Are AI-Developed Drugs the Cure of the Future?
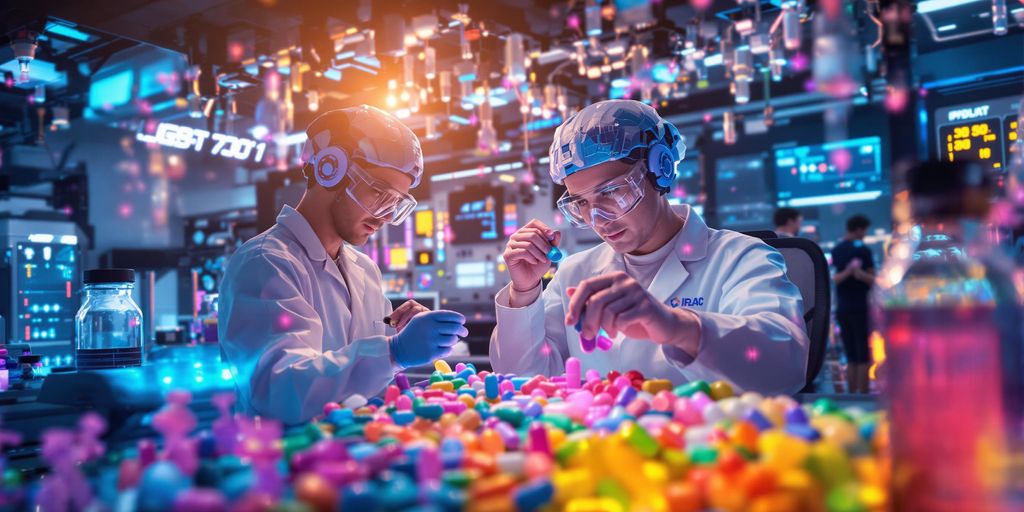
AI is shaking up the world of drug development. From speeding up research to cutting costs, it's becoming a game-changer in how we find and create new medicines. But it's not all smooth sailing. While the potential is huge, challenges like data quality and ethical concerns still loom large. So, what does this mean for the future of healthcare? Let's dive in.
Key Takeaways
- AI is revolutionizing drug discovery by making it faster and more cost-effective.
- The technology is being used for tasks like drug repurposing and optimizing clinical trials.
- Despite its promise, challenges like data reliability and ethical concerns remain.
- AI has the potential to address unmet medical needs, such as rare diseases and antimicrobial resistance.
- Collaborations between tech companies and pharma are driving innovation in AI-driven drug development.
The Role of AI in Revolutionizing Drug Discovery
How AI Identifies Drug Targets
Traditional drug discovery often feels like searching for a needle in a haystack. AI changes the game by analyzing massive biological datasets to pinpoint potential drug targets like proteins or genes linked to diseases. Machine learning tools can sift through genetic, proteomic, and clinical data to identify the key players in a disease process. This means researchers can focus on the most promising targets instead of wasting time on dead ends.
- Key benefits of AI in target identification:
- Faster analysis of complex datasets.
- Discovery of previously overlooked targets.
- Reduced reliance on trial-and-error methods.
Accelerating Compound Screening with AI
Screening chemical compounds for potential drugs is like testing millions of keys to find the one that fits a lock. AI speeds up this process by predicting which compounds are most likely to succeed. Algorithms can simulate how a compound interacts with a target, cutting down the need for physical testing. This not only saves time but also slashes costs.
Traditional Approach | AI-Powered Screening |
---|---|
Takes years to test thousands of compounds. | Screens millions of compounds in weeks. |
High failure rates in later stages. | Early identification of promising candidates. |
Costly lab experiments. | Reduced need for physical testing. |
AI-Driven Predictive Modeling for Drug Safety
Drug safety is a major hurdle in development. Nearly 90% of drug candidates fail due to safety or efficacy issues. AI helps predict potential side effects early by modeling how a drug interacts with the human body. Using historical data, these models can flag risks before clinical trials even begin.
AI isn’t just about speed—it’s about making smarter decisions early on. By predicting safety concerns ahead of time, it prevents wasted resources and improves the odds of success.
Applications of AI in Drug Development
AI in Drug Repurposing
AI has turned out to be a game-changer in finding new purposes for existing drugs. By analyzing huge datasets of clinical and molecular information, AI can uncover unexpected uses for medications that were originally developed for other conditions. For instance, a drug designed for heart disease might show promise in treating a rare neurological disorder. This approach not only saves time but also reduces the cost and risks associated with developing entirely new drugs. AI helps connect dots that humans might miss, bringing overlooked research findings into the spotlight.
Optimizing Clinical Trials with AI
Clinical trials are one of the most expensive and time-consuming steps in drug development. AI is helping streamline this process by selecting the right participants and predicting outcomes more accurately. By analyzing patient data, AI can identify those who are most likely to benefit from a treatment, improving the chances of success. It also helps design better trial protocols by simulating different scenarios, which can lead to faster approvals and fewer delays. This means fewer failed trials and more effective treatments reaching patients sooner.
AI for Multi-Omics Data Integration
Diseases are complex, and understanding them requires looking at multiple layers of biological data—like genomics, proteomics, and metabolomics. AI excels in combining these "omics" datasets to provide a clearer picture of how diseases develop and progress. This integration allows researchers to identify new drug targets and develop therapies that are more tailored to individual patients. Think of it as putting together a giant, intricate puzzle where AI helps find the pieces that fit perfectly together.
Challenges in Adopting AI for Drug Development
Data Quality and Model Reliability Issues
AI systems thrive on data, but not all data is created equal. In drug development, the datasets often come from different sources, and they can be incomplete, inconsistent, or biased. This lack of uniformity can seriously mess with how well AI models perform. If the input data is flawed, the predictions and insights generated by AI can be unreliable.
- Many datasets lack diversity, which limits the AI's ability to generalize findings.
- Errors in data collection can cascade into larger issues when training models.
- High-quality, curated datasets are expensive and time-consuming to create.
Regulatory and Ethical Considerations
The regulatory world hasn’t quite caught up with the AI boom in drug discovery. Agencies like the FDA are still figuring out how to evaluate AI-based tools. Ethical concerns about how patient data is used and shared also add to the complexity.
- Guidelines for AI validation are still evolving.
- Ensuring patient data privacy remains a big hurdle.
- Lack of clear standards makes it harder for companies to gain approval for AI-driven solutions.
Overcoming the Black Box Problem
One of the biggest hurdles is the "black box" nature of many AI systems. Scientists and regulators often don’t fully understand how these models arrive at their conclusions, which can make them hesitant to trust the results.
Without transparency, it’s tough to rely on AI for critical decisions like predicting drug safety or efficacy.
Efforts to improve explainability are underway, but they’re not yet widespread. This is a major sticking point for getting more people on board with using AI in such a high-stakes field.
The Economic Impact of AI in Drug Discovery
Reducing Costs in Drug Development
Traditional drug development is notoriously expensive. It takes over a decade and upwards of $2 billion to bring a single drug to market. AI, however, is flipping the script. By automating labor-intensive tasks like target identification and compound screening, AI slashes both time and costs. This means fewer failed experiments and less wasted money.
Here’s how AI is cutting costs:
- Automating data analysis reduces the need for extensive human intervention.
- AI-guided compound screening identifies promising candidates faster.
- Predictive models minimize expensive late-stage failures.
Shortening Timeframes for Drug Approval
Time is money, especially in drug development. AI accelerates processes like preclinical testing, clinical trial design, and regulatory submissions. For example, AI-driven simulations can predict how a drug interacts with the human body, enabling researchers to skip unnecessary steps.
A quick comparison:
Stage | Traditional Timeframe | AI-Assisted Timeframe |
---|---|---|
Target Identification | 2-3 years | 6-12 months |
Preclinical Testing | 3-6 years | 1-2 years |
Clinical Trials | 6-8 years | 3-5 years |
Market Growth of AI-Driven Pharmaceuticals
The integration of AI into drug discovery isn’t just a scientific breakthrough—it’s an economic boom. Reports suggest the market for AI in drug development could hit billions in the next few years. Companies are racing to invest in AI platforms, and startups are emerging as key players in this growing field.
AI is not just transforming science; it’s reshaping the business landscape of pharmaceuticals. The potential to reduce costs and speed up timelines is attracting attention from investors and industry leaders alike.
AI’s ability to integrate with nature-inspired drug discovery is another game-changer, combining cutting-edge technology with traditional methods to create innovative medicines faster and cheaper.
Future Prospects of AI in Drug Development
Emerging Technologies Enhancing AI Capabilities
AI is already a game-changer in drug development, but new technologies like quantum computing are set to take it further. Quantum computers could help AI analyze complex biological data faster and more accurately, opening doors to drug designs that were previously impossible. Imagine integrating AI with multi-omics data—like genomics and proteomics—to get a clearer picture of diseases and how to treat them. This combination could redefine how we approach drug discovery.
Potential for Fully AI-Designed Drugs
The idea of drugs being entirely designed by AI is no longer science fiction. Some AI-generated drugs are already in early clinical trials. While none have hit the market yet, their progress is promising. These drugs could drastically cut the time and cost associated with traditional drug development. The shift from "human-guided" to "AI-led" drug design might be the next big leap in medicine.
Building Trust in AI Models for Drug Discovery
For AI to truly transform the field, researchers and regulators need to trust its predictions. Transparency is a big hurdle—AI often works like a "black box," making decisions that are hard to explain. Efforts are underway to make these models more interpretable and reliable. Regulatory bodies, like the CDER, are also working on frameworks to ensure AI is used responsibly in drug development. Building this trust is essential for AI's widespread adoption.
AI’s Role in Addressing Unmet Medical Needs
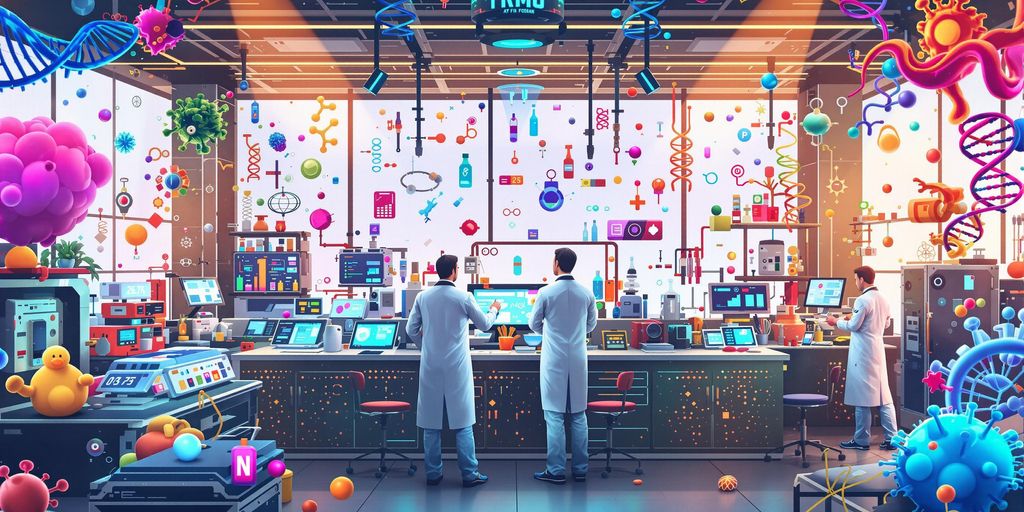
AI in Rare Disease Research
Rare diseases often face neglect due to their low prevalence and the high costs involved in researching treatments. AI is stepping in to change that. By analyzing genetic and clinical data, AI can uncover patterns that might otherwise go unnoticed. This helps in identifying potential targets for new therapies. AI-powered tools are transforming how we approach rare disease research, making it faster and more precise.
- AI algorithms can sift through vast datasets to identify genetic mutations linked to specific rare conditions.
- It can predict how these mutations affect biological pathways, guiding drug development.
- Researchers can prioritize the most promising drug candidates, reducing time and costs.
Tackling Antimicrobial Resistance with AI
Antimicrobial resistance (AMR) is a growing global crisis. Traditional methods of discovering new antibiotics are slow and often unfruitful. AI offers a new way forward:
- AI models analyze bacterial genomes to predict resistance mechanisms.
- They can design novel compounds that bacteria haven’t yet encountered, reducing the likelihood of resistance.
- AI also helps in repurposing existing drugs to counter resistant strains, saving both time and resources.
The ability of AI to predict and counteract bacterial resistance could be a game-changer in the fight against AMR.
AI-Driven Solutions for Cancer Therapies
Cancer treatment is highly complex, requiring personalized approaches. AI is making strides in this area by integrating multi-omics data—like genomics and proteomics—to develop tailored therapies. For instance:
- AI identifies biomarkers that predict how a patient will respond to a specific treatment.
- It can simulate drug interactions within the body, improving safety and efficacy.
- Researchers use AI to design combination therapies that attack cancer from multiple angles.
Organizations like Model Medicines are leveraging AI to model human biology and chemistry, expediting the development of transformative cancer therapies.
Collaborations Driving AI in Drug Development
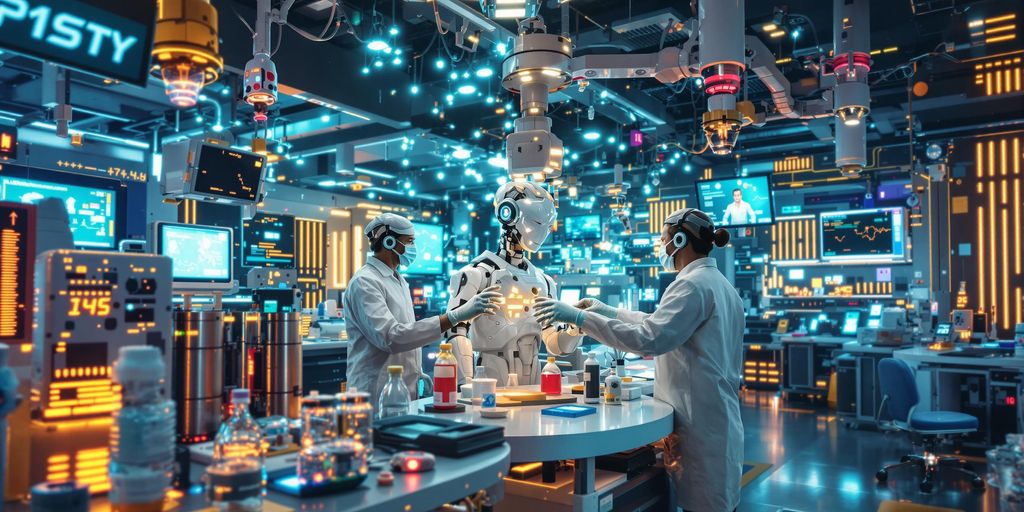
Partnerships Between Tech and Pharma
The pharmaceutical world and tech giants are increasingly joining forces to tackle the complex challenges of drug discovery. These partnerships aim to combine the computational power of AI with the deep biological expertise of pharmaceutical companies. For example, Servier and Google Cloud have extended their collaboration for five years, aiming to integrate AI and generative AI to speed up drug research Servier and Google Cloud partnership. This blend of expertise helps streamline processes like target identification and molecule design, which are traditionally slow and expensive.
Global Initiatives in AI Drug Research
Around the world, organizations are pooling resources and knowledge to drive innovation. Open-source platforms and international consortia are becoming popular for sharing AI models and datasets. This approach not only accelerates progress but also ensures that smaller players, like startups and academic labs, can contribute meaningfully. These global efforts aim to break down silos, allowing researchers to "connect the dots" between discoveries that might otherwise remain isolated.
The Role of Startups in AI-Driven Drug Discovery
Startups are playing a pivotal role in pushing the boundaries of AI in drug development. With their agility and focus, they often explore niche areas that larger organizations might overlook. Many startups specialize in using machine learning to predict protein structures, optimize drug formulations, or even generate entirely new compounds. By leveraging existing large-scale AI models and tailoring them for specific tasks, these companies can innovate without the massive costs typically associated with biotech R&D.
Collaboration is the backbone of AI-driven drug discovery. By working together, tech companies, pharmaceutical giants, and startups are reshaping what’s possible in medicine, making the process faster, cheaper, and more precise.
Conclusion
AI in drug development is still in its early days, but it’s already showing some serious promise. While no AI-designed drugs have hit the market yet, the technology is speeding up research and helping scientists make smarter decisions. It’s not perfect—there are still challenges like data quality and regulatory hurdles—but the potential is hard to ignore. As the tools improve and more people get on board, AI could change how we think about medicine entirely. Who knows? In a few decades, we might look back and wonder how we ever did it without AI.
Frequently Asked Questions
What is AI's role in drug discovery?
AI helps scientists find new drugs faster and cheaper by analyzing data, predicting how drugs will work, and identifying the best candidates for further testing.
Can AI help repurpose existing drugs?
Yes, AI can analyze data to find new uses for old drugs, potentially speeding up treatments for diseases by using medicines already proven safe.
How does AI make clinical trials better?
AI improves clinical trials by selecting the right patients, predicting outcomes, and designing better trial processes, making them more efficient.
What challenges does AI face in drug development?
AI struggles with issues like data quality, unclear regulations, and the difficulty of explaining its decisions, often called the 'black box' problem.
Are there AI-designed drugs in use today?
Some AI-designed drugs are in clinical trials, but none have been fully approved for public use yet. However, progress is being made.
How does AI impact the cost of drug development?
AI can reduce costs by identifying the most promising drug candidates early, cutting down on expensive lab work and failed trials.