Examining the Carbon Footprint of AI Training Models
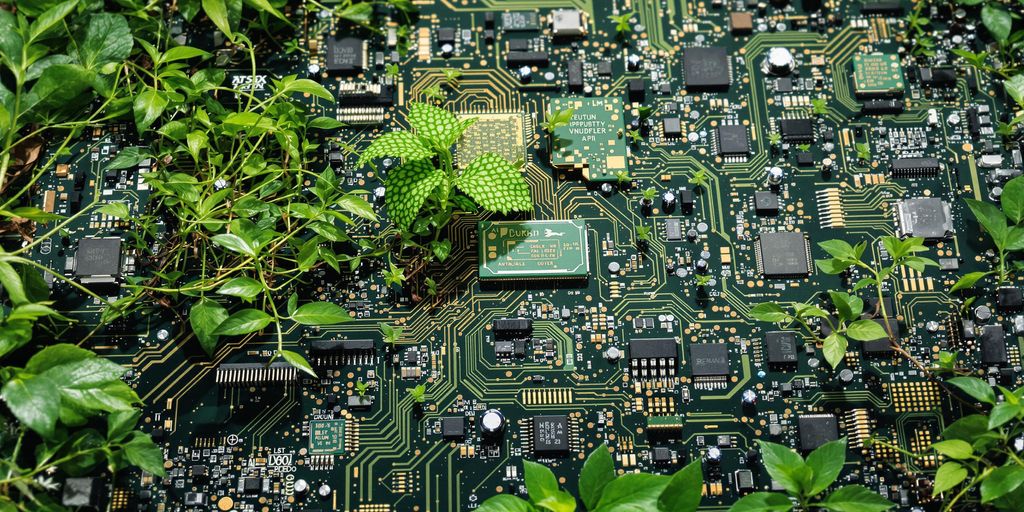
Artificial intelligence (AI) is transforming industries, but it comes with a hefty environmental price tag. Training AI models, especially the large ones, uses enormous amounts of energy, leading to significant carbon emissions. As AI becomes more integrated into our daily lives, it's crucial to examine its environmental impact and explore ways to make it more sustainable.
Key Takeaways
- Training AI models consumes a lot of energy, contributing to high carbon emissions.
- The size and complexity of AI models directly affect their environmental impact.
- Switching to renewable energy sources can significantly lower the carbon footprint of AI.
- Optimizing algorithms is a practical way to reduce energy use during training.
- Transparent reporting on energy consumption and emissions is essential for accountability.
Understanding the AI Carbon Footprint
Defining Carbon Footprint in AI Context
The "carbon footprint" of AI refers to the total greenhouse gas emissions produced during the lifecycle of AI systems. This includes emissions from training models, running algorithms, and maintaining the data centers that power them. AI systems require significant energy resources, and as a result, their carbon footprint is becoming a growing concern in the fight against climate change.
Key Contributors to AI's Carbon Emissions
Several factors drive AI's carbon emissions:
- Data center operations: These facilities consume massive amounts of electricity, primarily for computing and cooling.
- Training large models: Advanced AI models, especially those with billions of parameters, demand extensive computational power.
- Energy sources: The environmental impact heavily depends on whether the electricity used is from fossil fuels or renewable energy.
Why AI Training Models Are Energy Intensive
Training AI models is an energy-hungry process because:
- Complexity of models: Larger models with more parameters need longer training times on high-performance hardware.
- Iterations and datasets: Training involves running through datasets multiple times to fine-tune accuracy.
- Hardware requirements: GPUs and TPUs, commonly used for training, are power-intensive devices.
As AI continues to advance, understanding its environmental impact is key to ensuring a sustainable future.
The Environmental Cost of Training AI Models
Energy Consumption in AI Training
Training modern AI models is no small feat—it’s an energy-intensive process. These models rely on vast computational resources, often using thousands of GPUs or TPUs running for weeks or even months. The sheer amount of electricity required to train a single large model can rival the yearly consumption of a small town. This energy demand translates directly into significant carbon emissions, especially when powered by non-renewable energy sources.
For example, training a state-of-the-art language model like GPT-3 was estimated to consume enough energy to emit over 626,000 pounds of carbon dioxide equivalent. To put it in perspective, that’s roughly five times the lifetime emissions of an average American car, including its manufacturing.
Carbon Emissions from Large Language Models
Large language models are particularly notorious for their environmental impact. The emissions grow proportionally with model size and complexity. Adding more parameters or fine-tuning for higher accuracy can exponentially increase energy use. A process called neural architecture search, which tweaks models for optimal performance, often results in marginal gains but comes at a steep environmental cost.
Model Type | Estimated Carbon Emissions (lbs CO2e) | Equivalent Cars (Lifetime Emissions) |
---|---|---|
GPT-3 (Training) | 626,000 | 5 |
Smaller AI Models | 50,000 | 0.4 |
Comparing AI Training to Everyday Carbon Sources
To truly grasp the environmental toll, it helps to compare AI training to everyday activities:
- Training a large AI model can emit as much carbon as flying a passenger from New York to London and back 300 times.
- It’s comparable to the yearly electricity usage of 100 average U.S. homes.
- The emissions from a single training cycle could offset the savings of switching 10,000 homes to LED lighting for a year.
As AI continues to push boundaries, the environmental cost of training these models can no longer be ignored. Researchers and developers must weigh the benefits of advanced AI against its growing carbon footprint.
Strategies to Reduce AI's Carbon Footprint
Adopting Renewable Energy in Data Centers
Switching to renewable energy sources for powering data centers is a game-changer. Many cloud providers are already moving toward 100% renewable energy. For instance, some companies plan to achieve carbon-free operations by 2030, while others are on track for 2025. This shift drastically reduces the emissions associated with training and running AI models.
Key actions include:
- Investing in solar, wind, or hydroelectric power for data centers.
- Partnering with renewable energy providers for long-term contracts.
- Exploring innovative solutions like underwater data centers for efficient cooling.
Optimizing Algorithms for Efficiency
Efficient algorithms are like fuel-efficient cars—they do the same work but with less energy. By designing smarter algorithms, developers can cut down on the computational power required, which directly lowers energy consumption.
Strategies include:
- Developing lightweight models that need fewer parameters.
- Using techniques like pruning and quantization to simplify models.
- Employing transfer learning to reduce training time and resources.
The Role of Carbon Offsetting in AI
Even with all the optimizations, some emissions are unavoidable. That’s where carbon offsetting steps in. Companies can invest in projects like reforestation or renewable energy initiatives to balance out their emissions.
Here’s a simple breakdown:
- Calculate the carbon footprint of AI operations.
- Invest in certified carbon offset projects.
- Regularly review and adjust offset strategies to match emissions.
Reducing AI’s carbon footprint isn’t just about cutting emissions—it’s about creating a sustainable future where technology and the environment coexist responsibly.
For example, AI-powered energy management systems are already helping optimize energy use in industries, showcasing how AI can be part of the solution rather than the problem.
The Role of Model Size and Complexity
Impact of Model Parameters on Energy Use
The size of an AI model, particularly the number of parameters it contains, directly influences its energy consumption. Larger models require more computational power, which means longer training times and higher electricity demands. For example, training a model with billions of parameters can emit carbon dioxide equivalent to the lifetime emissions of several cars. This exponential energy demand highlights the environmental cost of prioritizing model size over efficiency.
Model Size (Parameters) | Estimated Carbon Emissions (lbs CO2) |
---|---|
Small (<100M) | ~1,000 |
Medium (~1B) | ~50,000 |
Large (>10B) | ~600,000 |
Trade-offs Between Accuracy and Environmental Cost
Bigger isn’t always better. While larger models often deliver higher accuracy, the environmental cost of achieving marginal improvements can be staggering. Researchers are exploring ways to balance performance with sustainability, such as:
- Pruning: Removing redundant parameters without affecting accuracy.
- Knowledge Distillation: Transferring knowledge from a large model to a smaller, efficient one.
- Task-Specific Models: Using smaller models tailored for specific tasks instead of general-purpose giants.
By adopting these strategies, developers can reduce the energy footprint without sacrificing too much accuracy.
Challenges in Scaling Down AI Models
Shrinking models isn’t as simple as cutting parameters. Developers face several hurdles, including:
- Loss of Generalization: Smaller models may struggle with diverse tasks.
- Algorithmic Complexity: Finding efficient algorithms requires significant effort and expertise.
- Hardware Limitations: Current hardware may not be optimized for smaller, specialized models.
Striking the right balance between model size and efficiency is not just a technical challenge—it’s an ethical one. As AI continues to scale, developers must consider not only what their models can achieve but also the environmental price of those achievements.
For instance, focusing on training large language models for every use case might not always justify the energy consumed. Instead, prioritizing smaller, task-specific models can significantly reduce emissions while meeting practical needs.
The Future of Sustainable AI Development
Emerging Trends in Green AI
AI is evolving, and so are the ways we can make it more sustainable. One promising trend is the use of pre-trained models, which cut down on the energy needed to train AI from scratch. Another is the rise of quantum computing and spiking neural networks—technologies designed to perform computations more efficiently. Federated learning, which processes data locally on devices instead of centralized servers, is also gaining traction. These innovations aim to reduce the carbon footprint of AI while still delivering powerful results.
Policy Recommendations for AI Sustainability
Governments and organizations need to step up with clear policies. A few ideas include:
- Mandating transparency in energy usage and carbon emissions for AI projects.
- Offering tax incentives or subsidies for AI companies that use renewable energy.
- Setting industry-wide benchmarks for energy efficiency in AI development.
These steps can encourage companies to prioritize sustainability without stifling innovation.
The Role of Researchers in Reducing Carbon Impact
Researchers play a big role in shaping the future of AI. By focusing on optimizing algorithms for energy efficiency, they can help lower the environmental costs of AI. Collaboration is key—sharing tools, data, and methods can lead to breakthroughs faster. Researchers should also advocate for clean energy sources for AI operations, making sure the energy powering their work comes from sustainable options.
"The future of AI doesn't just depend on what it can do, but how responsibly it can do it."
The Hidden Costs of AI Inference
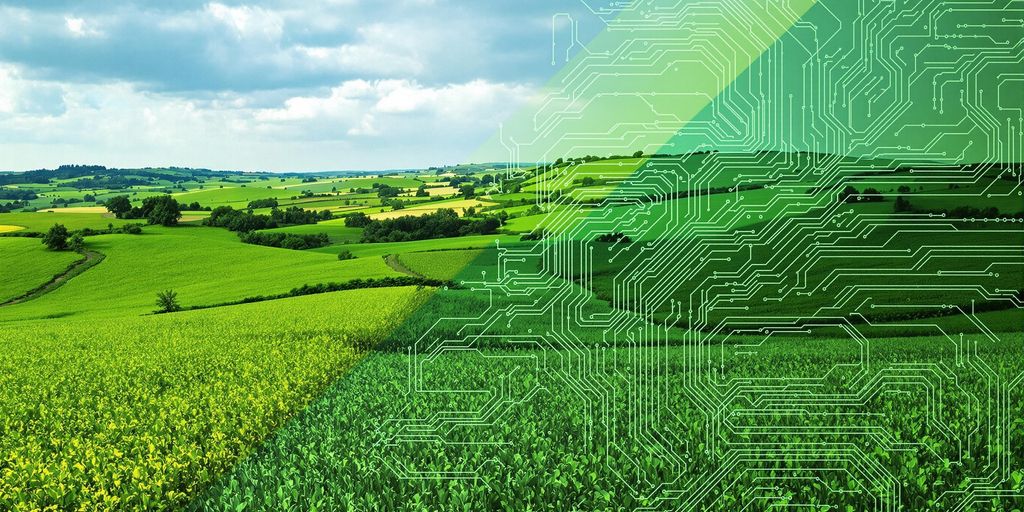
Energy Demands of AI Inference
AI inference, the phase where a trained model generates predictions or responses, is deceptively resource-intensive. Unlike training, which happens in controlled bursts, inference operates continuously, serving millions of queries daily. Google estimates that inference alone accounts for about 60% of AI's total energy consumption. This is because every time a user interacts with an AI-powered system, computational resources are engaged—sometimes for just seconds, but the sheer scale of usage adds up quickly.
For example, consider a popular language model serving 100 million users monthly. Each interaction, while seemingly minor, contributes to significant cumulative energy demands. This makes inference a hidden yet substantial contributor to AI's environmental impact.
Comparing Training and Inference Emissions
While training large models often grabs headlines for its carbon footprint, inference can outpace it in the long term. Training is a one-time or periodic effort, but inference is ongoing. A study estimated that a widely-used AI model's daily carbon footprint could reach 50 pounds of CO2, translating to over 8.4 tons annually. When scaled across multiple models and millions of users, the numbers become staggering.
Process | Carbon Footprint Example | Frequency |
---|---|---|
Model Training | 500-600 metric tons CO2 | Periodic |
Model Inference | 8.4 metric tons CO2/year | Continuous |
This comparison highlights why inference, though less publicized, deserves equal scrutiny when assessing AI's environmental cost.
Scaling AI for Millions of Users
The scalability of AI systems introduces another layer of complexity. As models are deployed to serve larger audiences, their energy consumption grows exponentially. For instance, models optimized for smaller datasets may struggle to maintain efficiency when scaled to global usage. This often leads to deploying additional infrastructure, such as high-powered servers, to meet demand.
- Increased user adoption drives higher energy requirements.
- Infrastructure upgrades, including specialized hardware, add to emissions.
- Serving real-time responses for global audiences compounds the problem.
The convenience of AI comes at an environmental price. As usage scales, so do the hidden costs, making it vital to address these challenges head-on.
Addressing the hidden costs of AI is not just about tweaking technology but rethinking how we design and deploy these systems. The road to sustainable AI must include solutions for inference, not just training.
Ethical Considerations in AI Carbon Footprint
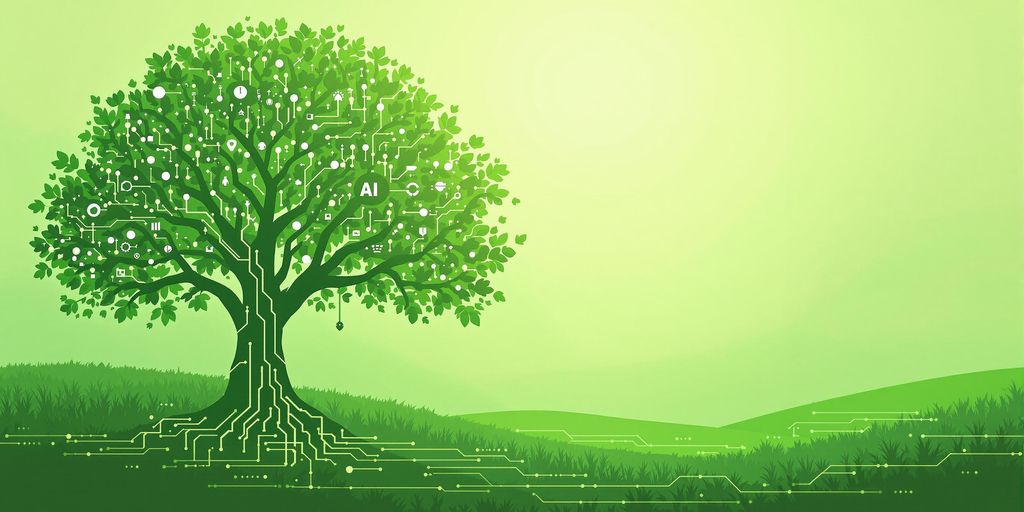
Incorporating Carbon Metrics in Research
When discussing the environmental impact of AI, it’s essential to include carbon metrics as a core part of research practices. Without measurable data, it’s impossible to know the true footprint of AI systems. Researchers should aim to publish the energy and carbon costs of their models alongside performance metrics. This level of transparency can drive accountability and push for greener innovations.
Balancing Innovation with Environmental Responsibility
AI advancements often come with a trade-off: innovation versus environmental cost. Balancing these two requires thoughtful decision-making. Here’s how:
- Prioritize energy-efficient models during development.
- Use renewable energy sources for training and deployment.
- Regularly assess whether the environmental cost of a project justifies its benefits.
AI can transform industries, but it must do so without leaving an unsustainable environmental footprint. Striking this balance is not just a technical challenge but a moral one.
The Need for Transparent Reporting in AI
Currently, many AI projects lack clear reporting on their energy use and emissions. This needs to change. Transparent reporting could include:
- Energy consumption during training and inference.
- The type of energy sources used (e.g., renewable vs. non-renewable).
- Comparisons with industry benchmarks.
By making these details public, companies and researchers can encourage competition for more sustainable practices. For example, renewable energy sources in data centers could significantly lower AI’s carbon impact, setting a new standard for the industry.
Conclusion
The environmental impact of training AI models is a growing concern that can't be ignored. While the advancements in AI are impressive, they come at a cost—both financially and environmentally. The energy demands of these systems are staggering, and the resulting carbon emissions are significant. However, there are ways to address this. Using renewable energy sources, designing more efficient algorithms, and being mindful of the resources we use can make a difference. It's clear that as we continue to push the boundaries of AI, we also need to take responsibility for its footprint. Balancing innovation with sustainability is not just an option; it's a necessity for the future.
Frequently Asked Questions
What is the carbon footprint of training AI models?
The carbon footprint of training AI models refers to the amount of greenhouse gases, like carbon dioxide, released into the atmosphere due to the energy used in the training process. This energy powers the computers and data centers that handle the training tasks.
Why do AI models require so much energy to train?
AI models need a lot of energy because they use powerful computers to process massive amounts of data. The larger and more complex the model, the more energy it consumes to achieve accurate results.
How can renewable energy help reduce AI’s carbon footprint?
Using renewable energy sources, like wind or solar power, to run data centers can significantly cut down the carbon emissions generated during AI training and inference processes.
Are there ways to make AI training more energy-efficient?
Yes, optimizing algorithms and using more efficient hardware can reduce the energy needed for training AI models. Researchers are also exploring ways to achieve high performance with smaller, less resource-intensive models.
What is the difference between AI training and inference in terms of energy use?
Training involves teaching the AI model using large datasets, which is energy-intensive. Inference, on the other hand, is when the trained model makes predictions or answers queries. While inference is less energy-intensive per task, it can still consume a lot of energy when scaled to millions of users.
Why is it important to track the carbon footprint of AI?
Tracking the carbon footprint helps researchers and companies understand the environmental impact of their AI projects. This awareness can drive efforts to adopt sustainable practices and reduce emissions.