Exploring the Disadvantages of AI in Healthcare: Challenges and Concerns
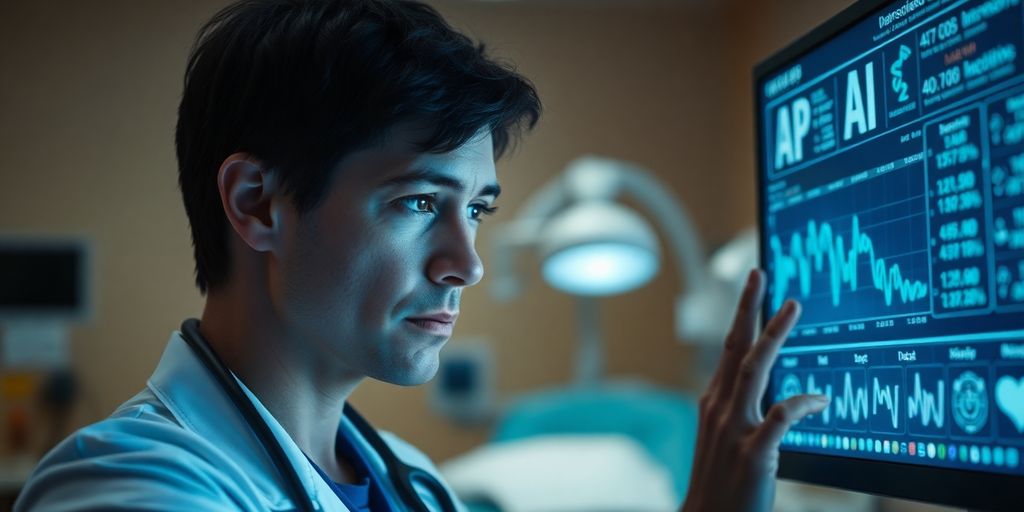
AI in healthcare is a hot topic these days. While it's bringing some cool advancements, it's not all sunshine and rainbows. There are some real downsides that we can't ignore. From privacy issues to biases in data, AI can sometimes cause more harm than good. And let's not forget the cost, which can be a big hurdle for many facilities. This article dives into the not-so-great side of AI in healthcare, focusing on the challenges and concerns that come with it.
Key Takeaways
- AI can jeopardize patient privacy, as sensitive data might be mishandled or exposed.
- Bias in AI systems can lead to unfair treatment and health disparities among different populations.
- The high cost of AI implementation can create economic barriers, limiting access to advanced healthcare technologies.
- Overreliance on AI for diagnosis may lead to misdiagnosis and undervalue the expertise of human healthcare providers.
- The lack of transparency in AI decision-making processes can hinder trust and understanding among healthcare professionals.
Ethical Concerns in AI-Driven Healthcare
Patient Privacy and Consent Issues
AI in healthcare is like a double-edged sword. While it can bring great benefits, it also raises some serious ethical questions. Patient privacy is a big one. With AI systems handling tons of sensitive data, there's always a risk of that data being misused or exposed. Imagine your personal health details floating around without your consent. That's a nightmare for anyone. Plus, AI can sometimes predict private info about patients that they might not even know themselves. How creepy is that?
Accountability in AI Decision-Making
Another major concern is accountability. When AI systems make decisions, who takes the blame if something goes wrong? Is it the developers, the healthcare providers, or the AI itself? This lack of clear accountability can be a huge problem. It’s like when a self-driving car crashes—who’s responsible? In healthcare, this can have even more serious consequences. We need to figure out who holds the reins when AI makes a bad call.
Balancing Human and Machine Roles
Finding the right balance between human expertise and AI capabilities is crucial. While AI can process data faster than any human, it lacks the empathy and nuanced understanding that doctors bring to the table. We can't just hand over the reins to machines entirely. Doctors and AI need to work together, each playing to their strengths. It's about finding that sweet spot where technology enhances human care without overshadowing it.
As AI continues to evolve, the healthcare industry must tread carefully, ensuring that ethical guidelines keep pace with technological advancements. Balancing innovation with responsibility is key to making sure AI benefits everyone without compromising ethical standards.
Data Privacy and Security Risks
Vulnerability to Data Breaches
In the healthcare world, data breaches are a constant worry. Hospitals and clinics gather heaps of sensitive patient information, which makes them prime targets for cyberattacks. Hackers often see healthcare data as a goldmine because it can be used for identity theft or sold on the dark web. This is why robust cybersecurity measures are essential to protect patient data. Despite these efforts, breaches still happen, sometimes exposing thousands of records at once.
Ensuring Patient Confidentiality
Patient confidentiality is the backbone of trust in healthcare. When AI systems process medical data, they must keep this information safe and private. The challenge is that AI needs lots of data to work well, which sometimes means sharing information across different platforms. This can lead to privacy concerns if not managed properly. Healthcare providers must implement strict protocols to ensure that patient info doesn't fall into the wrong hands.
Regulatory Compliance Challenges
Navigating the maze of regulations like HIPAA in the U.S. or GDPR in Europe is tricky. These laws are meant to protect patient data, but they also create hurdles for healthcare providers. AI systems must be designed to comply with these regulations, which can be a complex process. Failure to comply can result in hefty fines and legal issues. Balancing regulatory compliance with the need for innovation is a tightrope act that healthcare organizations must master.
Bias and Inequality in AI Systems
Impact of Biased Training Data
AI systems in healthcare often learn from existing datasets, which might not be as unbiased as we'd like. If these datasets mostly include data from certain groups, the AI might not work well for others. For example, if a dataset mainly consists of data from urban hospitals, the AI might not perform well in rural settings. This algorithm bias can lead to unfair treatment recommendations, especially for those not well represented in the data.
Addressing Health Disparities
Dealing with health disparities is crucial. AI can sometimes make these disparities worse if not handled carefully. For instance, if an AI system is trained on data that includes historical biases, it might continue those biases in its decisions. There are initiatives, like certain funding opportunities, aiming to tackle these issues by promoting projects that address these disparities.
Ensuring Fairness in AI Algorithms
To ensure fairness, it's important to regularly check AI systems for bias and update them with diverse datasets. This means including data from various demographics to make sure AI tools provide fair treatment to everyone. Regular audits and diverse training data are key steps in this process. Moreover, promoting equitable access to AI advancements can help bridge the digital divide, ensuring all communities benefit from AI in healthcare.
"Even with the best data, AI can still reflect existing biases in the healthcare system, which is why continuous monitoring and adjustments are necessary."
Overreliance on AI Diagnostics
Potential for Misdiagnosis
AI has been a game-changer in healthcare, but it's not without its pitfalls. Relying heavily on AI for diagnostics can lead to misdiagnosis. These systems, while sophisticated, can make errors, especially if they're based on biased data or flawed algorithms. Imagine an AI missing a tumor on a scan or suggesting the wrong medication. Such errors can have serious consequences, impacting patient health and trust in technology.
Undervaluing Human Expertise
There's a risk that as AI becomes more prevalent, the expertise of healthcare professionals might be undervalued. Doctors and nurses bring a wealth of experience and intuition that AI simply can't replicate. They notice subtle cues and have the empathy needed in patient care. Overreliance on AI could lead to a scenario where human judgment is sidelined, which isn't ideal for complex medical decisions.
Balancing AI and Human Judgment
Finding the right balance between AI and human judgment is crucial. AI can process vast amounts of data quickly, offering insights that might take humans much longer to deduce. However, it's essential to combine this with human oversight. A collaborative approach ensures that AI aids rather than replaces human decision-making. This balance helps prevent diagnostic bias and ensures that technology enhances rather than hinders medical practice.
AI in healthcare is a tool, not a replacement for human touch. It should complement, not substitute, the nuanced understanding and care that healthcare professionals provide.
Transparency and Explainability Challenges
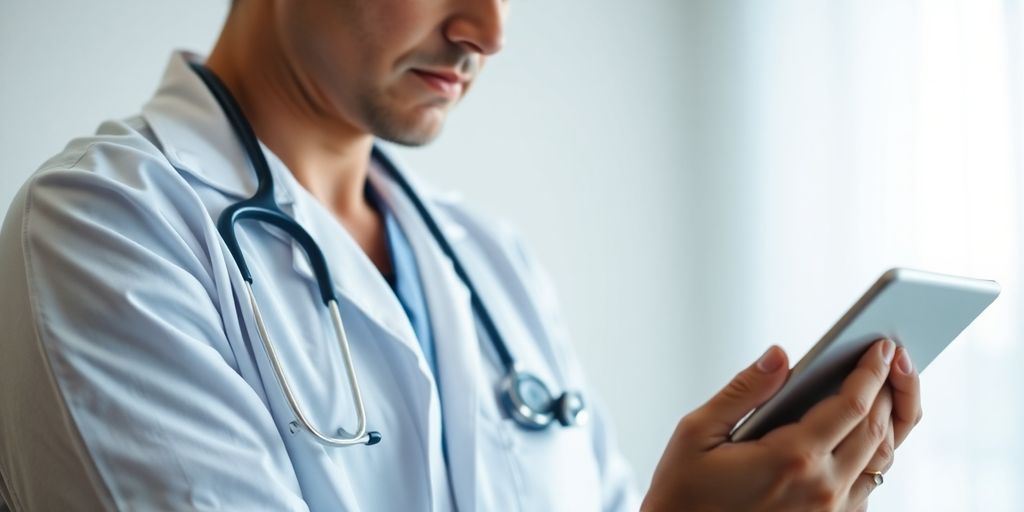
Understanding AI Decision Processes
One of the biggest hurdles in healthcare AI is figuring out how these systems make decisions. Often, AI models function like a "black box," where the logic behind decisions is hidden. This lack of transparency can make it hard for doctors and patients to trust AI outcomes. Being able to explain AI decisions is crucial for building trust and ensuring that these technologies are safe to use in medical settings.
Black-Box Nature of AI Systems
Many AI systems, especially those using deep learning, operate in a way that's not easily understandable to humans. This "black-box" nature means that the inner workings of AI models are often invisible, which can lead to diminished trust and transparency. In healthcare, where decisions can be life-altering, this lack of clarity is a significant concern.
Improving AI Interpretability
Efforts are being made to make AI more understandable. Explainable AI (XAI) is a step in the right direction, aiming to shed light on how AI systems come to their conclusions. This innovation is transforming healthcare by making AI systems more transparent and accountable. The goal is to ensure that AI tools are not only effective but also trustworthy, providing clear and understandable insights into their decision-making processes.
Cost and Accessibility Issues
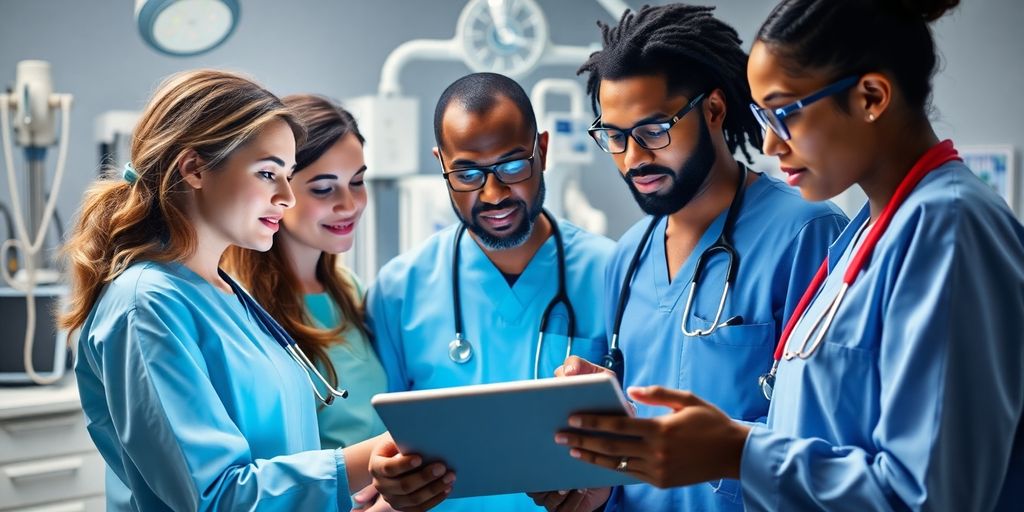
High Implementation Costs
Implementing AI in healthcare isn't cheap. From buying the technology to training staff, the expenses add up quickly. Hospitals and clinics often face hefty initial costs, which can be a major barrier, especially for smaller facilities. These costs include software licenses, hardware upgrades, and the ongoing maintenance of systems. Even with the potential for long-term savings, the upfront investment can be daunting.
Resource Disparities in Healthcare
AI technology isn't equally accessible everywhere. Some hospitals have the resources to adopt the latest tech, while others lag behind. This disparity can widen the gap between urban and rural healthcare facilities, causing unequal access to quality care. In regions where resources are limited, AI implementation might not even be a possibility, leaving patients without the benefits that AI could provide.
Economic Barriers to AI Adoption
The economic landscape plays a big role in how AI is adopted in healthcare. For many institutions, the financial burden is too high, preventing them from embracing AI solutions. This is particularly true for publicly funded healthcare systems, which may struggle to allocate funds for such advancements. Moreover, the cost of AI-driven healthcare might be passed down to patients, making it less affordable for those who need it most.
AI in healthcare holds great promise, but the financial hurdles can't be ignored. The challenge is to find a way to make this powerful technology accessible to all, without compromising on quality or affordability.
- Initial setup costs can be prohibitive.
- Resource allocation varies greatly between institutions.
- Economic factors influence AI's reach and impact.
The promise of AI is there, but tackling these cost and accessibility issues is critical to ensuring that everyone can benefit from these technological advancements in healthcare.
Safety and Reliability Concerns
Ensuring AI System Accuracy
AI systems in healthcare are expected to be accurate, but sometimes they fall short. Imagine a system that doesn't spot a tumor on a scan or suggests a wrong medication. These aren't just mistakes; they're life-impacting errors. Accuracy is crucial because even a tiny error can lead to big issues. Plus, if an AI makes the same mistake repeatedly, it could affect many patients. Regular updates and checks are needed to keep these systems reliable.
Managing AI-Induced Errors
When AI systems make mistakes, it’s not just a technical glitch; it can have serious consequences. Errors might happen because of flaws in the algorithm or unexpected data inputs. To manage these, it's important to have a plan that includes quick error detection and a way to fix them fast. Healthcare providers need to be ready to step in when AI misfires, ensuring patient safety isn't compromised.
Continuous Monitoring and Evaluation
AI systems can't just be set up and forgotten. Continuous monitoring is key to spotting issues early. Regular evaluations help ensure the system works well across different environments and patient groups. It's like keeping an eye on a complex machine to make sure all parts are functioning smoothly. This ongoing process helps maintain trust in AI, ensuring it remains a helpful tool in healthcare.
Impact on Healthcare Workforce
Job Displacement Risks
AI in healthcare is shaking things up big time. While it offers a lot of promise, it also brings worries about job displacement. Some roles, especially those involving repetitive tasks, might become obsolete. For instance, tasks like data entry or simple diagnostics can be automated, leaving workers wondering about their job security. This shift might push some professionals out of their current roles, creating a need for retraining and adaptation.
Changing Roles of Healthcare Professionals
With AI stepping in, the roles of healthcare professionals are evolving. Doctors and nurses might find themselves focusing more on patient interaction and less on administrative tasks, thanks to AI handling the grunt work. This shift can be both exciting and challenging. On the one hand, it frees up time for more meaningful patient care; on the other, it demands new skills and a willingness to embrace technology. Here's how roles are changing:
- Increased Focus on Patient Interaction: With AI taking over routine tasks, healthcare workers can spend more time with patients.
- Need for Technical Proficiency: Professionals may need to learn how to work alongside AI tools, requiring new training.
- Shift in Responsibilities: Some tasks traditionally done by humans might be handled by AI, leading to a redistribution of duties.
Training and Skill Development Needs
As AI becomes more common in healthcare, the need for training and skill development is growing. Healthcare workers must become familiar with AI technologies and learn how to integrate them into their daily routines. Continuous education is crucial to keep up with the rapid advancements in AI. Training programs focusing on AI literacy, data management, and ethical considerations will be essential. Here's a look at what's needed:
- AI Literacy Programs: Understanding the basics of AI and its applications in healthcare.
- Data Management Skills: Knowing how to handle and interpret data generated by AI systems.
- Ethical Training: Learning about the ethical implications of AI in patient care.
The integration of AI in healthcare isn't just about technology; it's about transforming the workforce. As roles evolve, professionals must adapt, learning new skills and embracing the change. This transformation, while daunting, offers an opportunity for growth and improvement in patient care.
Legal and Regulatory Challenges
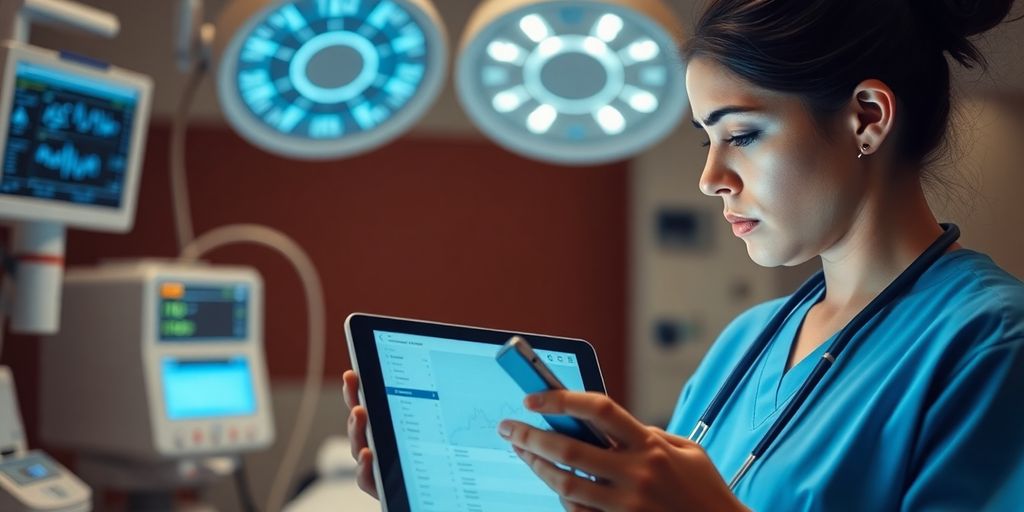
Establishing AI Governance Frameworks
Creating a governance framework for AI in healthcare is like trying to build a house on shifting sands. The technology is evolving so fast that any rules or guidelines we set today might be outdated tomorrow. Establishing a robust framework requires a careful balance between encouraging innovation and ensuring patient safety. It’s like walking a tightrope, and nobody wants to fall off. A solid framework could help manage risks and set clear standards for AI use in healthcare.
Liability in AI-Driven Decisions
Who’s to blame when AI makes a mistake? That’s the million-dollar question. Imagine a scenario where an AI system misdiagnoses a patient. Is it the developer’s fault, the hospital's, or the AI itself? This lack of clarity can lead to a legal quagmire. Assigning liability is tricky but necessary to protect patients and encourage responsible AI development. It’s not just about pointing fingers; it’s about making sure there’s accountability.
Adapting Regulations to AI Innovations
Keeping up with AI innovations is like chasing a moving target. Regulations need to be flexible yet firm, allowing for the rapid pace of technological change without compromising on safety and effectiveness. Regulatory bodies must work closely with tech developers to understand the nuances of AI technologies. This collaboration is crucial to ensure that regulations are not just a hindrance but a guide for safe and effective AI deployment in healthcare.
The challenge lies in creating laws that are comprehensive enough to cover the vast potential of AI, yet specific enough to address individual cases. This requires ongoing dialogue between lawmakers, tech experts, and healthcare professionals to ensure that regulations are both practical and protective.
In summary, tackling legal and regulatory challenges in AI healthcare is a complex task that requires collaboration and forward-thinking. From establishing frameworks to defining liability and adapting regulations, each step is crucial for the safe and effective use of AI in healthcare.
For more insights on how AI is transforming the healthcare landscape, check out Artificial intelligence (AI) in medicine offers significant benefits but poses challenges such as algorithmic bias, patient privacy concerns, and regulatory gaps and The use of AI tools in healthcare raises significant legal risks, particularly concerning data privacy and cybersecurity.
Ethical Implications of AI in Patient Care
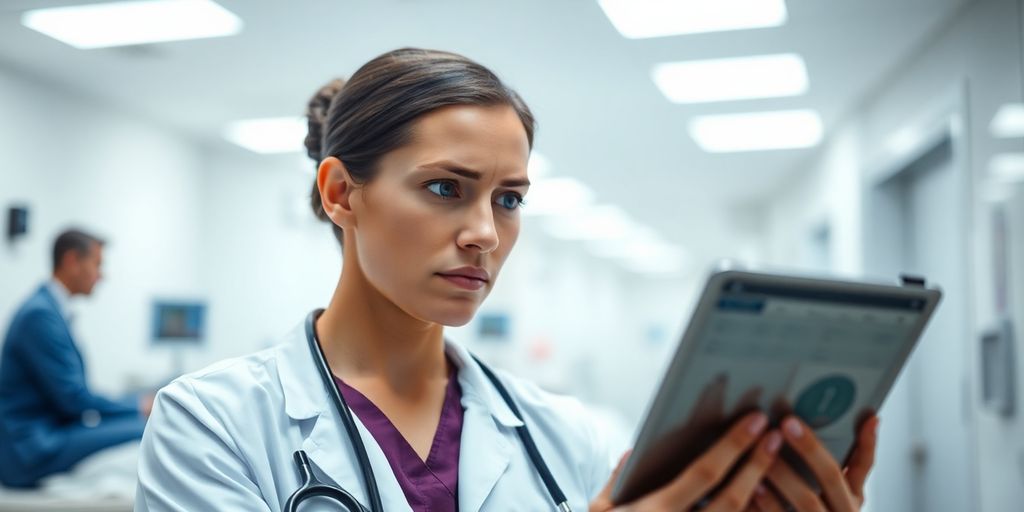
Moral Dilemmas in AI Usage
AI in healthcare brings up some tricky moral questions. Should a machine play a part in life-and-death decisions? It's a bit unsettling, right? Machines lack empathy, and their decisions can seem cold. Some folks worry about how much control we're handing over to algorithms. Balancing machine efficiency with human compassion is a real challenge.
Patient Autonomy and AI
Patients want control over their healthcare choices, and rightly so. When AI steps in, it can feel like losing that grip. Imagine an AI suggesting treatments without a doc's input—kinda scary, huh? It's crucial that patients are informed and consent to AI's role. This way, they know what's happening and can make choices that feel right to them.
Ethical Guidelines for AI Deployment
Setting up ethical guidelines is a must. We need clear rules about how AI should operate in healthcare. This includes ensuring transparency, respecting patient privacy, and defining accountability. Without guidelines, we risk chaos and mistrust in AI systems. Here's what we should focus on:
- Transparency: How does AI make its decisions?
- Accountability: Who's responsible if AI messes up?
- Privacy: Keeping patient data safe and sound.
AI in healthcare is like a double-edged sword. It can revolutionize treatment, but without proper ethical considerations, it might lead to more harm than good.
Challenges in AI Integration with Existing Systems
Compatibility with Current Technologies
Integrating AI into existing healthcare systems isn't always smooth sailing. Many hospitals are still using older systems that aren't designed to work with AI. These legacy systems can be rigid and hard to update, making it tough for new AI tools to fit in. Interoperability is a big word in this space, meaning how well different systems can talk to each other. When systems don't communicate well, it can lead to delays and errors in patient care.
Data Integration and Interoperability
Data is the backbone of any AI system, but getting all the data in one place is easier said than done. Healthcare facilities often have data spread across various platforms, each with its own format. This lack of standardization makes it hard to integrate AI effectively. Imagine trying to piece together a puzzle where none of the pieces fit—frustrating, right? That's what healthcare providers face when they try to integrate AI without a solid data strategy.
Scalability of AI Solutions
Once AI is up and running, the next challenge is scaling it to meet the demands of a growing patient population. Not all AI solutions are built to handle large volumes of data or users. This can be a major roadblock, especially in large hospital networks. To scale effectively, healthcare providers need to ensure their AI systems are robust and flexible enough to grow with their needs.
Implementing AI in healthcare is like trying to fit a square peg in a round hole. It requires a lot of adjustments and a willingness to change old ways of doing things. But with the right approach, the benefits can be huge.
Public Perception and Trust Issues
Building Trust in AI Technologies
Building trust in AI technologies in healthcare is a bit like trying to convince your grandma to use a smartphone. It takes time, patience, and a lot of explaining. People are naturally cautious about new tech, especially when it involves their health. They want to know that AI won't mess up their diagnosis or share their private info with the world. Trust is built through transparency and reliability. When AI systems prove they can consistently deliver accurate results, people start to relax a little. But it's not just about the tech working well; it's also about making sure the people using it understand it. This means doctors and patients need to be on the same page about how AI is being used and what it can and can't do.
Addressing Public Concerns
Addressing public concerns involves tackling a laundry list of worries. People are scared of data breaches, biased algorithms, and losing the human touch in healthcare. AI's black-box nature doesn't help here. When folks can't see how decisions are made, they're less likely to trust the outcome. To ease these fears, healthcare providers need to be proactive. This means having open conversations about how AI works and what safeguards are in place to protect patients. It's also about showing that AI isn't replacing doctors but helping them do their jobs better. When people see AI as a tool rather than a threat, they're more likely to accept it.
Promoting AI Literacy in Healthcare
Promoting AI literacy is like teaching a kid to ride a bike. At first, it seems daunting, but with the right guidance, it becomes second nature. In healthcare, this means educating both providers and patients about AI. For doctors, it's about understanding how AI can assist in diagnostics and treatment planning. For patients, it's about knowing what AI can do for their health and how it impacts their care. Lists of key points to focus on might include:
- Understanding the basics of how AI works in healthcare
- Recognizing the benefits and limitations of AI
- Knowing what questions to ask when AI is part of their treatment plan
AI in healthcare is here to stay, but for it to be effective, everyone involved needs to be comfortable and informed about its role. Just like any other tool, the more you know about it, the better you can use it.
Conclusion
So, AI in healthcare, huh? It's a mixed bag. On one hand, it promises to shake things up, making processes faster and maybe even more accurate. But let's not get ahead of ourselves. There are some real bumps in the road. We're talking about privacy issues, potential biases, and the fear of machines taking over jobs. Plus, there's the whole "black box" problem where nobody really knows how AI comes to its conclusions. It's like magic, but not the fun kind. And while AI can crunch numbers like a champ, it lacks the human touch that patients often need. So, as we move forward, it's crucial to keep these challenges in mind. Balancing innovation with caution will be key to making sure AI truly benefits healthcare without leaving anyone behind.
Frequently Asked Questions
How does AI affect patient privacy in healthcare?
AI can collect and analyze large amounts of patient data, which might put privacy at risk if not properly managed. Ensuring data is secure and used ethically is crucial.
What are the risks of AI making decisions in healthcare?
AI might make mistakes if it relies on wrong or biased data. It's important for humans to check AI decisions to avoid errors in patient care.
Why is there concern about AI replacing healthcare workers?
AI can perform some tasks faster, but it lacks the human touch and understanding. There's worry it might replace jobs, but it can also help workers by handling routine tasks.
How can AI lead to unfair treatment in healthcare?
If AI is trained on biased data, it might treat some patients unfairly. Ensuring AI systems are fair and unbiased is important to provide equal care.
What makes AI in healthcare expensive?
Setting up AI systems can be costly, requiring new technology and training. Smaller hospitals might struggle with these costs, affecting access to AI benefits.
Why is AI transparency important in healthcare?
Understanding how AI makes decisions helps doctors trust and use it correctly. If AI is like a 'black box,' it can be hard to know why it suggests certain treatments.
How does AI impact data security in healthcare?
AI needs lots of data, which can be a target for hackers. Protecting this data is important to keep patient information safe and private.
What are the legal challenges of using AI in healthcare?
Laws about AI in healthcare are still developing. It's important to have clear rules about who is responsible if AI makes a mistake.