Bias in AI—How Algorithms Are Influencing Society
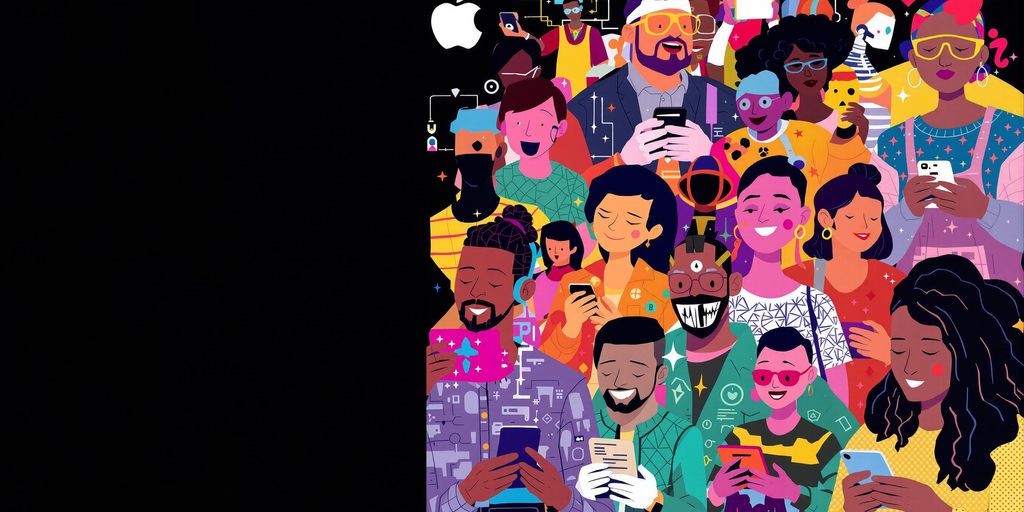
AI is everywhere these days—whether it's deciding what ad you see online or helping companies sort through job applications. But here's the kicker: these systems aren't perfect. Sometimes, they pick up on biases from the data they're trained on, and that can lead to some pretty unfair outcomes. This isn't just a tech problem; it's a people problem, too. When AI mirrors and amplifies human prejudices, it can seriously mess with our lives, especially for those who are already marginalized. So, let's break down how this happens and what we can do about it.
Key Takeaways
- AI bias happens when algorithms reflect or amplify human prejudices.
- Training data plays a huge role in how biases form in AI systems.
- Real-world examples show bias in hiring, healthcare, and policing.
- Marginalized groups often face the worst consequences of biased AI.
- Fixing AI bias requires better data, ethical guidelines, and constant checks.
Understanding AI Bias and Its Societal Impact
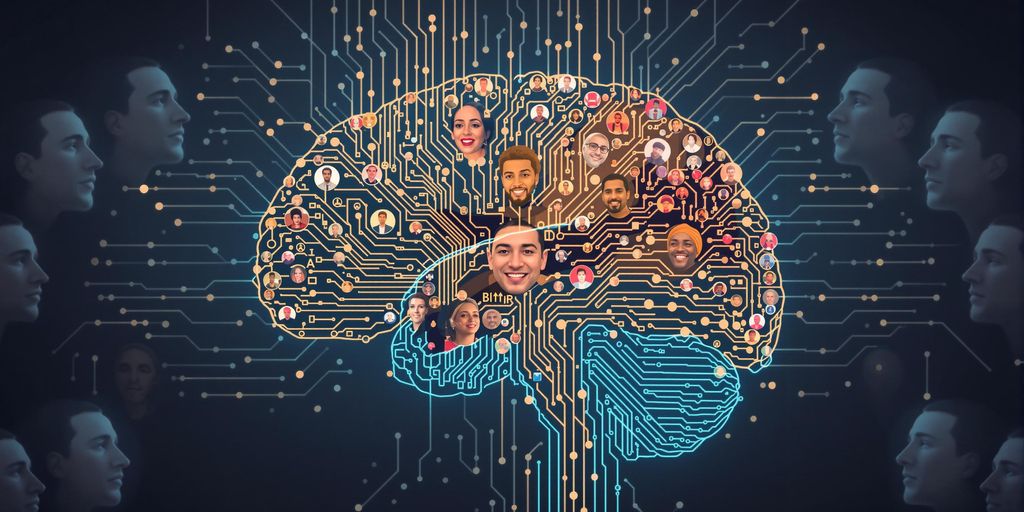
Defining AI Bias and Its Origins
AI bias happens when artificial intelligence systems produce unfair results because of the data or algorithms they rely on. This bias often mirrors the inequalities and prejudices already present in society. It can show up in the training data, the way algorithms are designed, or even in the predictions AI makes. For example, if a dataset mostly includes data from one demographic, the AI might struggle to make accurate decisions for people outside that group. This isn’t just a tech issue—it’s a human one.
How AI Bias Reflects Human Prejudices
AI doesn’t operate in a vacuum. It learns from historical data, and that data often carries the biases of the people or systems that created it. Whether it’s favoring one gender over another or overlooking minority groups, AI systems can amplify these problems. Think about it: if humans have been biased in hiring, lending, or policing, and AI learns from those patterns, the bias doesn’t just stay—it scales up. It’s like putting a magnifying glass on society’s flaws.
The Role of Training Data in Bias Formation
Training data is like the foundation of a house—if it’s flawed, everything built on it will be too. AI systems depend on data to learn, but if that data is incomplete or skewed, the results will be as well. Here’s what typically goes wrong:
- Underrepresentation: Certain groups might not be well-represented in the data.
- Historical Inequalities: Past injustices can show up in the data and carry forward.
- Data Collection Methods: How data is gathered can introduce its own set of biases.
When AI reflects biased patterns, it’s not just a technical glitch; it’s a mirror of societal inequities. Fixing it requires more than just better algorithms—it demands a hard look at the data and the systems behind it.
Real-World Examples of AI Bias
Bias in Healthcare Algorithms
Healthcare is one of the most critical areas where AI bias has surfaced. Predictive algorithms often fail to provide accurate results for underrepresented groups. For instance, some diagnostic tools have shown lower accuracy rates for Black patients compared to White patients. This happens because the data used to train these systems often lacks diversity, skewing the results. When healthcare tools are biased, it can lead to misdiagnoses and unequal treatment, putting lives at risk.
Gender Disparities in Recruitment Tools
AI-driven recruitment tools have also shown bias, particularly against women. A notable example is a hiring algorithm that favored male candidates because it was trained on resumes predominantly submitted by men. The algorithm picked up on gendered language, like words such as "executed" or "captured," which appeared more frequently in male resumes. This kind of bias perpetuates existing inequalities in the workplace and makes it harder for women to compete on a level playing field.
Racial Profiling in Predictive Policing
Predictive policing systems, designed to allocate law enforcement resources, have been criticized for racial bias. These algorithms often direct more police activity toward minority neighborhoods, not because of higher crime rates, but because the historical data used to train them reflects systemic biases. This creates a feedback loop where over-policing in these areas leads to more arrests, reinforcing the original bias.
AI bias systematically discriminates against specific individuals or groups, highlighting a significant real-world crisis that requires urgent action.
The Root Causes of AI Bias
Historical Exclusion in Data Collection
The foundation of AI systems lies in the data they are trained on. When this data excludes certain groups, the resulting AI models inherit these gaps. For instance, datasets that predominantly include information from urban areas might fail to represent rural communities. This exclusion isn't always intentional, but its effects can be far-reaching.
- Early medical datasets often lacked sufficient data on women and minorities, leading to diagnostic tools that work better for men.
- Language models trained on English-centric data struggle with non-English dialects or languages, sidelining global populations.
- Historical underrepresentation of certain professions by gender or race skews predictions in recruitment tools.
Cognitive Bias in Algorithm Design
Humans build AI systems, and our biases inevitably seep into the design. Whether it's the choice of features, weighting factors, or even the decision on which data to prioritize, cognitive bias can shape AI outcomes in ways we don't always foresee.
- Developers might unconsciously favor datasets that align with their own experiences, such as using American-centric data for global applications.
- Labeling inconsistencies, like associating certain job titles with specific genders, can perpetuate stereotypes.
- Algorithms might overemphasize irrelevant factors, like income or vocabulary, unintentionally disadvantaging certain groups.
AI systems are only as unbiased as the people who create them, making human oversight a double-edged sword.
Oversimplification and Stereotyping
AI models are designed to find patterns, but this can lead to oversimplification. Complex human behaviors and identities get reduced to binary categories or averages, stripping away nuance.
- Facial recognition systems often struggle with diverse skin tones because they "average out" features during training.
- Predictive policing tools may stereotype certain neighborhoods as high-crime based on historical data, perpetuating systemic issues.
- Simplistic categorization in recommendation systems can pigeonhole users, limiting their exposure to diverse content.
Source of Bias | Example | Impact |
---|---|---|
Historical Exclusion | Lack of rural data in urban datasets | Misrepresentation of rural needs |
Cognitive Bias | Gendered job title associations | Reinforcement of stereotypes |
Oversimplification | Skin tone averaging in facial recognition | Reduced accuracy for darker skin tones |
Understanding these root causes is the first step in addressing AI bias. By identifying where things go wrong, we can start to build systems that are more equitable and inclusive for everyone.
Consequences of AI Bias on Marginalized Communities
Impact on People with Disabilities
AI systems often fail to account for the diverse needs of individuals with disabilities. For example, facial recognition software may misidentify individuals with facial asymmetry or those who use assistive devices. Speech recognition tools, too, can struggle to understand atypical speech patterns. These inaccuracies can lead to exclusion or even life-threatening consequences.
- Misidentification by AI security systems can label assistive devices as threats.
- Speech impairments may render voice-activated technologies inaccessible.
- People with cognitive disabilities might face challenges navigating AI-driven interfaces.
When technology overlooks disability-specific needs, it risks perpetuating systemic barriers rather than breaking them.
Reinforcement of Gender Stereotypes
AI algorithms often mirror societal biases, including those related to gender. Recruitment tools, for instance, have been found to favor male candidates by prioritizing language and experiences more commonly associated with men. Similarly, advertising algorithms might target job ads for high-paying roles predominantly to men, reinforcing outdated stereotypes.
- Hiring algorithms may undervalue resumes using "female-coded" language.
- Job ads for leadership roles may disproportionately reach men.
- AI-driven content moderation can unfairly censor discussions on gender issues.
Economic Inequities Amplified by AI
AI has the potential to widen economic disparities, especially for marginalized groups. Loan approval systems, for example, may deny credit to individuals from historically underserved communities due to biased training data. Predictive algorithms in hiring can also favor candidates from wealthier backgrounds, sidelining those with fewer resources.
Scenario | Potential Bias | Impact |
---|---|---|
Loan Approvals | Historical exclusion from financial data | Reduced access to credit opportunities |
Hiring Algorithms | Preference for elite educational backgrounds | Marginalization of lower-income applicants |
Gig Economy Platforms | Low prioritization of minority workers | Limited earning potential |
Without deliberate intervention, AI risks becoming a mechanism for deepening existing inequalities.
Strategies to Mitigate AI Bias
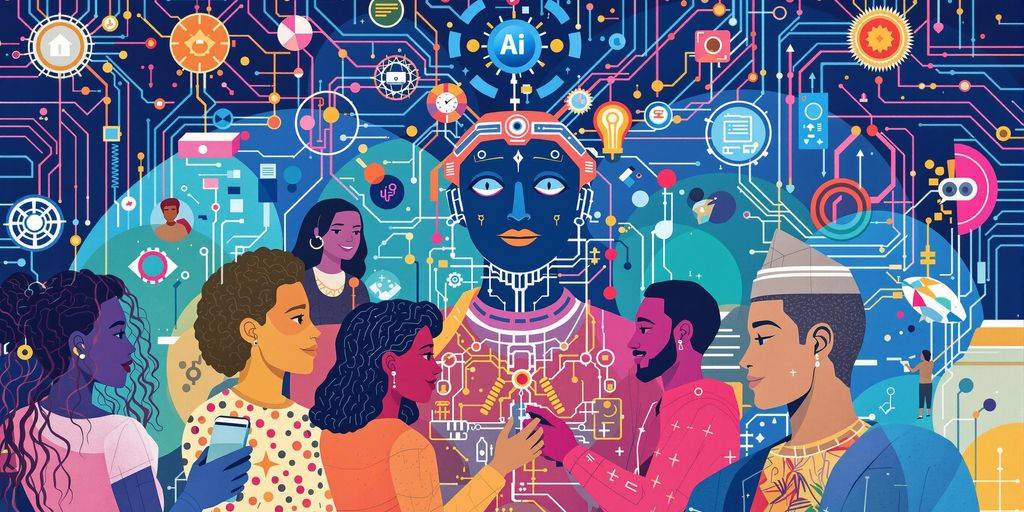
Ensuring Diverse and Inclusive Data
To tackle AI bias, the first step is addressing the data it learns from. Algorithms are only as good as the information they’re fed. If the training data is skewed, the results will be too. Here’s how to improve data diversity:
- Collect data from a wide range of demographics to ensure balanced representation.
- Regularly audit datasets to identify and correct over- or underrepresentation.
- Avoid historical data that perpetuates systemic biases.
Implementing Ethical AI Governance
Building ethical AI isn’t just about the tech—it’s also about the policies and practices surrounding it. Companies need clear frameworks to guide their AI development:
- Assemble cross-functional teams to oversee AI projects. This could include engineers, ethicists, and legal experts.
- Set up accountability measures, like regular bias audits.
- Make AI decisions transparent to the public whenever possible.
Continuous Monitoring and Evaluation
AI systems evolve over time, which means bias can creep in even after deployment. Ongoing vigilance is key:
- Use tools to monitor model performance and flag disparities.
- Update algorithms as societal norms and expectations shift.
- Involve external reviewers to provide unbiased assessments.
Addressing AI bias isn’t a one-and-done task. It’s a continuous process that requires commitment, collaboration, and constant reevaluation.
The Future of Ethical AI Development
Interdisciplinary Collaboration for Fair AI
Creating ethical AI isn’t something that can be done in a vacuum. It needs input from all kinds of people—engineers, sociologists, ethicists, and even policymakers. Teams that mix different perspectives are better at spotting blind spots in algorithms. For example, a diverse group can help identify biases that a single-discipline team might overlook. The more varied the voices at the table, the fairer the AI systems can become.
- Encourage partnerships between tech companies and academic researchers to test AI models.
- Include marginalized communities in discussions about AI applications.
- Establish regular cross-disciplinary reviews to catch issues early.
The Role of Policy in Reducing Bias
Governments and organizations need clear rules to guide AI development. Policies can help set boundaries, like ensuring algorithms are transparent and explainable. Some countries are already drafting laws to regulate AI, but there’s still a long way to go. Policies should also focus on accountability—who’s responsible when AI makes a bad decision?
Policy Focus | Examples |
---|---|
Transparency | Requiring companies to disclose how AI works. |
Accountability | Defining liability for AI-driven errors. |
Equity | Mandating unbiased training datasets. |
Building Trust Through Transparency
AI systems often feel like black boxes—you see the output, but you have no idea how it got there. This lack of clarity can make people distrustful. To fix this, AI developers should make their models as open as possible. Explainability tools, for instance, can show why an algorithm made a specific decision.
Trust isn’t just about making AI work well; it’s about showing people that it’s working fairly and ethically.
- Share detailed documentation about AI processes.
- Use plain language to explain complex systems.
- Regularly update stakeholders on improvements.
For a world where AI aligns with fairness and accountability, the future of AI ethics is more important than ever.
Conclusion
AI is shaping our world in ways we couldn’t have imagined just a few decades ago, but it’s clear that it’s not without its flaws. Bias in AI isn’t just a tech problem—it’s a reflection of the biases we carry as a society. Whether it’s in hiring, healthcare, or even the ads we see online, these systems can amplify inequalities if we’re not careful. The good news? Awareness is growing, and people are starting to ask the right questions. But fixing this won’t happen overnight. It’s going to take effort from developers, businesses, and policymakers to ensure AI works for everyone, not just a select few. The future of AI depends on us getting this right.
Frequently Asked Questions
What is AI bias?
AI bias happens when artificial intelligence systems produce results that unfairly favor or disadvantage certain groups. This can occur because of biased data, flawed algorithms, or the way systems are designed.
How does AI bias affect people?
AI bias can lead to unfair treatment in areas like hiring, healthcare, and policing. For example, biased systems might favor certain genders for jobs or misdiagnose people from specific racial groups.
Why does AI bias exist?
AI bias exists because the data used to train AI often reflects human prejudices or historical inequalities. Additionally, the way algorithms are designed can unintentionally amplify these biases.
Can AI bias be fixed?
Yes, AI bias can be reduced by using diverse and inclusive data, testing systems for fairness, and involving different perspectives in the development process.
What are some real-world examples of AI bias?
Examples include hiring tools that favor male applicants, healthcare algorithms that perform poorly for minority groups, and predictive policing systems that unfairly target certain communities.
What can be done to prevent AI bias?
To prevent AI bias, developers can ensure the data is balanced and representative, test systems regularly, and follow ethical guidelines for AI development.