Cloud AI vs On-Premise Solutions—Which One Wins?
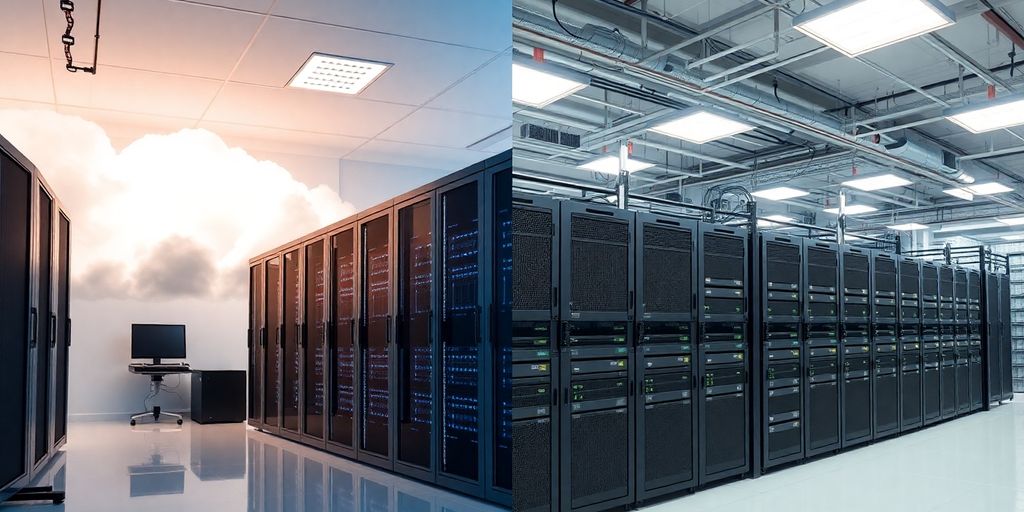
Choosing between Cloud AI and On-Premise AI can feel like picking between two different worlds. Both have their strengths and weaknesses, and what works for one business might not suit another. From cost to security, scalability to long-term value, there’s a lot to think about. This article breaks it down to help you find the best fit for your needs.
Key Takeaways
- Cloud AI offers flexibility with a pay-as-you-go model, but costs can add up quickly for high usage.
- On-Premise AI provides full control over data and infrastructure, making it ideal for sensitive or regulated industries.
- Scalability is easier with Cloud AI, while On-Premise AI may require significant upfront investment for expansion.
- Cloud AI can pose compliance challenges, whereas On-Premise AI keeps data closer to home and under tighter control.
- Hybrid models combine the best of both worlds, offering a balanced approach to flexibility and security.
Understanding the Core Differences Between Cloud AI and On-Premise Solutions
Defining Cloud AI and On-Premise AI
Cloud AI operates through remote servers managed by external providers, offering businesses the ability to scale and adapt quickly. On the other hand, on-premise AI is hosted entirely on an organization’s own infrastructure, giving them complete control over their data and systems. This difference often boils down to flexibility versus ownership—cloud AI excels in accessibility, while on-premise AI ensures tighter control.
Key Architectural Variations
The architecture of these two models is fundamentally different. Cloud AI relies on shared resources, meaning multiple users access the same servers, often leading to cost savings but less customization. In contrast, on-premise AI uses dedicated infrastructure, which:
- Requires upfront investment in hardware and maintenance.
- Offers the ability to tailor resources to specific workloads.
- Avoids dependency on third-party providers.
For companies needing unique configurations or working in regulated industries, this architectural control can be a game-changer.
How Data Management Differs
Data management is another critical area of divergence. With cloud AI, data is stored and processed offsite, raising potential concerns about privacy and compliance. On-premise AI keeps data within the organization’s own servers, ensuring it never leaves their control. Here’s a quick comparison:
Feature | Cloud AI | On-Premise AI |
---|---|---|
Data Location | Stored in external servers | Stored on internal servers |
Compliance | Dependent on provider policies | Fully controlled internally |
Scalability | Highly scalable | Limited by local resources |
Choosing the right approach often depends on how sensitive your data is and how much control you need over it.
Each model has its strengths, but understanding these differences is the first step in selecting the one that aligns with your business goals.
Cost Implications: Cloud AI vs On-Premise
Predictable Costs with On-Premise AI
When you go with on-premise AI, you're essentially committing to a fixed cost structure. You buy the hardware upfront—servers, GPUs, and all that jazz—and it's yours to keep. Sure, there are ongoing costs like electricity, maintenance, and the occasional upgrade, but these are pretty stable. This predictability makes budgeting a whole lot easier compared to the rollercoaster of cloud expenses.
Here's a quick breakdown of typical on-premise costs:
Cost Type | Description |
---|---|
Capital Expenses | Initial hardware purchase and setup |
Operational Costs | Power, cooling, and maintenance |
Staff and Training | IT personnel and end-user training |
Software Licenses | Necessary tools and applications |
While it might seem like a big chunk of change upfront, the long-term savings can be significant, especially for businesses with heavy AI workloads.
Cloud AI’s Pay-As-You-Go Model
Cloud AI flips the script. Instead of buying hardware, you pay for what you use—whether it’s compute power, storage, or specific AI services. This means no upfront investment, but the monthly bills can vary wildly depending on usage. For some businesses, this flexibility is a godsend. For others, it’s a budgeting nightmare.
Let’s look at what you’re paying for in the cloud:
- Compute and storage resources (e.g., virtual machines)
- Data transfer costs
- Management and support fees
The big advantage? You can scale up or down as needed without worrying about physical hardware. But keep an eye on those hidden expenses—like data migration or exceeding usage limits. It adds up.
Hidden Costs to Watch For
Neither option is 100% straightforward. On-premise solutions come with surprise costs like unexpected repairs or the need for specialized staff. Meanwhile, cloud AI has its own sneaky pitfalls:
- Data Egress Fees: Moving data out of the cloud can cost a fortune.
- Over-Provisioning: Paying for more resources than you actually need.
- Vendor Lock-In: Switching providers can be complicated and pricey.
"Choosing between cloud and on-premise AI isn’t just about the sticker price. It’s about understanding the full cost picture—both the obvious and the hidden."
When deciding, consider your workload, scale, and how much control you want over your costs. For businesses looking for stable budgetary planning, on-premise might be the better bet. But if flexibility is key, cloud AI could be your go-to.
Data Security and Compliance in Cloud AI vs On-Premise
Regulatory Challenges in Cloud AI
Cloud-based AI solutions often come with shared responsibility models. While the cloud provider secures the infrastructure, the organization must manage its own data, access permissions, and configurations. This dual responsibility can lead to gaps in security if not handled meticulously. For example, failing to properly configure cloud storage can expose sensitive data to unauthorized access. Moreover, compliance with regulations like GDPR or HIPAA can be tricky in a cloud environment due to the distributed nature of data storage. Companies must ensure that their cloud vendors provide the necessary compliance enablers and audit trails.
On-Premise AI for Sensitive Data
On-premise AI solutions offer complete control over data security and compliance. With data stored and processed locally, organizations can directly implement their own security protocols and ensure compliance with industry-specific regulations. This is particularly beneficial for sectors like healthcare, finance, and government, where data privacy is non-negotiable. However, this approach requires significant investment in physical and network security measures, as well as ongoing maintenance and monitoring. While this can be resource-intensive, it eliminates the risks associated with third-party data custodianship.
Zero-Trust Security in Cloud AI
Zero-trust security models are becoming increasingly popular in cloud environments. These models operate on the principle of "never trust, always verify," ensuring that every access request is authenticated and authorized, regardless of its origin. This approach can significantly enhance security in cloud AI solutions, making them a viable option even for sensitive applications. However, implementing zero-trust security requires robust identity management systems and continuous monitoring, which can be complex and costly.
Choosing between cloud and on-premise AI solutions for data security and compliance often boils down to the specific needs of your organization. While cloud solutions offer scalability and modern security features like zero-trust, on-premise systems provide unparalleled control and are better suited for highly regulated industries.
Scalability and Flexibility: Which Model Excels?
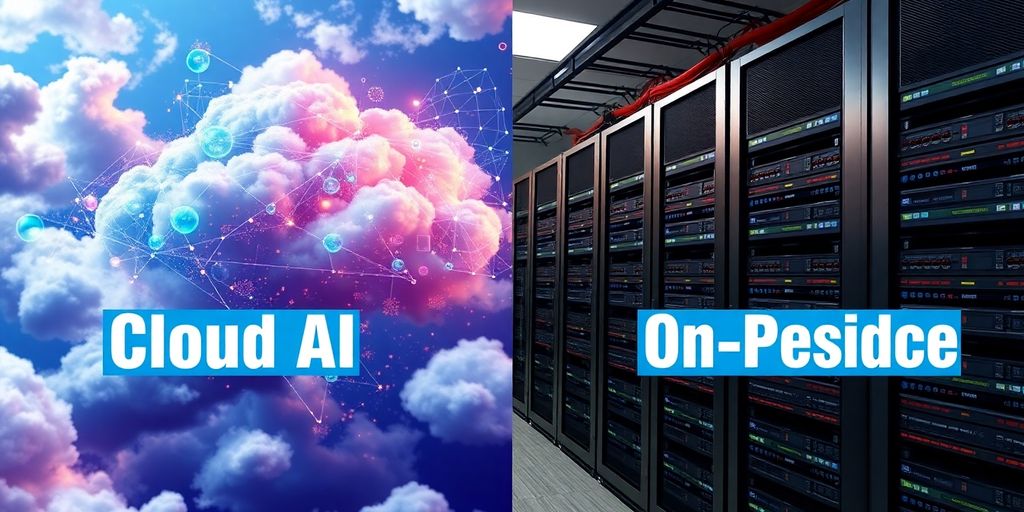
Scaling AI Workloads in the Cloud
When it comes to scaling, the cloud is the clear winner for businesses that need to adapt quickly. Cloud platforms allow you to increase or decrease resources on demand. No need for extra hardware or long-term commitments. For example:
- If your AI workload spikes during holiday seasons, you can allocate more resources instantly.
- Once the peak period ends, scale back to save costs.
- Automated tools can even predict and adjust resource needs in real-time.
This elasticity ensures you’re not overpaying for unused capacity or scrambling to add more when demand surges. Plus, cloud providers handle the infrastructure maintenance, so your team can focus on your AI models instead of hardware headaches.
On-Premise AI’s Modular Approach
On-premise solutions, on the other hand, give you full control—but scaling isn’t as straightforward. To grow, you’ll need to invest in new hardware, which can be both costly and time-consuming. Here’s what scaling on-premise might involve:
- Purchasing additional servers or upgrading existing ones.
- Configuring the new hardware to integrate with your current setup.
- Ensuring your IT team is ready to manage the expanded infrastructure.
While this approach gives you more control, it’s less flexible. If your growth slows down, you’re stuck with hardware you might not fully utilize.
Hybrid Models as a Middle Ground
For organizations that need both flexibility and control, hybrid models offer a balanced solution. These setups combine cloud and on-premise systems, allowing businesses to:
- Use the cloud for unpredictable workloads or testing new AI models.
- Keep sensitive or critical data on-premise for added security.
- Scale incrementally without overcommitting to one approach.
A hybrid model lets you take advantage of cloud elasticity while maintaining the control of on-premise systems. It’s like having the best of both worlds without fully committing to either one.
In the end, the right choice depends on your specific needs. If your business thrives on rapid changes, the cloud might be your best bet. But if control and predictability are your priorities, on-premise or hybrid solutions could be more fitting.
Operational Control and Customization
Vendor Lock-In Risks with Cloud AI
When you go with a cloud AI provider, you're often locking yourself into their ecosystem. Sure, they offer plenty of tools and services, but they also hold the keys to your data and infrastructure. If you decide to switch providers later, it can be a logistical headache. Data migration costs, compatibility issues, and potential downtime are all risks to consider. This is particularly true if the provider uses proprietary systems that don't play well with others.
Full Stack Control in On-Premise AI
On-premise AI solutions give you total control over your infrastructure. You own the hardware, the software, and the data. This means you can tweak and optimize every layer of your AI stack to fit your business needs. However, this level of control comes with a price—literally. Initial setup costs can be steep, and you'll need a skilled IT team to manage and maintain the system. For businesses that value autonomy and have the resources, though, this is often the preferred route.
Tailoring AI Solutions to Business Needs
Customization is another area where on-premise solutions shine. You can build and modify your AI systems to meet specific business requirements. Cloud AI, while flexible, often relies on standardized modules that may not offer the granularity you need. That said, cloud providers are improving in this area, offering more "a la carte" options for customization. But even then, the level of control doesn't quite match what you get with an on-premise setup.
If your business has unique needs or operates in a highly regulated industry, on-premise AI might be worth the investment. The ability to customize and control every aspect of the system can provide a significant competitive advantage.
Quick Comparison Table
Feature | Cloud AI | On-Premise AI |
---|---|---|
Initial Costs | Low | High |
Long-Term Costs | Pay-as-you-go | Maintenance and upgrades |
Customization | Limited | Extensive |
Data Ownership | Shared with provider | Full control |
Scalability | Easy and fast | Requires hardware upgrades |
For businesses weighing their options, it's all about balancing flexibility, control, and cost. While Cloud Learning Management Systems (LMS) make a compelling case for lower initial expenses, on-premise solutions often win in terms of customization and long-term control.
Evaluating Long-Term Business Value
Measuring ROI in Cloud AI
Determining the return on investment (ROI) for cloud AI solutions can be straightforward if you know what to look for. The "pay-as-you-go" model is a standout feature, allowing businesses to scale costs directly with usage. This flexibility is especially useful for companies with fluctuating workloads. To measure ROI effectively, consider these steps:
- Track operational efficiency improvements, such as reduced manual processes.
- Compare pre- and post-implementation metrics, like task completion times or customer response rates.
- Monitor cost savings from reduced hardware and maintenance expenses.
For example, a company using cloud AI for customer service might see a 15% reduction in response times, leading to higher customer satisfaction scores.
Cost Efficiency of On-Premise AI
On-premise AI shines when it comes to predictable, long-term costs. Once the initial setup is complete, ongoing expenses like hardware upgrades and staffing are easier to forecast. However, there’s a trade-off: upfront investment is higher. Here’s a quick comparison:
Feature | On-Premise AI | Cloud AI |
---|---|---|
Upfront Costs | High | Low |
Maintenance Costs | Medium | Low to Medium |
Scalability Costs | High | Variable |
Businesses that prioritize control over their infrastructure often find on-premise AI aligns better with their financial planning.
Avoiding Technical Debt
Technical debt is a hidden cost that can sneak up on businesses, especially those locked into proprietary cloud platforms. This "debt" arises when short-term solutions limit future flexibility. To avoid this:
- Choose open-source or vendor-agnostic tools whenever possible.
- Regularly evaluate your AI stack for compatibility with emerging technologies.
- Plan for scalability and future integrations from day one.
Thinking long-term means balancing immediate gains with the ability to adapt to future needs. Decisions made today can either empower or restrict your business tomorrow.
By carefully evaluating these factors, businesses can ensure their AI investments provide lasting value, whether in the cloud or on-premise.
Industry-Specific Considerations
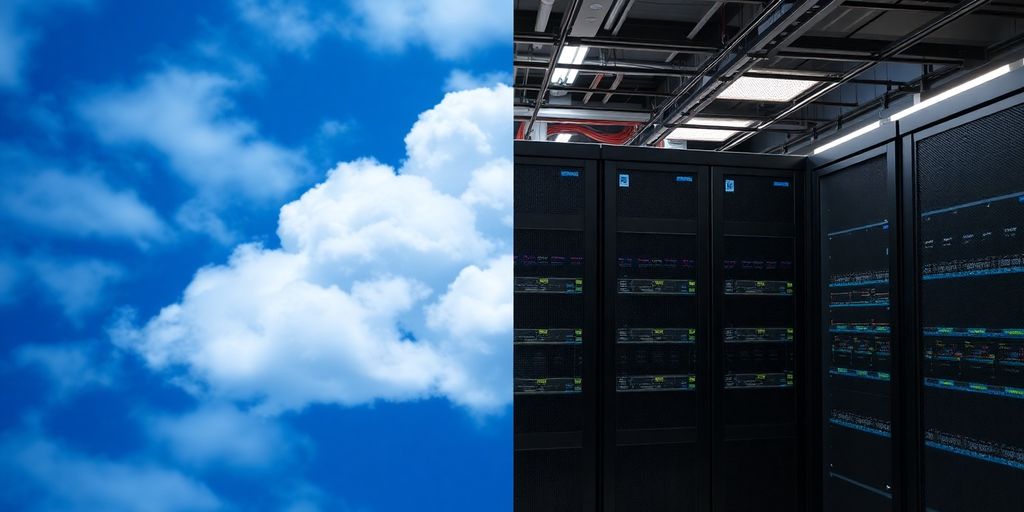
Healthcare and Data Privacy
Healthcare organizations face unique challenges when it comes to adopting AI solutions. Patient data is one of the most sensitive types of information, and ensuring compliance with regulations like HIPAA in the U.S. is non-negotiable. On-premise AI solutions often provide the control needed to meet these strict requirements. However, cloud AI can still be a viable option if paired with robust encryption and data segmentation strategies. For healthcare providers, the choice often hinges on balancing the need for security with the scalability offered by cloud solutions.
Government and Regulatory Compliance
Governments operate under tight regulatory frameworks that vary widely across regions. Many public sector entities opt for on-premise AI to maintain control over sensitive data and to comply with local laws. For example, certain jurisdictions require that data never leave the country, making cloud solutions less feasible unless they offer localized data centers. On the other hand, cloud AI can be advantageous for non-sensitive applications, such as traffic management or public service chatbots, where flexibility and cost-efficiency are key.
Finance and Operational Security
The financial sector is another industry where operational security is paramount. AI applications like fraud detection and risk assessment often deal with real-time data that must be processed quickly and securely. On-premise solutions provide the speed and control necessary for these critical operations. However, cloud AI is gaining traction for its ability to scale and integrate with other financial technologies. Hybrid models are increasingly popular, allowing financial institutions to keep sensitive operations in-house while leveraging the cloud for less critical tasks.
Conclusion
When it comes to choosing between cloud AI and on-premise solutions, there’s no one-size-fits-all answer. Each option has its perks and challenges, and the right choice really depends on what your business needs. If you’re looking for flexibility and scalability, the cloud might be your best bet. But if control, data privacy, and predictable costs are your priorities, on-premise solutions could be the way to go. At the end of the day, it’s about finding the balance that works for your goals and resources. Whether you go all-in on one approach or mix the two, the key is to make a decision that aligns with your long-term strategy.
Frequently Asked Questions
What is the main difference between Cloud AI and On-Premise AI?
Cloud AI relies on remote servers to process and store data, while On-Premise AI operates locally within a company's own infrastructure. This means Cloud AI is more flexible and scalable, but On-Premise AI offers greater control and data privacy.
Which is more cost-effective: Cloud AI or On-Premise AI?
It depends on your needs. Cloud AI uses a pay-as-you-go model, which can be cost-efficient for smaller or fluctuating workloads. On the other hand, On-Premise AI has higher upfront costs but can save money in the long run for consistent, heavy workloads.
Is Cloud AI secure enough for sensitive data?
Cloud AI can be secure if proper measures like encryption and zero-trust security are in place. However, industries with strict compliance requirements, like healthcare or government, often prefer On-Premise AI for full control over data security.
Can On-Premise AI scale as easily as Cloud AI?
On-Premise AI can scale, but it requires additional hardware and infrastructure, which takes time and money. Cloud AI, however, can scale almost instantly by leveraging virtual resources.
What are the hidden costs of Cloud AI?
Hidden costs in Cloud AI can include data transfer fees, storage costs, and vendor lock-in expenses. These can add up quickly if not carefully managed.
Which is better for customization: Cloud AI or On-Premise AI?
On-Premise AI offers more customization options because you have full control over the hardware, software, and configurations. Cloud AI, while flexible, often comes with limitations tied to the provider's ecosystem.