example, AI automation in healthcare document management offers financial benefits by cutting labor costs and speeding up processes. It's a balancing act between the investment and the potential savings down the line.
Balancing Efficiency and Employment
The challenge lies in finding a balance between harnessing AI's efficiency and maintaining employment levels. AI can streamline operations, but it's crucial to ensure that it doesn't lead to a workforce that's too lean. One way to address this is by retraining existing staff to work alongside AI, ensuring they have the skills needed for new roles that AI might create. Embracing AI doesn't mean sidelining human workers; instead, it should be about enhancing their roles and providing opportunities for growth.
AI in healthcare significantly influences the economy, not only by reducing costs but also by creating new revenue streams and boosting productivity. However, the transition needs careful management to avoid negative impacts on employment.
Challenges in Integrating AI with Existing Healthcare Systems
Technical Compatibility Issues
Integrating AI into existing healthcare systems isn't just a plug-and-play situation. One major hurdle is technical compatibility. Many healthcare facilities operate on legacy systems that weren't designed to support the sophisticated algorithms AI requires. This mismatch can lead to significant challenges in achieving seamless integration. Compatibility issues may arise from outdated software, hardware limitations, or proprietary systems that don't easily communicate with AI technologies. Overcoming these obstacles often requires substantial updates or complete overhauls of existing systems, which can be both time-consuming and costly.
Training Healthcare Professionals
Introducing AI into healthcare means more than just updating tech; it also involves a learning curve for the people using it. Healthcare professionals need to be trained not only in how to use these new tools but also in understanding their limitations and potential pitfalls. Training programs should focus on practical applications, potential biases in AI recommendations, and the importance of human oversight. This kind of comprehensive training ensures that AI can be used effectively and safely, augmenting rather than replacing human expertise.
Maintaining System Reliability
Once AI is integrated, maintaining its reliability becomes a critical task. AI systems must operate consistently and accurately to be trusted in clinical settings. Regular monitoring and updates are necessary to ensure that these systems remain effective and secure. Additionally, contingency plans should be in place to address any system failures or inaccuracies promptly. This ongoing maintenance is crucial to maintaining trust in AI technologies and ensuring they deliver the promised benefits without compromising patient care.
"The integration of AI in healthcare systems offers transformative potential, yet faces significant challenges. Addressing these challenges is essential for realizing the full benefits of AI in improving healthcare outcomes."
- Adapted from insights on Integrating AI and machine learning into EHR systems.
The Legal and Regulatory Challenges of AI in Healthcare
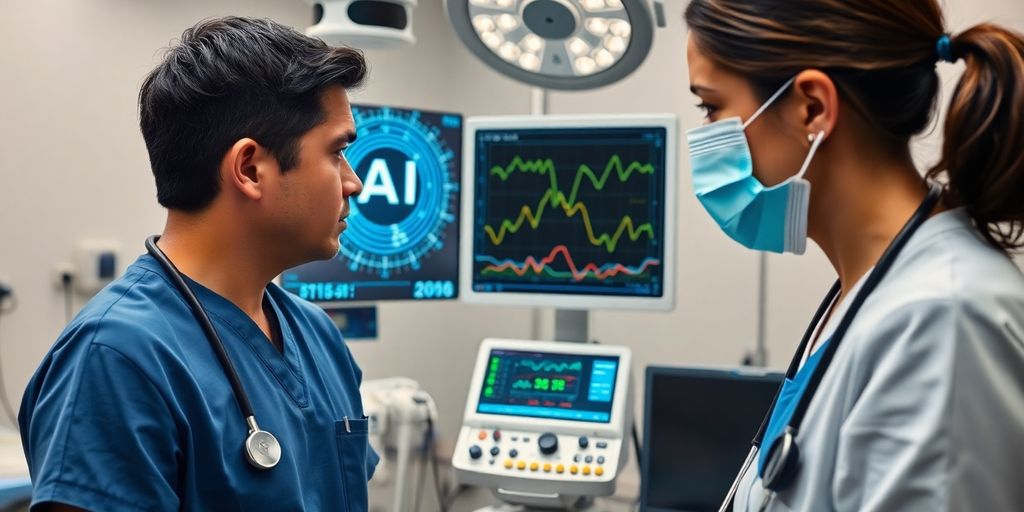
Liability in AI-Driven Decisions
When AI systems make decisions in healthcare, who takes the blame if things go wrong? That's a big question. AI in healthcare brings up tricky issues about responsibility. If a diagnosis or treatment suggested by AI leads to harm, figuring out who's liable can be a tangled mess. Is it the software developer, the healthcare provider, or the institution? This uncertainty can make it hard for healthcare providers to fully trust and use AI systems.
Regulatory Compliance Requirements
As AI becomes more common in healthcare, regulatory frameworks are scrambling to keep up. Current laws often don't fit well with AI's unique capabilities and risks. There's a push for new regulations that can handle AI's complexities, ensuring that these systems are safe and effective. Healthcare regulation in the U.S. is evolving to address these challenges, but it's a work in progress. The goal is to craft rules that protect patients without stifling innovation.
Developing AI-Specific Legislation
Creating laws specifically for AI in healthcare is a daunting task. It involves balancing innovation with safety and privacy concerns. Policymakers are working to draft legislation that addresses the unique aspects of AI, like its potential for bias and its need for vast amounts of data. This legislation aims to ensure that AI tools are used safely and ethically, providing a framework for their adaptable integration into healthcare systems.
"Navigating the legal landscape of AI in healthcare is like walking a tightrope—it's about finding the right balance between innovation and regulation."
The Limitations of AI in Understanding Human Emotions
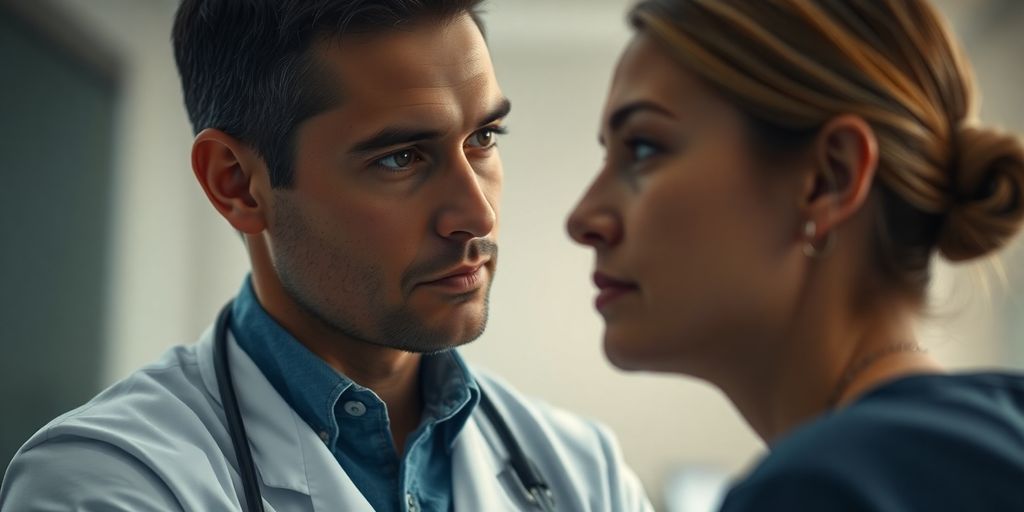
Lack of Empathy in AI Systems
AI in healthcare is pretty impressive, right? It's great at crunching numbers and analyzing data. But when it comes to emotions, it's a whole different ball game. AI just can't feel empathy like humans do. This gap in emotional intelligence is a big deal, especially for AI's cognitive abilities in complex decision-making. Sure, AI can simulate a caring response, but it doesn't actually feel anything. This lack of genuine empathy can be a problem, particularly in areas like mental health care where understanding and emotional support are key.
Importance of Human Interaction
Even with all the tech advancements, the human touch is irreplaceable. People need that personal connection, especially when dealing with health issues. Imagine going through a tough diagnosis and only talking to a machine—sounds cold, right? Human interaction provides comfort and understanding that AI can't match. Plus, emotional connections with AI are limited, often depending on how well individuals can connect with real people. This makes human involvement crucial in healthcare.
AI's Role in Mental Health Care
AI is making strides in mental health care, offering data-driven insights and support. But it's not a replacement for human therapists. AI lacks the ability to fully grasp human experiences and misses out on social cues, which are essential in therapy. This highlights the limitations of AI and the irreplaceable role of human psychotherapists. While AI can assist with monitoring and initial assessments, the depth of understanding and empathy required in mental health care is something only humans can provide.
AI can do a lot in healthcare, but when it comes to emotions and empathy, it still has a long way to go. The human element is essential for truly understanding and connecting with patients.
The Potential for AI to Widen Health Disparities
Access to AI Technologies
Artificial Intelligence (AI) in healthcare promises many benefits, but not everyone gets to enjoy them equally. Disparities in access to AI technologies may exacerbate healthcare inequalities among different groups. For instance, rural areas often lack the infrastructure needed to support advanced AI systems, leaving them behind urban centers. Similarly, smaller clinics might not afford the latest AI tools, unlike bigger hospitals with more resources. This gap means that some patients receive more advanced care than others, simply based on where they live or the resources of their healthcare provider.
Socioeconomic Barriers
Socioeconomic factors play a huge role in healthcare access and outcomes. AI's integration into healthcare can unintentionally widen these gaps. Patients from low-income backgrounds might struggle to access AI-driven treatments or diagnostics due to high costs. Additionally, the digital divide—where some people lack internet access or digital literacy—can prevent these patients from benefiting from AI's potential. These barriers make it harder for disadvantaged groups to receive timely and effective care.
Ensuring Equitable Healthcare
To prevent AI from worsening health disparities, the healthcare community must focus on ensuring equitable access to these technologies. This involves not only making AI tools more affordable but also addressing biases that might exist in AI algorithms. A recent seminar at Penn LDI discussed how healthcare algorithms can both improve and exacerbate disparities in health outcomes. Strategies could include investing in infrastructure for underserved areas, providing training programs to improve digital literacy, and developing policies that ensure AI tools are used fairly across all populations.
AI holds the potential to transform healthcare, but without careful consideration and planning, it risks leaving the most vulnerable behind.
The Unintended Consequences of AI in Patient Care
Loss of Personal Touch
AI systems, with their precise algorithms and data-driven insights, often miss the human element that is vital in healthcare. Patients can feel neglected when their care is overly reliant on machines. The absence of a human connection can lead to a sense of isolation, especially for those who value personal interaction in their care journey. While AI can streamline processes, it often lacks the warmth and empathy that human caregivers provide.
Dependence on Technology
There's a growing worry that the healthcare sector might become too reliant on AI. This dependence can lead to complacency among healthcare professionals, potentially reducing their skills over time. If systems fail or data is incorrect, the consequences can be severe. It's crucial to maintain a balance between utilizing AI and preserving human oversight to ensure that technology enhances rather than hinders patient care.
Patient Trust and Satisfaction
Trust is the cornerstone of the patient-provider relationship. As AI becomes more prevalent, patients may question the reliability of their care if they perceive that decisions are made by machines rather than humans. Patient satisfaction could decline if they feel their concerns aren't heard or if they suspect that AI-driven decisions lack the nuance of human judgment. Building confidence in AI systems is essential to maintain trust and satisfaction in healthcare.
In the quest for efficiency, healthcare must not lose sight of the human touch. Balancing technology with personal care is key to sustaining trust and satisfaction among patients.
Ensuring the Safety and Reliability of AI in Healthcare
Testing and Validation Protocols
Before any AI system is deployed in a clinical setting, it must undergo thorough testing and validation. This process is crucial to confirm that the system performs as expected under various conditions. Rigorous testing protocols help identify potential flaws or biases in AI algorithms. These protocols should include:
- Simulated clinical environments to test AI responses.
- Comparison against human expert decisions to assess accuracy.
- Stress testing to evaluate performance under high-demand scenarios.
Monitoring AI Performance
Continuous monitoring of AI systems is essential to maintain their reliability and effectiveness. Regular checks can help detect deviations from expected performance, allowing for timely interventions. This monitoring should involve:
- Automated alerts for unusual system behavior.
- Routine audits of AI decisions against patient outcomes.
- Feedback loops with healthcare professionals to refine AI tools.
Addressing AI System Failures
Even with the best precautions, AI systems can fail. It's vital to have a plan in place for such events to minimize harm to patients. Steps to address failures include:
- Immediate shutdown protocols to prevent further errors.
- Detailed incident reports to understand the cause of failure.
- Regular updates and patches to fix identified issues.
"In the fast-paced world of healthcare, the importance of AI safety cannot be overstated. Regular evaluations and a detailed inventory of AI systems are key to identifying and mitigating risks effectively."
By focusing on these areas, healthcare organizations can ensure that AI technologies are not only innovative but also safe and reliable for patient care.
The Future of AI in Healthcare: Balancing Innovation and Risk
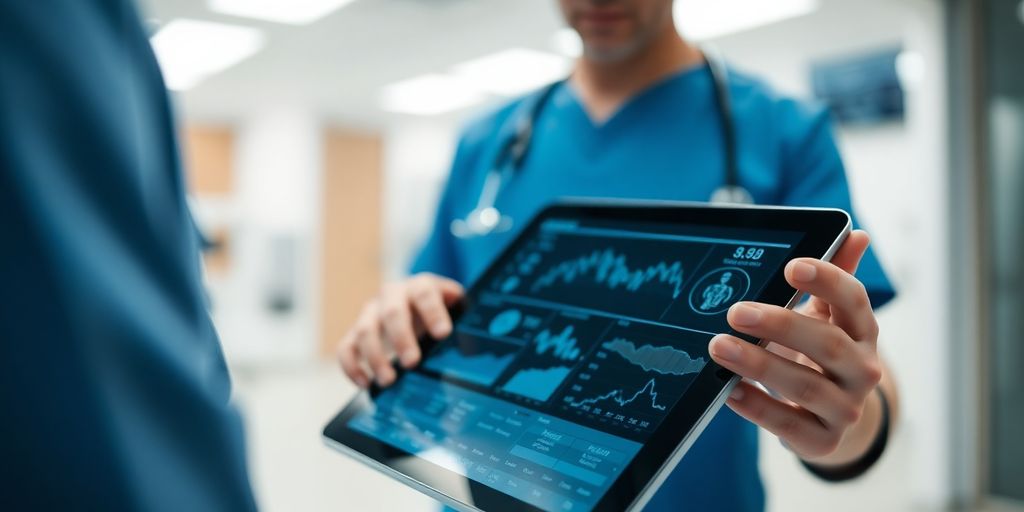
As we look ahead, the landscape of healthcare is poised to be reshaped by emerging AI technologies. From predictive analytics that anticipate patient needs to AI-driven robots assisting in surgeries, the possibilities seem endless. However, with great potential comes the responsibility to tread carefully. Healthcare providers must balance innovation with the ethical and practical challenges these technologies present. While AI can enhance capabilities, it also demands rigorous testing and validation to ensure patient safety.
In the rush to integrate AI into healthcare, ethical considerations must not be sidelined. Issues like patient privacy, data security, and algorithmic bias require careful thought. AI in healthcare raises questions about who is accountable when machines make decisions. It's crucial to develop frameworks that address these ethical dilemmas, ensuring that AI advancements do not compromise patient trust or safety. As technology evolves, so must our ethical standards and regulatory measures.
Transitioning to an AI-driven healthcare system isn't just about adopting new tools; it's about preparing the workforce and infrastructure. Training healthcare professionals to work alongside AI tools is essential, as is updating systems to support these technologies. Investments in education and infrastructure will be key to a successful integration. Moreover, continuous monitoring and assessment of AI systems will help in adapting to unforeseen challenges and ensuring that AI serves as a complement to human expertise, not a replacement.
As AI continues to evolve, the healthcare sector must remain vigilant, ensuring that innovation does not outpace the ethical and practical considerations vital to patient care. Balancing these elements will determine the success of AI integration in healthcare.
Conclusion
So, there you have it. AI in healthcare is a bit of a double-edged sword. Sure, it's got the potential to change the game with faster diagnoses and personalized treatment plans. But let's not kid ourselves—there are some pretty big hurdles to jump over. Privacy issues, the risk of errors, and the fear of losing that human touch are all real concerns. Plus, there's the whole thing about AI being a bit of a mystery box, making decisions that even experts can't always explain. As we move forward, it's crucial to keep these challenges in mind and work towards solutions that balance innovation with caution. After all, the goal is to make healthcare better for everyone, not just more high-tech.
Frequently Asked Questions
What is AI in healthcare?
AI in healthcare refers to using computer systems to analyze and interpret medical data, helping doctors diagnose and treat patients more effectively.
How does AI impact patient privacy?
AI can impact patient privacy by handling large amounts of sensitive data, which, if not properly secured, could lead to privacy breaches.
Can AI replace doctors?
AI cannot replace doctors because it lacks the human touch and empathy necessary for patient care. It serves as a tool to assist doctors, not replace them.
What are the risks of relying too much on AI for diagnosis?
Relying too much on AI for diagnosis can lead to misdiagnosis if the AI system makes errors or misses subtle cues that a human doctor would catch.
How does AI create job concerns in healthcare?
AI might lead to job concerns by automating tasks that healthcare workers currently perform, which could reduce the need for certain jobs.
What are some challenges of using AI in healthcare?
Challenges include ensuring data privacy, preventing biases in AI algorithms, and integrating AI systems with existing healthcare infrastructure.
How can AI widen health disparities?
AI might widen health disparities if access to AI technologies is unequal, leading to differences in the quality of care received by different populations.
What steps can be taken to ensure AI is used safely in healthcare?
To ensure AI is used safely, healthcare providers should follow strict testing and validation protocols, monitor AI performance, and address any system failures promptly.